Fairness in Streaming Submodular Maximization over a Matroid Constraint
CoRR(2023)
摘要
Streaming submodular maximization is a natural model for the task of selecting a representative subset from a large-scale dataset. If datapoints have sensitive attributes such as gender or race, it becomes important to enforce fairness to avoid bias and discrimination. This has spurred significant interest in developing fair machine learning algorithms. Recently, such algorithms have been developed for monotone submodular maximization under a cardinality constraint. In this paper, we study the natural generalization of this problem to a matroid constraint. We give streaming algorithms as well as impossibility results that provide trade-offs between efficiency, quality and fairness. We validate our findings empirically on a range of well-known real-world applications: exemplar-based clustering, movie recommendation, and maximum coverage in social networks.
更多查看译文
关键词
streaming submodular maximization,fairness
AI 理解论文
溯源树
样例
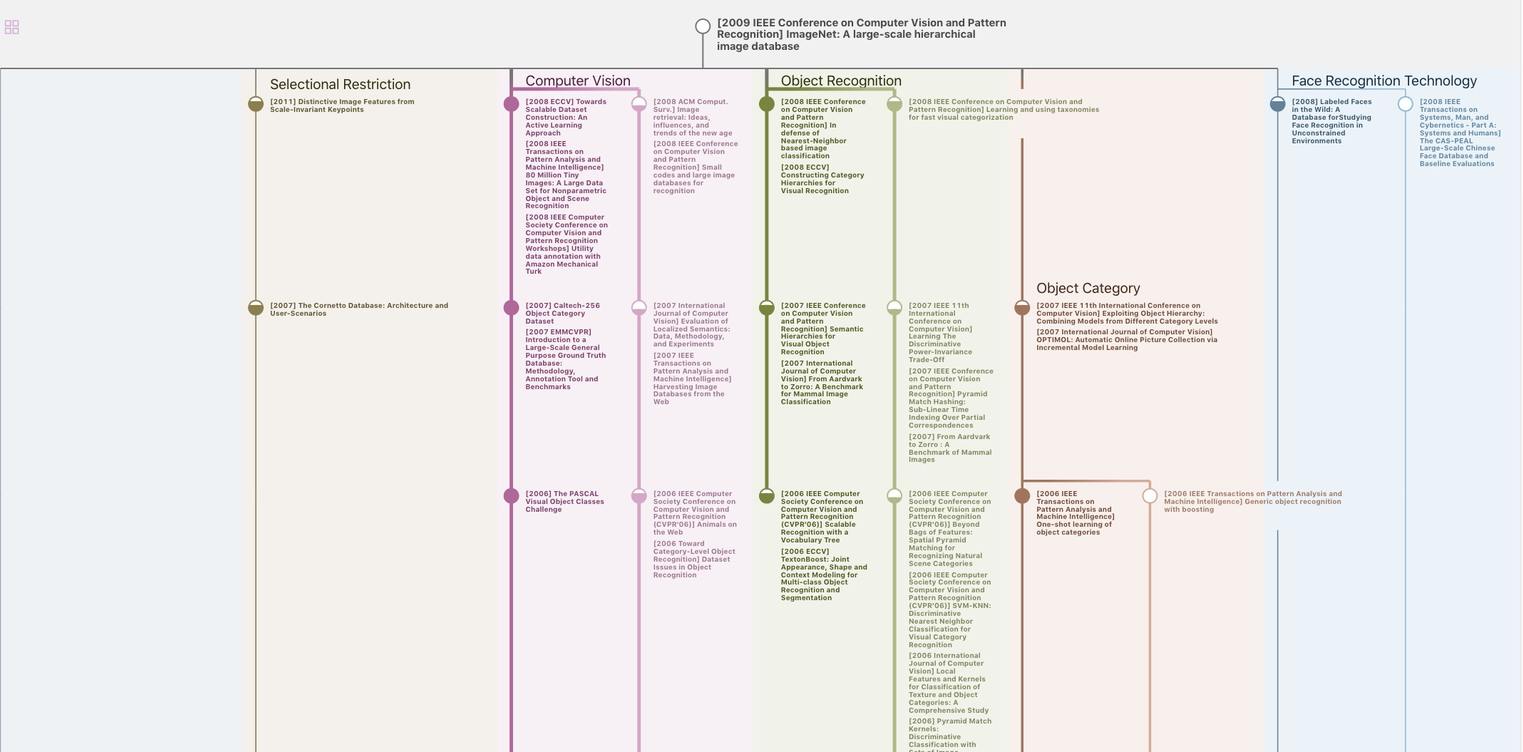
生成溯源树,研究论文发展脉络
Chat Paper
正在生成论文摘要