DirecT2V: Large Language Models are Frame-Level Directors for Zero-Shot Text-to-Video Generation
CoRR(2023)
摘要
In the paradigm of AI-generated content (AIGC), there has been increasing
attention to transferring knowledge from pre-trained text-to-image (T2I) models
to text-to-video (T2V) generation. Despite their effectiveness, these
frameworks face challenges in maintaining consistent narratives and handling
shifts in scene composition or object placement from a single abstract user
prompt. Exploring the ability of large language models (LLMs) to generate
time-dependent, frame-by-frame prompts, this paper introduces a new framework,
dubbed DirecT2V. DirecT2V leverages instruction-tuned LLMs as directors,
enabling the inclusion of time-varying content and facilitating consistent
video generation. To maintain temporal consistency and prevent mapping the
value to a different object, we equip a diffusion model with a novel value
mapping method and dual-softmax filtering, which do not require any additional
training. The experimental results validate the effectiveness of our framework
in producing visually coherent and storyful videos from abstract user prompts,
successfully addressing the challenges of zero-shot video generation.
更多查看译文
关键词
large language models,frame-level,zero-shot,text-to-video
AI 理解论文
溯源树
样例
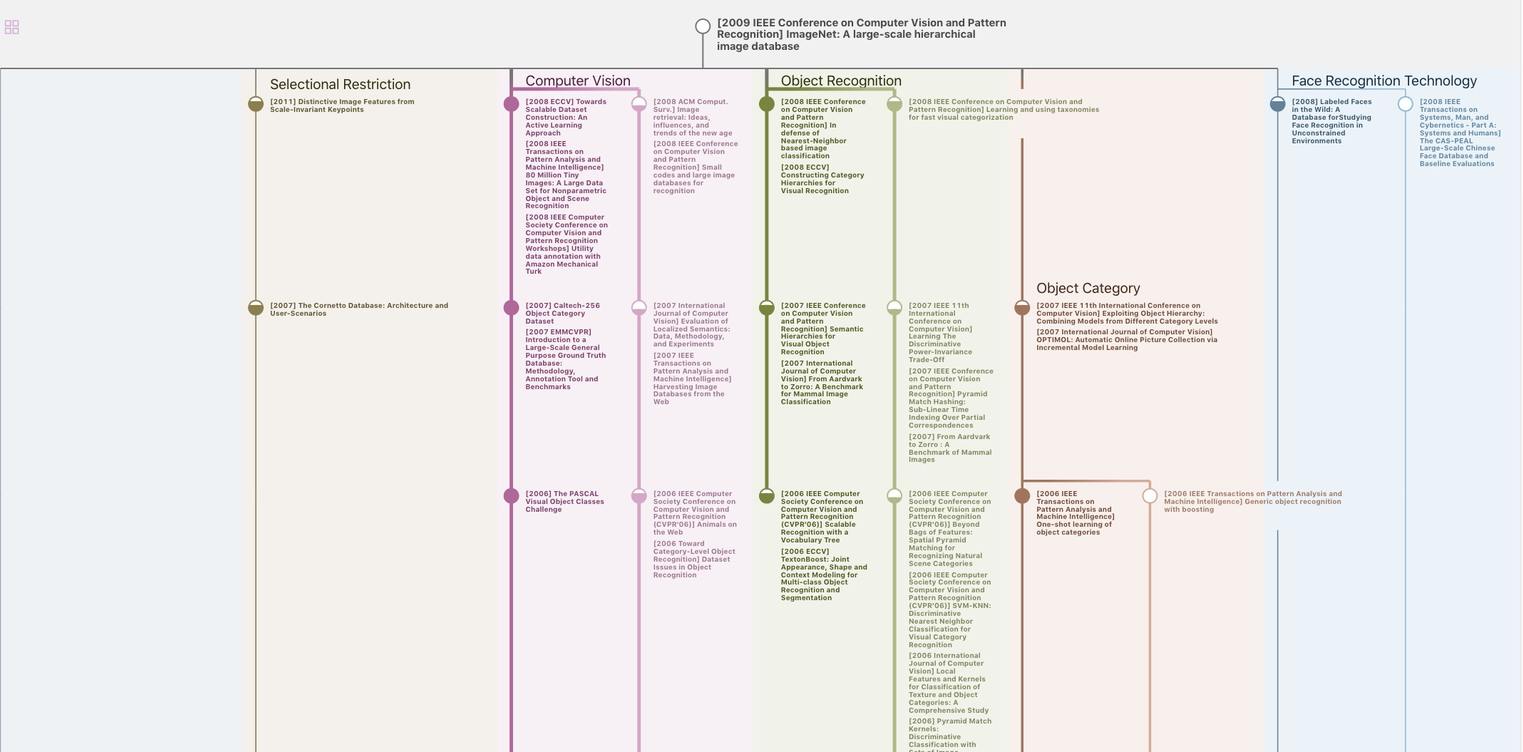
生成溯源树,研究论文发展脉络
Chat Paper
正在生成论文摘要