Relating Implicit Bias and Adversarial Attacks through Intrinsic Dimension
CoRR(2023)
摘要
Despite their impressive performance in classification, neural networks are known to be vulnerable to adversarial attacks. These attacks are small perturbations of the input data designed to fool the model. Naturally, a question arises regarding the potential connection between the architecture, settings, or properties of the model and the nature of the attack. In this work, we aim to shed light on this problem by focusing on the implicit bias of the neural network, which refers to its inherent inclination to favor specific patterns or outcomes. Specifically, we investigate one aspect of the implicit bias, which involves the essential Fourier frequencies required for accurate image classification. We conduct tests to assess the statistical relationship between these frequencies and those necessary for a successful attack. To delve into this relationship, we propose a new method that can uncover non-linear correlations between sets of coordinates, which, in our case, are the aforementioned frequencies. By exploiting the entanglement between intrinsic dimension and correlation, we provide empirical evidence that the network bias in Fourier space and the target frequencies of adversarial attacks are closely tied.
更多查看译文
关键词
adversarial attacks,implicit bias,intrinsic
AI 理解论文
溯源树
样例
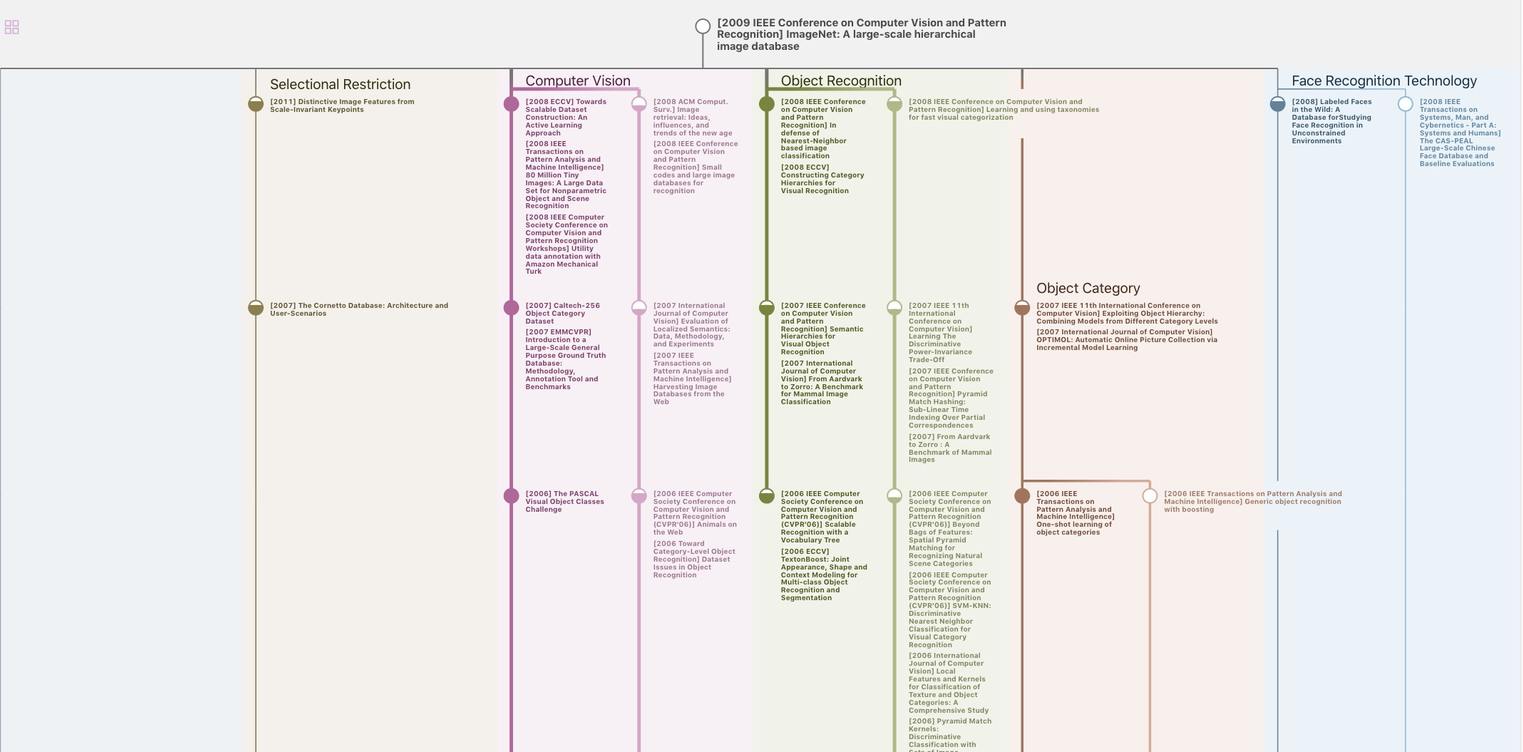
生成溯源树,研究论文发展脉络
Chat Paper
正在生成论文摘要