Novel, Configurable Approximate Floating-point Multipliers for Error-Resilient Applications
2023 24th International Symposium on Quality Electronic Design (ISQED)(2023)
摘要
By exploiting the gap between the user’s accuracy requirement and the hardware’s accuracy capability, approximate circuit design offers enormous gains in efficiency for a minor accuracy loss. In this paper, we propose two approximate floating point multipliers (AxFPMs), named DTCL (decomposition, truncation and chunk-level leading-one quantization) and TDIL (truncation, decomposition and ignoring LSBs). Both AxFPMs introduce approximation in mantissa multiplication. DTCL works by rounding and truncating LSBs and quantizing each chunk. TDIL works by truncating LSBs and ignoring the least important terms in the multiplication. Further, both techniques multiply more significant terms by simply exponent addition or shift-and-add operations. These AxFPMs are configurable and allow trading off accuracy with hardware overhead. Compared to exact floating-point multiplier (FPM), DTCL(4,8,8) reduces area, energy and delay by 11.0%, 69% and 61%, respectively, while incurring a mean relative error of only 2.37%. On a range of approximate applications from machine learning, deep learning and image processing domains, our AxFPMs greatly improve efficiency with only minor loss in accuracy. For example, for image sharpening and Gaussian smoothing, all DTCL and TDIL variants achieve a PSNR of more than 30dB. The source-code is available at https://github.com/CandleLabAI/ApproxFloatingPointMultiplier.
更多查看译文
关键词
approximate circuit design,approximate floating point multipliers,AxFPM,decomposition truncation and chunk-level leading-one quantization,DTCL,error-resilient applications,exponent addition,hardware overhead,LSB,mantissa multiplication,shift-and-add operations,TDIL
AI 理解论文
溯源树
样例
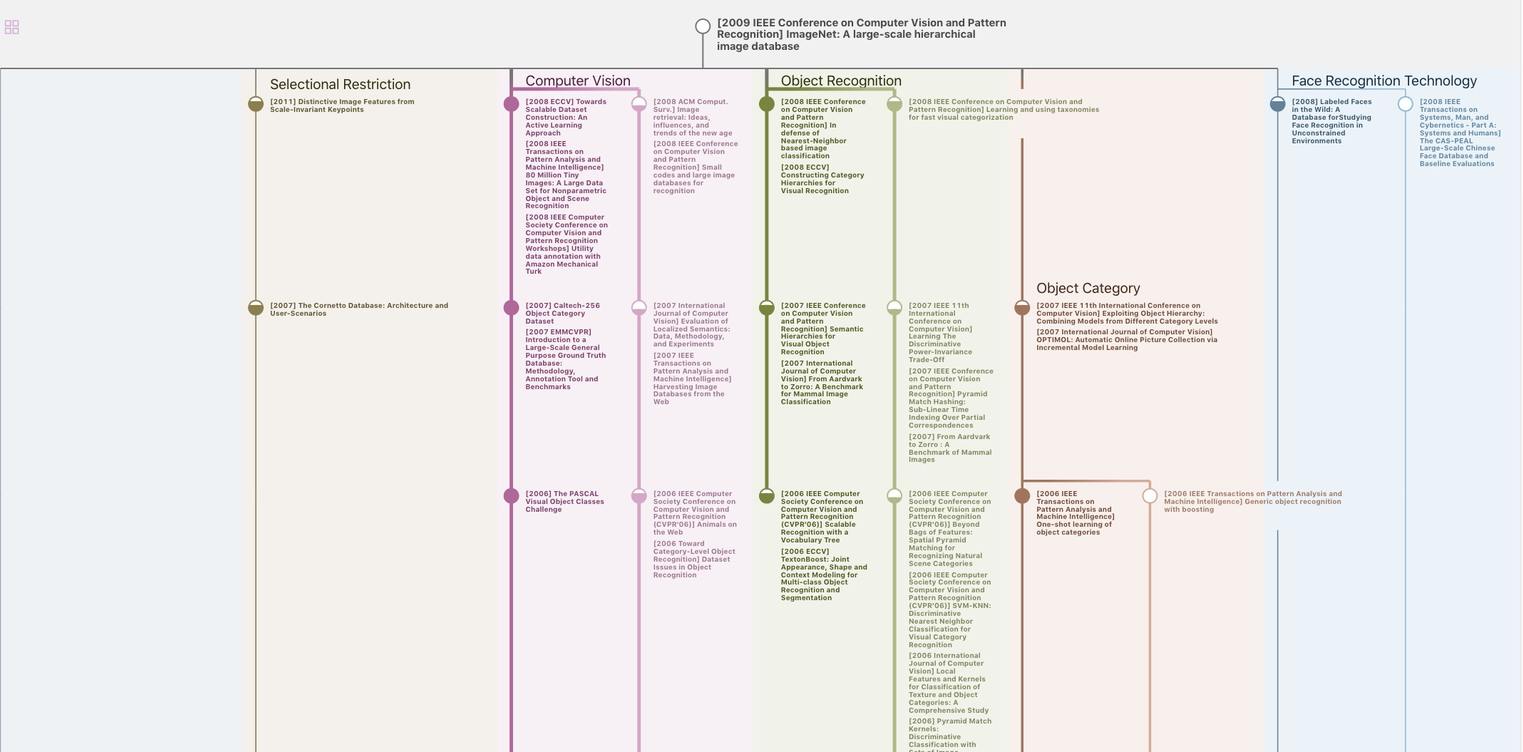
生成溯源树,研究论文发展脉络
Chat Paper
正在生成论文摘要