Comments on the importance of visualizing the distribution of pain-related data
European Journal of Pain(2023)
摘要
In a recent discussion on how to deal with data analysis issues initiated by reviewers of pain-related scientific manuscripts in the European Journal of Pain, a seemingly simple statistical issue was raised: two subsets of data in a paper had the same mean and standard deviation. A reviewer asked for a statistical test for or against the identity of the subset distributions. The authors insisted that if the mean and standard deviation were the same, this was sufficient evidence that the subsets of data were not significantly different. This prompted a discussion among pain researchers, who are not necessarily primarily from the field of data science, a discussion of the importance of carefully examining the distribution of pain-related data in a journal whose primary audience is pain researchers seems warranted. The problem of ‘equal means and equal standard deviations’ as sufficient evidence of the absence of a statistically significant difference has been formulated as an absolute truth. Therefore, it is sufficient to provide a counter-example to refute it. The above statement implicitly assumes that the distributions are normal or uniform. Consider the two distributions in Figure 1 for which the t-test (Student, 1908) refutes that the distributions are different at a p-value of nearly p = 1 (Figure 1). These data had the same overall means and standard deviations as the original data set (Figure 1), that is for the two data sets (‘Set1’ and ‘Set2’ in Figure 1), the overall means and standard deviations are equal at m = 2 and s = 7.2. A t-test (Student, 1908) shows no statistically significant difference. However, the difference in distribution is supported by a highly significant Kolmogorov–Smirnov test (Smirnov, 1948) (Figure 1f). The non-parametric Wilcoxon test (Mann & Whitney, 1947; Wilcoxon, 1945) supports the difference in the data sets. This concludes the discussion mentioned at the beginning: equal means and standard deviations do not mean that the data sets are not statistically significantly different. The above demonstration highlights the need for appropriate data visualization in scientific reports. However, such visualizations must be carefully selected. Figure 1c clearly shows that bar charts showing mean and standard deviation are inadequate. With such a visualization, the reader cannot judge whether a missing difference between two data sets is a valid result. Alternatives are shown, from typical distribution plots such as density plots or histograms to violin plots superimposed on individual data points, which are probably the best representation of the data among the options shown. It is highly advisable to present the (raw) research data visually, along with the usual summary statistics. Without this information, readers will simply have to take the authors' word that the data have been adequately analysed, although it has been shown that errors can occur (see below). The presentation of bar plots with error bars is definitely inadequate and should be abandoned. Regarding box plots, which are commonly used in scientific publications in pain research, it must be mentioned that they are not ideal. Although in the above example, the boxplot representation seemed to sufficiently illustrate the inequality of the two data sets, simple boxplots can distort the representation of the data in other cases. Figure 2 shows a pet example where boxplots are an inadequate visualization of data. Consider an ideal bimodal data set with means m1,2 = [0,1] and standard deviations s1,2 = [0.1, 0.1], with half of the data in mode 1 (weights w1,2 = [0.5, 0.5]). The violin plot shows exactly this information. The box plot, on the other hand, produces a meaningless visualization from which the true distribution cannot be deduced. Overlaying the box plot with single data points makes the error clear but emphasizes that the box plot was an inadequate visualization. To show that the example of a one-dimensional bimodal data set is not an artificial case with little relevance to real pain-related data, a data set of pain thresholds to heat after sensitization with capsaicin is shown. It comes from an in-house study of pain thresholds to different stimuli, with and without sensitization by local application of menthol or capsaicin, carried out on n = 125 healthy young volunteers (Doehring et al., 2011). Analysis of differences in heat pain thresholds between the unsensitized and sensitized conditions for a possible modal distribution using automated separation of one-dimensional Gaussian mixtures (Lötsch et al., 2022) revealed that a two-modal distribution provided the best fit to the distribution of the data (Figure 3). This is consistent with another study on different subjects, in whom modal separation of capsaicin sensitivity was reflected in genotype differences between subgroup members (Kringel et al., 2018). Evidence for a multimodal distribution is also found in other pain-related data. For example, cold thresholds in humans are clearly bimodally distributed in Figure 2 in Maier et al. (2010), although this has not been commented on. More generally, there is a need to visualize data sets. In a more general way, high-dimensional data sets from pain research can be visualized using a non-clustered heatmap (pixelmap) (Wilkinson & Friendly, 2009). A simple visual overview of high-dimensional data sets from pain research is shown in Figure 4 for two data sets collected in the context of the development of neuropathy following pharmacological cancer treatment. The columns of the graphs show the concentrations of d = 238 lipid markers and the rows show the probes taken from each patient before and after treatment. The rows are ordered in the order of the laboratory analyses, without clustering or another reordering (non-clustering heatmap). In cohort 2, the graph shows a pathology in the dataset. That is, from the 53rd sample onwards, the concentrations appear to be consistently different from the concentrations above. A machine-learning algorithm trained on the data from cohort 1 to identify whether a probe was taken before or after therapy failed to do so on the data from cohort 2. When the outlying samples were omitted, the algorithm was successful. A review of the laboratory workflow revealed that the cohort 2 sample was analysed in three batches. All aberrant samples and no