A new concatenated Multigraph Neural Network for IoT intrusion detection
Internet of Things(2023)
摘要
The last few years have seen a high volume of sophisticated cyber-attacks leading to financial instability and privacy breaches. This reveals the need for a Network Intrusion Detection System (NIDS) that is more robust, effective, and capable of learning complex relations of network flows. Existing machine learning approaches are barely effective for picking new attacks in IoT traffic, primarily due to treating flows independently. Recently, Graph Neural Networks (GNNs) have gained popularity due to their ability to model underlying topology in the form of nodes and edges. We propose a GNN-based NIDS that maximizes the possibility of involving structural characteristics of normal and malicious network traffic. We construct a multi-edged graph structure that captures full communication between any pair of IoT nodes at the edge level. This novel GNN model combines the benefits of spectral and spatial GNNs tailored to process and learn from complex multigraph-structured information. The proposed GNN model is adjusted to work on multi-edges and multi-dimensional edge features. Extensive experimental analysis using evaluation metrics is carried out on four benchmark datasets, demonstrating the competence of our proposed scheme compared to the state-of-the-art GNN models. Our proposed model shows about 2% to 5% improvement in accuracy, precision, F1 and recall offering less training time and model size in the considered datasets. The proposed model has achieved high accuracy ranging from 98.91% to 99.96%, with precision ranging from 98.86 to 99.96, recall ranging from 98.91% to 99.96%, and F1-score ranging from 98.74% to 99.95% in the four benchmark datasets.
更多查看译文
关键词
Graph Neural Networks, Network Intrusion Detection System, Internet of Things, Graph Convolution Networks, Graph attention
AI 理解论文
溯源树
样例
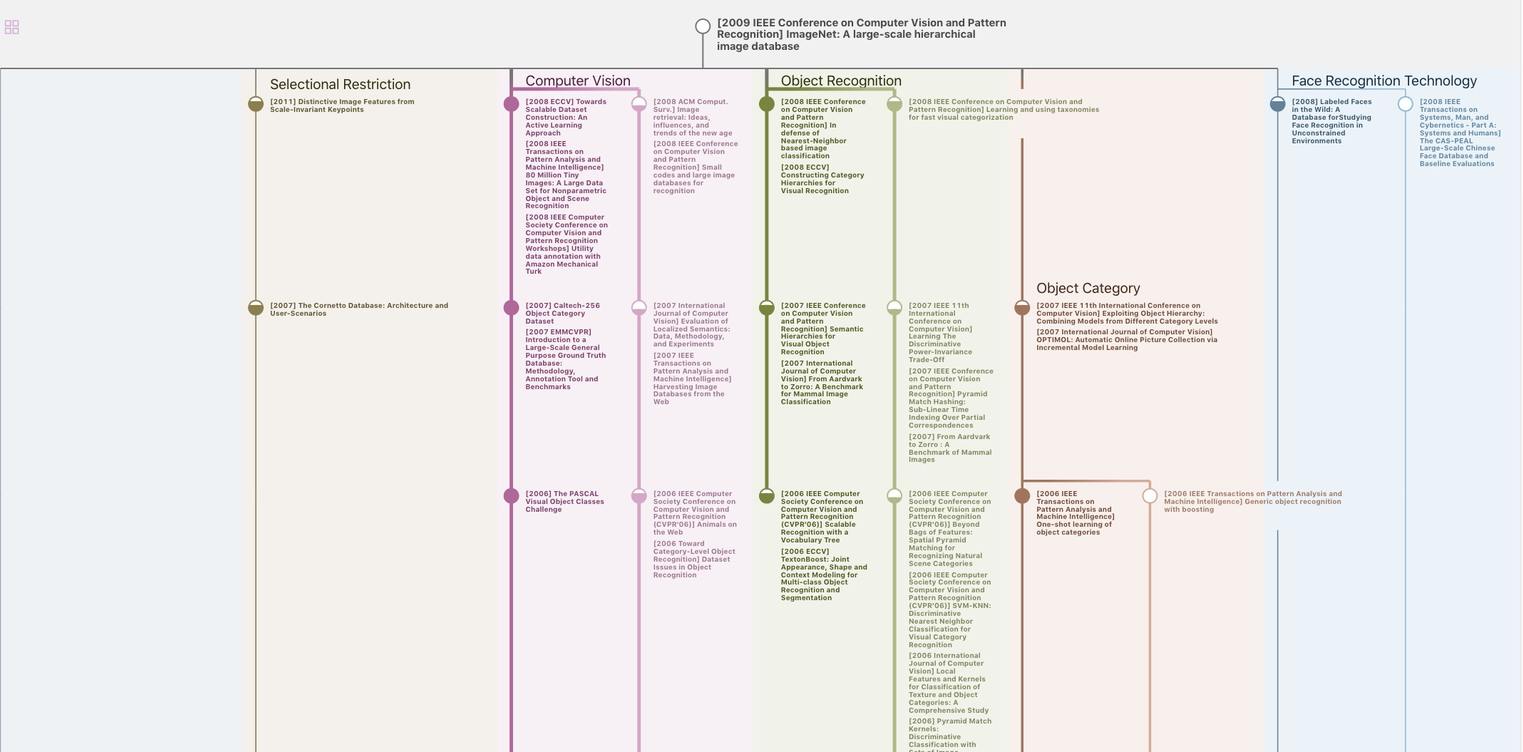
生成溯源树,研究论文发展脉络
Chat Paper
正在生成论文摘要