Pre-training Multi-task Contrastive Learning Models for Scientific Literature Understanding.
CoRR(2023)
摘要
Scientific literature understanding tasks have gained significant attention due to their potential to accelerate scientific discovery. Pre-trained language models (LMs) have shown effectiveness in these tasks, especially when tuned via contrastive learning. However, jointly utilizing pre-training data across multiple heterogeneous tasks (e.g., extreme classification, citation prediction, and literature search) remains largely unexplored. To bridge this gap, we propose a multi-task contrastive learning framework, SciMult, with a focus on facilitating common knowledge sharing across different scientific literature understanding tasks while preventing task-specific skills from interfering with each other. To be specific, we explore two techniques -- task-aware specialization and instruction tuning. The former adopts a Mixture-of-Experts Transformer architecture with task-aware sub-layers; the latter prepends task-specific instructions to the input text so as to produce task-aware outputs. Extensive experiments on a comprehensive collection of benchmark datasets verify the effectiveness of our task-aware specialization strategy in various tasks, where we outperform state-of-the-art scientific LMs.
更多查看译文
关键词
literature,learning,models,scientific,pre-training,multi-task
AI 理解论文
溯源树
样例
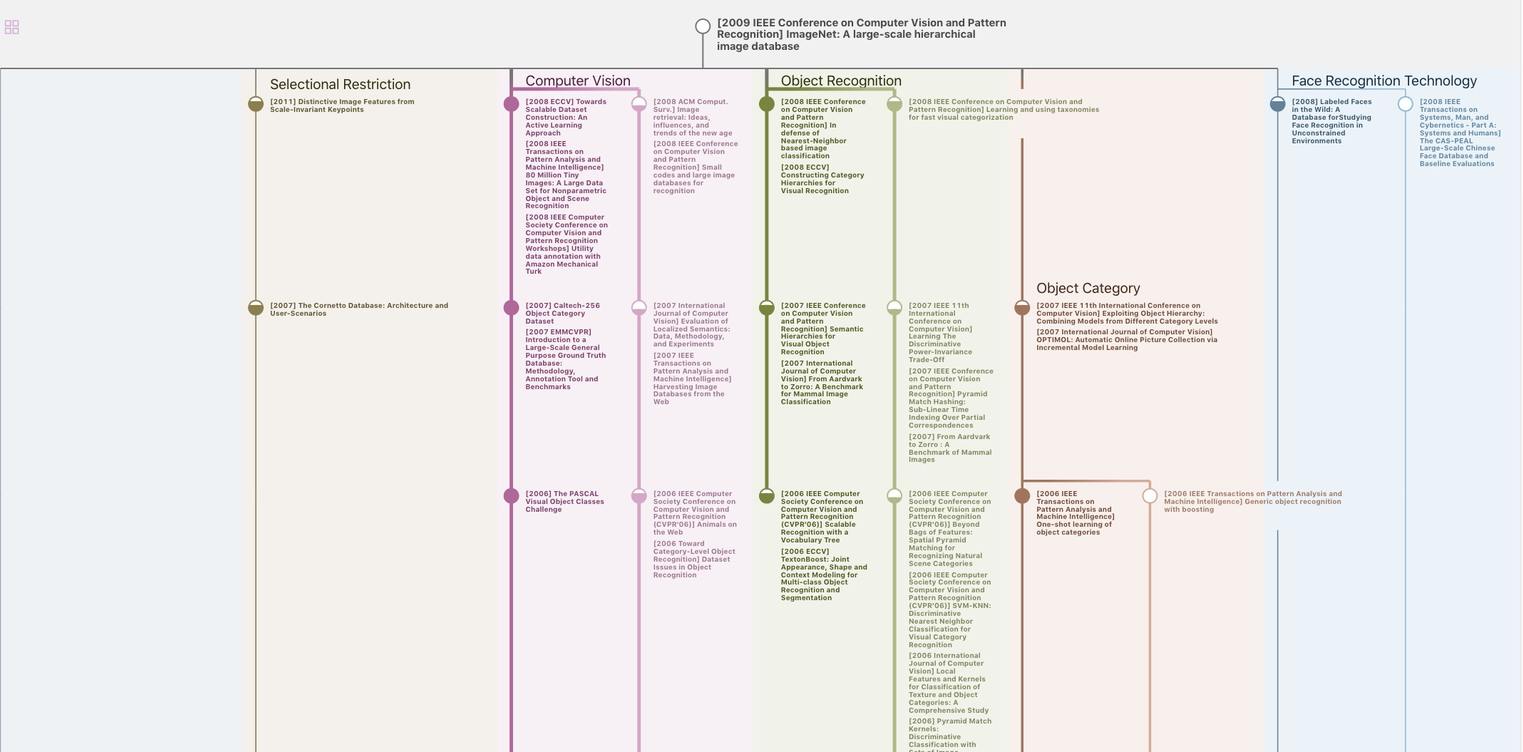
生成溯源树,研究论文发展脉络
Chat Paper
正在生成论文摘要