others belonged to the third batch, suggesting pre-analytical mishandling of the samples or a technical error. This data error was not detected by standard laboratory quality control measures, nor was it apparent from the mean minimum and maximum variable values. The figure makes this immediately clear to researchers or reviewers and readers of such a publication. Without the data visualization, the error might have gone unnoticed in a scientific publication. In virtually every textbook of statistics, the first step in a statistical analysis is the formulation of a hypothesis about the data-generating process. In almost all cases, this already includes a hypothesis about the distribution of a variable. Often, this distribution hypothesis implicitly states that the data are normal, or at least so distributed that the seemingly assumption-free calculation of means and variances (standard deviations) yields meaningful values. It is shown above that this is not true in practical situations. Therefore, this paper calls for measuring some basic properties of the distribution before making a hypothesis. Measuring the distribution is different from analysing the data based on preconceived assumptions. For example, calculating means and variances implicitly assumes that these values exist and are meaningful for a particular set of data. For a binary variable with yes/no responses coded as [1, 0], it is possible to calculate a mean, but whether this is an appropriate result seems questionable and needs at least careful consideration in the actual data context. Again, visualizing the raw data in such a way that its distribution can be observed makes it clear to the reader what the authors of a scientific paper have observed and on what their conclusions are based. Measuring data characteristics, on the other hand, compares the given data to a standard. One of the best tools for doing this is the quantile–quantile (QQ) plot (Figure 5). This plot compares the quantiles of the data, usually on the y-axis, with the quantiles of a known distribution on the x-axis. Except for the largest and smallest parts of a distribution, measuring quantiles is a robust and hypothesis-free method. For example, comparing an empirical data set to the Gaussian (normal) distribution gives a first indication of whether the data can be used as is or whether some non-linear transformations are needed (Figure 5). In the introductory example, one could have hypothesized that the two sets of data would have the same means. If this were a reasonable hypothesis, the study would have succeeded in testing it. However, if the hypothesis is that the two data sets are not statistically significantly different, the conclusions are different as shown above. It seems reasonable to look at the distribution of the data and not make an assumption about it and calculate a mean when it is not appropriate. For example, it should be noted that calculating means and standard deviations per se is not appropriate for the distributions B through D in Figure 5. Measurement plots must be distinguished from plots that already impose a model of the data on the visualization. This is sometimes not transparent and can lead to incorrect results. For example, the density plot shown in Figure 2a adequately represents the Gaussian mixture of the two normally distributed variables with means [0, 1] and standard deviations [0.1, 0.1]. However, using the same plot on a binary variable [0, 1] results in the same data visualization, only this time it is incorrect (Figure 6). The probability density function provided in the R standard density plotting routine is a kernel density function that smooths the data, making a binary variable look like two Gaussians. More appropriate data visualizations include the standard histogram or another type of probability density estimator, such as the Pareto density estimation (PDE) (Ultsch, 2003), which is also a kernel density but uses a different algorithm; however, it is not a standard in statistical or plotting software. It should be noted that histograms, like all examples in Figure 6, also make assumptions about the data by applying a certain bin width, the default settings just happen to be better suited for the binary data example, which is also true for the PDE. The present visualizations were performed by programming code in the R language (Ihaka & Gentleman, 1996) using the R software package (R Development Core Team, 2008) (version 4.2.2 for Linux), which is available for free on the Comprehensive R Archive Network at https://cran.r-project.org. However, both the figures and the statistics can be produced using virtually any statistical software package, whether coding-driven or point-and-click, although the latter usually has limited data visualization options and less flexibility. The initial question of whether two sets of data with the same means and standard deviations can be statistically different, and, whether a statistical test is even necessary in such cases, was answered with a clear ‘yes’. The statistical background for this is, of course, available in the pain research community. The above comments underline that adequate visualization of the data is one of the keys to a correct analysis. Before making any (implicit) hypotheses about the data, it is necessary to make measuring visualizations such as QQ plots or pixel matrix plots as examples in this commentary. While statistical signals that the distribution is supposedly not normal are sometimes missed and non-parametric or parametric tests are performed without regard to them, the likelihood that authors, reviewers or readers of research reports will overlook such errors is greatly reduced if the raw data are presented in such a way that their distribution is clear. Therefore, reporting standard descriptive statistics falls short if it is not accompanied by an informative visualization of the (raw) data. In summary, before ‘step one’ in a scientific analysis of data, namely the formulation of a hypothesis, step zero should be the use of measurement visualizations to avoid (implicit) false hypotheses or assumptions about the nature of the given data. Open Access funding enabled and organized by Projekt DEAL. Data S1. Please note: The publisher is not responsible for the content or functionality of any supporting information supplied by the authors. Any queries (other than missing content) should be directed to the corresponding author for the article.
