PMSM Combination Modeling for Multiparameter Estimation Using Bayesian Learning With Inverter Distortion Cancellation and Temperature Compensation
IEEE Transactions on Energy Conversion(2023)
摘要
Permanent magnet synchronous machine (PMSM) drives with better efficiency are highly demanded, and accurate flux linkage and inductance models or maps are critical to achieve such drives. However, precise modeling and estimation of these parameters should employ redundant data and are affected by magnetic saturation and inverter distortion. This paper firstly derives a flux linkage combination model from machine model for flux linkage and inductance estimation, in which inverter distortion is cancelled and thus inverter influence is minimized for performance improvement. To consider magnetic saturation, radial basis functions are employed to model the nonlinear flux linkages with a small number of relevance vectors, which can effectively depict the nonlinear variation. Bayesian learning approach is then explored to estimate the sparse coefficients of the flux linkage model in the context of radial basis functions, which can deal with non-Gaussian noise to improve the estimation accuracy and guarantee flux linkage model with better computation efficiency and less memory occupation. Moreover, temperature effect is considered to ensure the model accuracy under temperature rise. The proposed approach is validated with experiments and comparisons on a laboratory PMSM drive.
更多查看译文
关键词
Flux linkage modeling,inverter distortion cancellation,multiparameter estimation,sparse Bayesian learning,temperature compensation
AI 理解论文
溯源树
样例
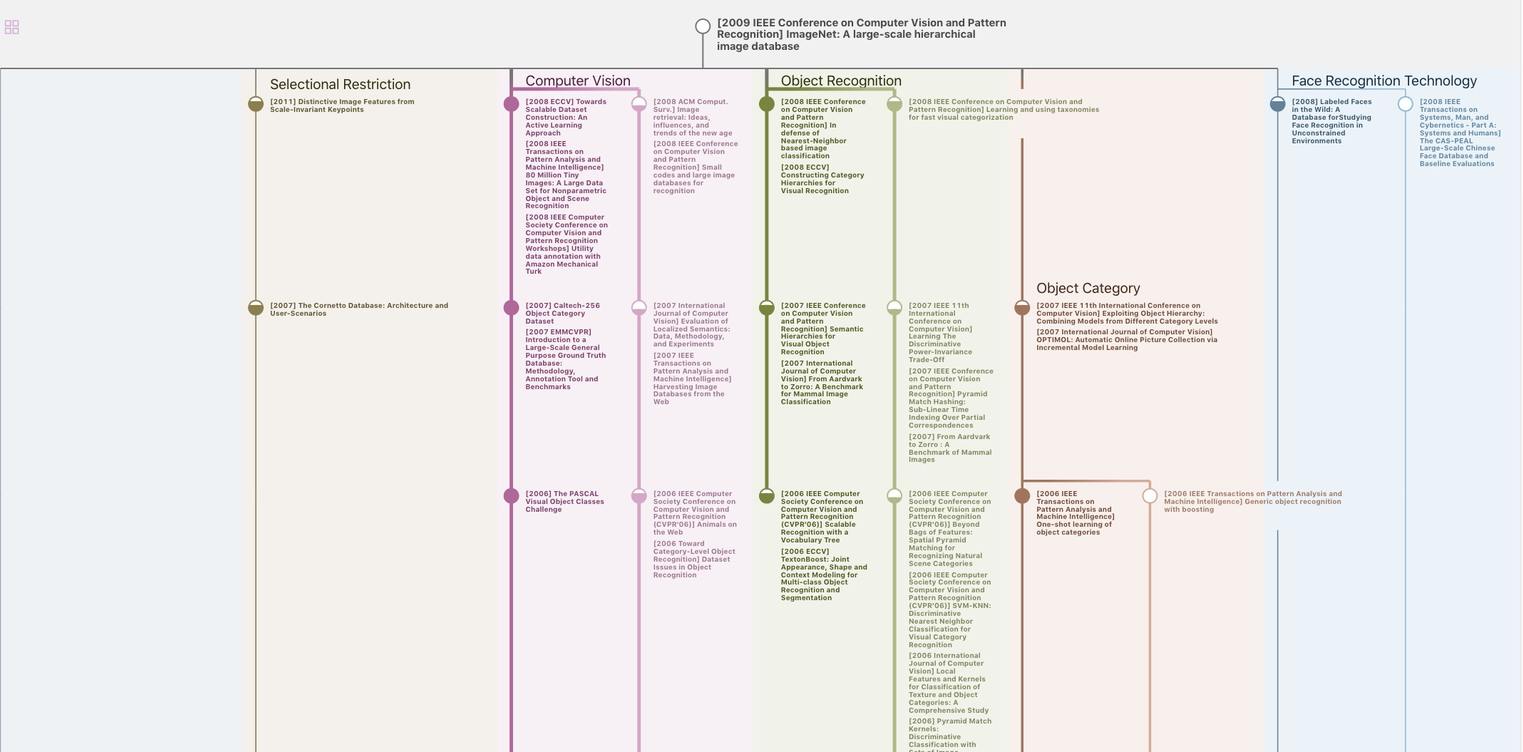
生成溯源树,研究论文发展脉络
Chat Paper
正在生成论文摘要