Learning Remote Sensing Object Detection With Single Point Supervision
IEEE TRANSACTIONS ON GEOSCIENCE AND REMOTE SENSING(2024)
摘要
Pointly supervised object detection (PSOD) has attracted considerable interest due to its lower labeling cost when compared to box-level supervised object detection. However, the complex scenes and densely packed and dynamic-scale objects in remote-sensing (RS) images hinder the development of PSOD methods in the RS field. In this article, we make the first attempt to achieve RS object detection with single-point supervision and propose a PSOD method tailored for RS images. Specifically, we design a point label upgrader (PLUG) to generate pseudo-box labels from single-point labels and then use the pseudo-boxes to supervise the optimization of existing detectors. Moreover, to handle the challenge of the densely packed objects in RS images, we propose a sparse feature-guided semantic prediction (SemPred) module that can generate high-quality semantic maps by fully exploiting informative cues from sparse objects. Extensive ablation studies on the DOTA dataset have validated the effectiveness of our method. Our method can achieve significantly better performance when compared to state-of-the-art image-level and point-level supervised detection methods and reduce the performance gap between PSOD and box-level supervised object detection. The code is available at https://github.com/heshitian/PLUG.
更多查看译文
关键词
Remote sensing (RS),single-point supervised object detection (PSOD),sparse guided feature aggregation
AI 理解论文
溯源树
样例
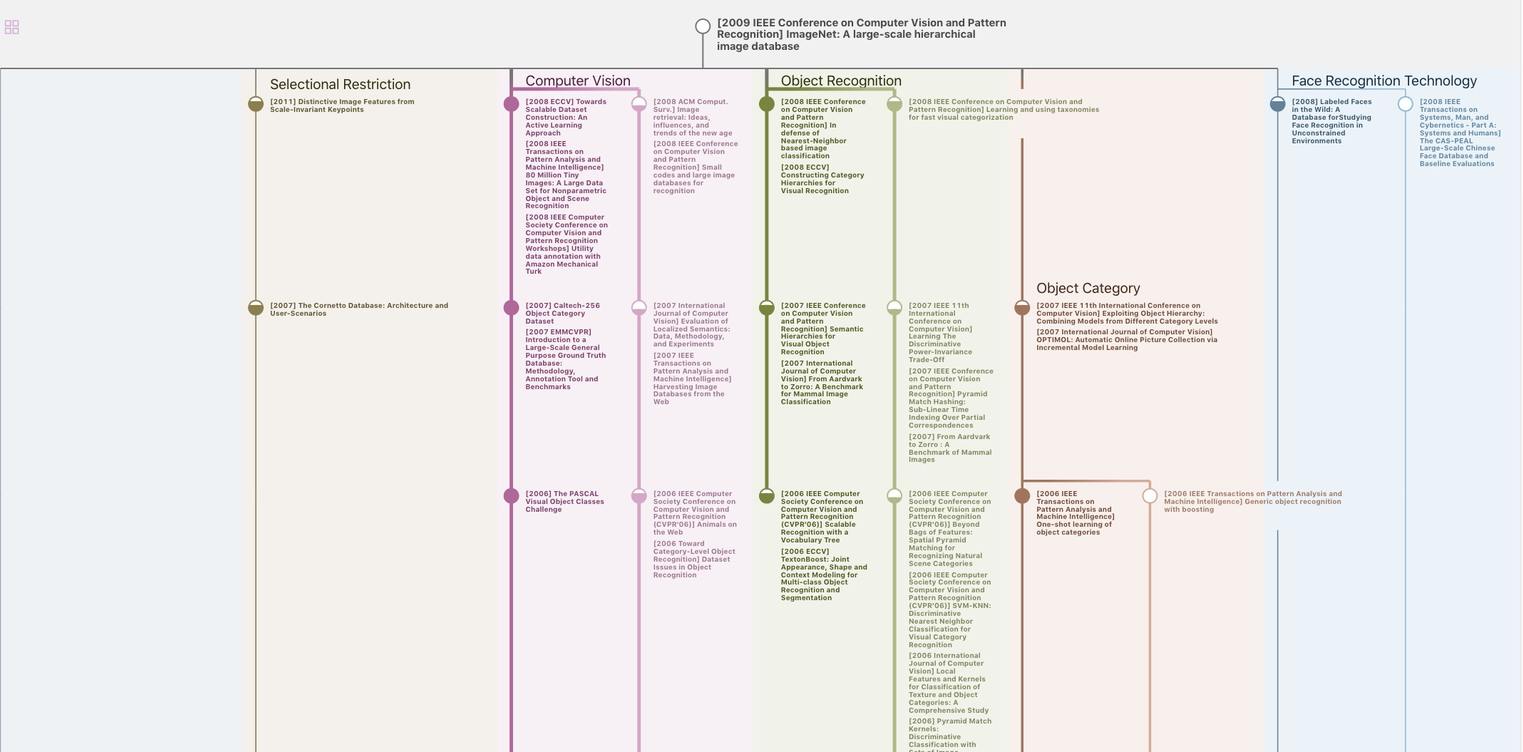
生成溯源树,研究论文发展脉络
Chat Paper
正在生成论文摘要