Block Coordinate Descent on Smooth Manifolds
arxiv(2023)
摘要
Block coordinate descent is an optimization paradigm that iteratively updates one block of variables at a time, making it quite amenable to big data applications due to its scalability and performance. Its convergence behavior has been extensively studied in the (block-wise) convex case, but it is much less explored in the non-convex case. In this paper we analyze the convergence of block coordinate methods on non-convex sets and derive convergence rates on smooth manifolds under natural or weaker assumptions than prior work. Our analysis applies to many non-convex problems (e.g., generalized PCA, optimal transport, matrix factorization, Burer-Monteiro factorization, outlier-robust estimation, alternating projection, maximal coding rate reduction, neural collapse, adversarial attacks, homomorphic sensing), either yielding novel corollaries or recovering previously known results.
更多查看译文
AI 理解论文
溯源树
样例
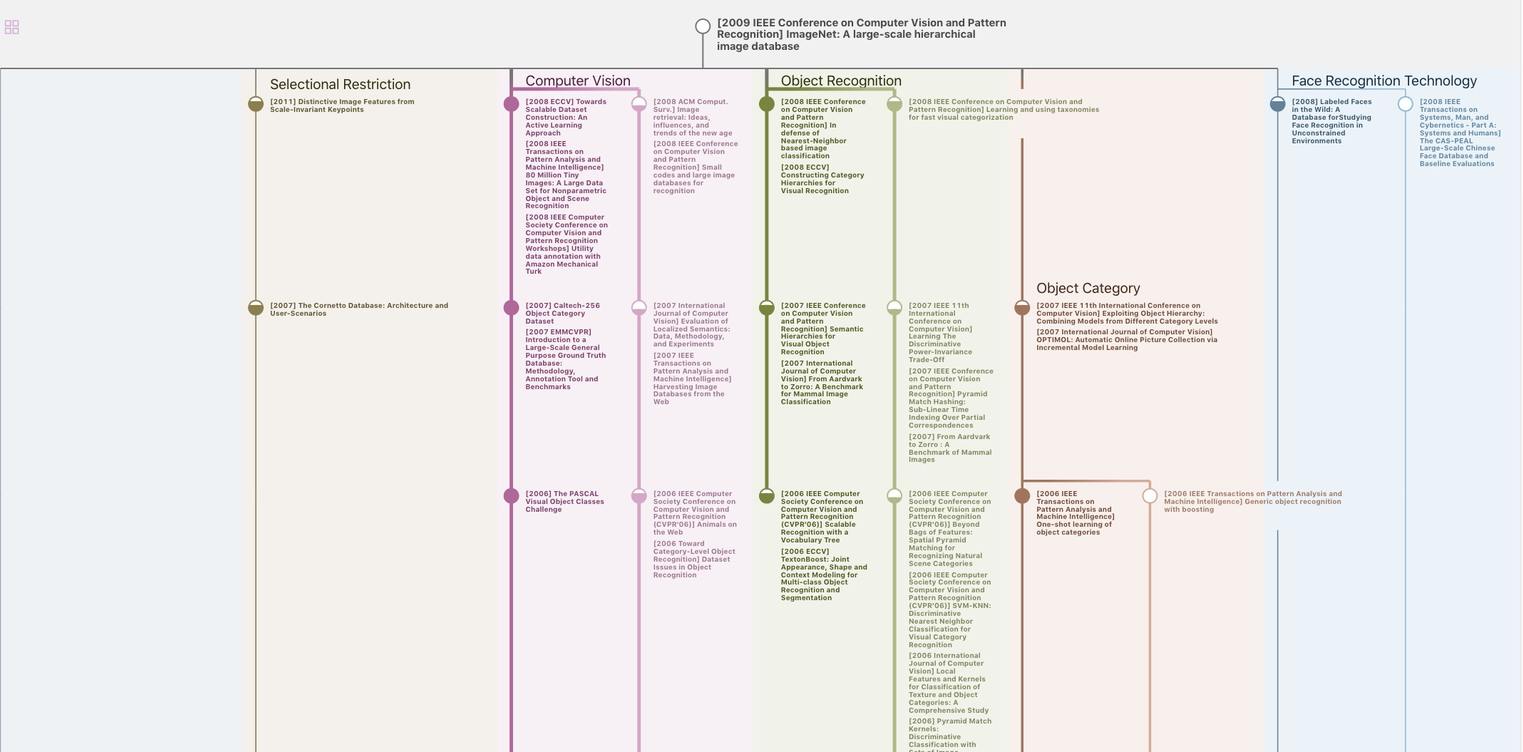
生成溯源树,研究论文发展脉络
Chat Paper
正在生成论文摘要