Heterogeneous Multi-Functional Look-Up-Table-based Processing-in-Memory Architecture for Deep Learning Acceleration
2023 24th International Symposium on Quality Electronic Design (ISQED)(2023)
摘要
Emerging applications including deep neural networks (DNNs) and convolutional neural networks (CNNs) employ massive amounts of data to perform computations and data analysis. Such applications often lead to resource constraints and impose large overheads in data movement between memory and compute units. Several architectures such as Processing-in-Memory (PIM) are introduced to alleviate the bandwidth bottlenecks and inefficiency of traditional computing architectures. However, the existing PIM architectures represent a trade-off between power, performance, area, energy efficiency, and programmability. To better achieve the energy-efficiency and flexibility criteria simultaneously in hardware accelerators, we introduce a multi-functional look-up-table (LUT)-based reconfigurable PIM architecture in this work. The proposed architecture is a many-core architecture, each core comprises processing elements (PEs), a stand-alone processor with programmable functional units built using high-speed reconfigurable LUTs. The proposed LUTs can perform various operations, including convolutional, pooling, and activation that are required for CNN acceleration. Additionally, the proposed LUTs are capable of providing multiple outputs relating to different functionalities simultaneously without the need to design different LUTs for different functionalities. This leads to optimized area and power overheads. Furthermore, we also design special-function LUTs, which can provide simultaneous outputs for multiplication and accumulation as well as special activation functions such as hyperbolics and sigmoids. We have evaluated various CNNs such as LeNet, AlexNet, and ResNet18,34,50. Our experimental results have demonstrated that when AlexNet is implemented on the proposed architecture shows a maximum of 200× higher energy efficiency and 1.5× higher throughput than a DRAM-based LUT-based PIM architecture.
更多查看译文
AI 理解论文
溯源树
样例
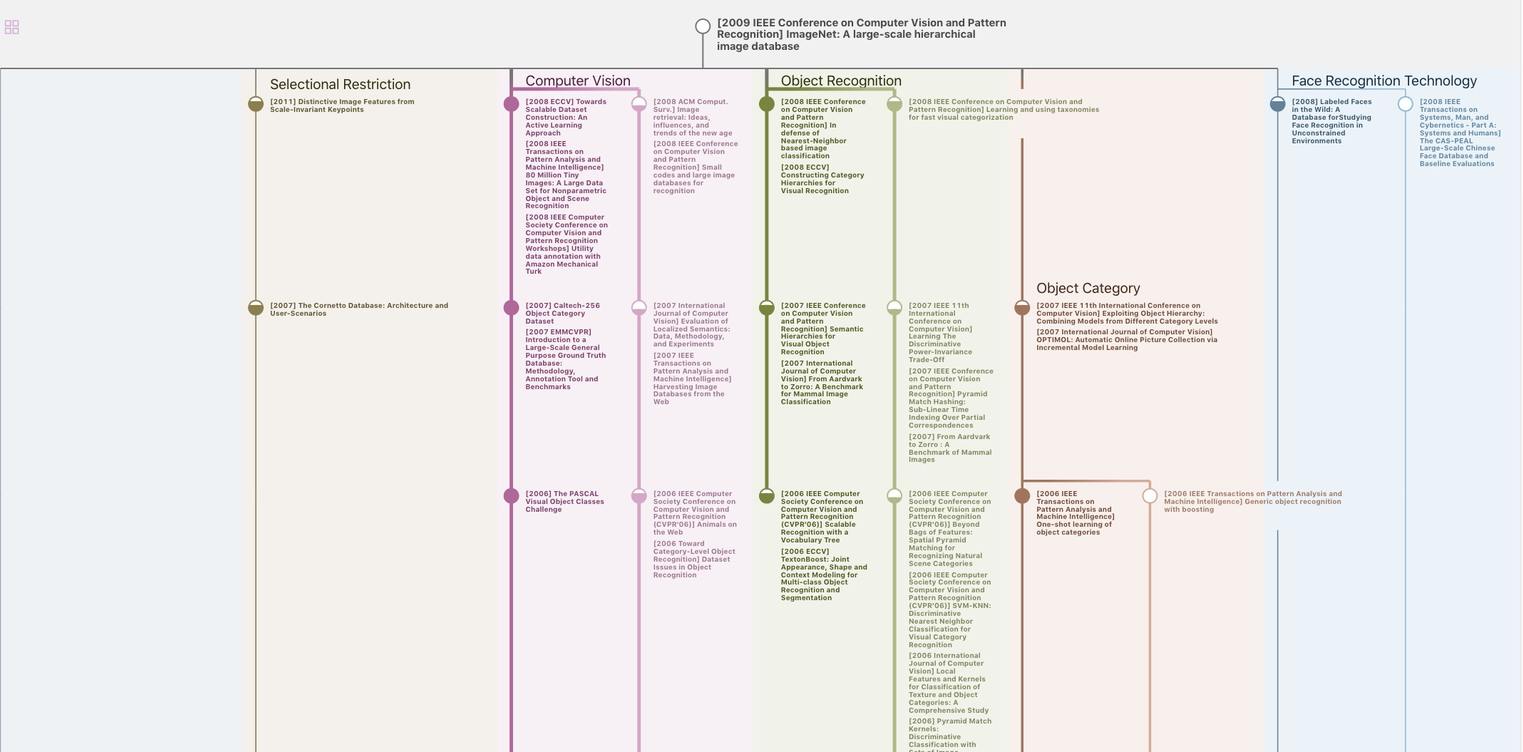
生成溯源树,研究论文发展脉络
Chat Paper
正在生成论文摘要