更多查看译文
关键词
pain‐related,data
AI 理解论文
溯源树
样例
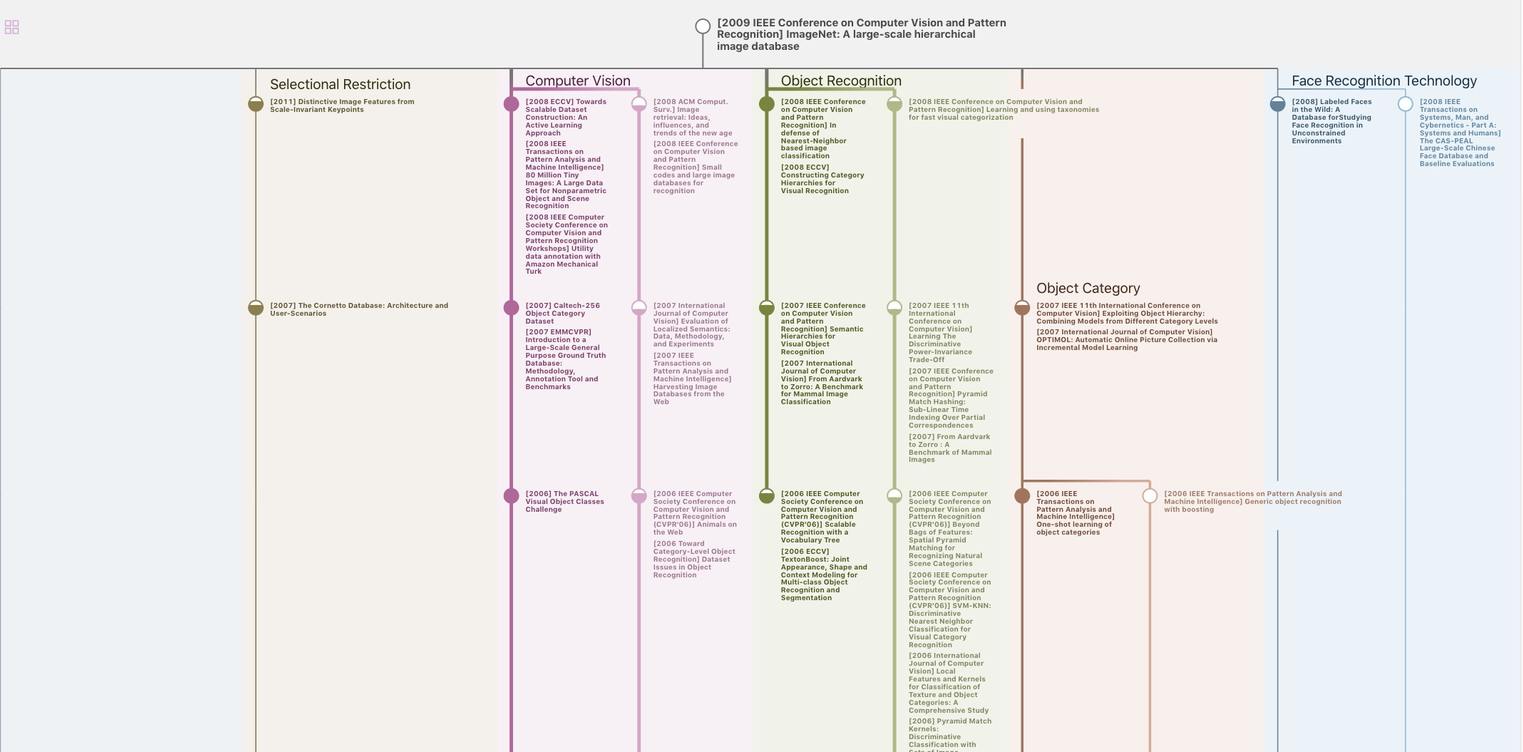
生成溯源树,研究论文发展脉络
Chat Paper
正在生成论文摘要