Data imputation strategies for process optimization of laser powder bed fusion of Ti6Al4V using machine learning
Materials Science in Additive Manufacturing(2023)
摘要
A database linking process parameters and material properties for additive manufacturing enables the performance of the material to be determined based on the process parameters, which are useful in the design and fabrication stage of a product. The data, however, are often incomplete as each individual research work focused on certain process parameters and material properties due to the wide range of variables available. Imputation of missing data is thus required to complete the material library. In this work, we attempt to collate the data of Ti6Al4V, a popular alloy used in aerospace and biomedical industries, fabricated using powder bed fusion, or commonly known as selective laser melting (SLM). Various imputation techniques of missing data of the SLM Ti6Al4V dataset, such as the k-nearest neighbor (kNN), multivariate imputation by chained equations, and graph imputation neural network (GINN) are investigated in this article. It was observed that kNN performed better in imputing variables related to process parameters, whereas GINN performed better in variables related to material properties. To further improve the quality of imputation, a strategy to use the median of the imputed values obtained from the three models has resulted in significant improvement in terms of the relative mean square error. Self-organizing map was used to visualize the relationship among the process parameters and the material properties.
更多查看译文
关键词
laser powder bed fusion,ti6al4v,process optimization,machine learning
AI 理解论文
溯源树
样例
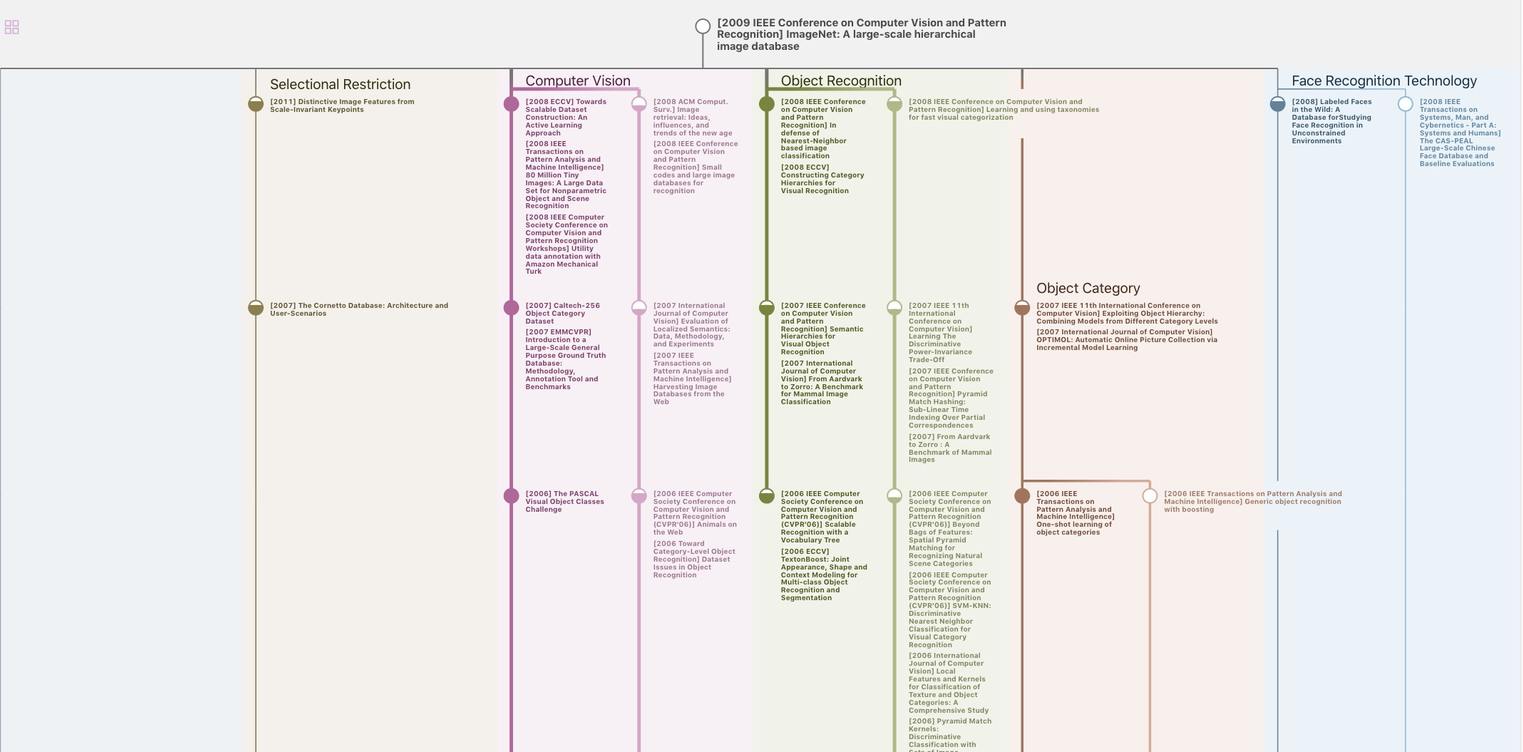
生成溯源树,研究论文发展脉络
Chat Paper
正在生成论文摘要