Deep Active Learning-Enabled Cost-Effective Electricity Theft Detection in Smart Grids
IEEE TRANSACTIONS ON INDUSTRIAL INFORMATICS(2024)
摘要
In industrial informatics-enabled smart grids, machine learning approaches have exhibited high potential in data-driven electricity theft detection (ETD), whereas none of the existing studies pay sufficient attention to the high costs of manually labeling massive sensing data during learning data preparation. To address this defect, this article develops a cost-effective data-driven ETD approach that significantly reduces the data labeling costs without sacrificing the reliability of ETD. Specifically, the approach is systematically realized via an intelligent deep active learning (DAL) scheme. By seamlessly incorporating convolutional neural network (CNN) learning with Monte Carlo dropout-based Bayesian active query, the DAL scheme efficiently selects the most valuable instances for ETD model training. In this way, the proposed approach is able to derive a reliable CNN-based ETD model with limited labeled learning instances, thus largely reducing the data labeling costs. Experimental test results on an actual ETD dataset provided by the State Grid Corporation of China extensively illustrate the efficacy of the proposed approach.
更多查看译文
关键词
Active learning (AL),deep learning (DL),dropout,electricity theft detection (ETD),smart grid data analytics,smart meter
AI 理解论文
溯源树
样例
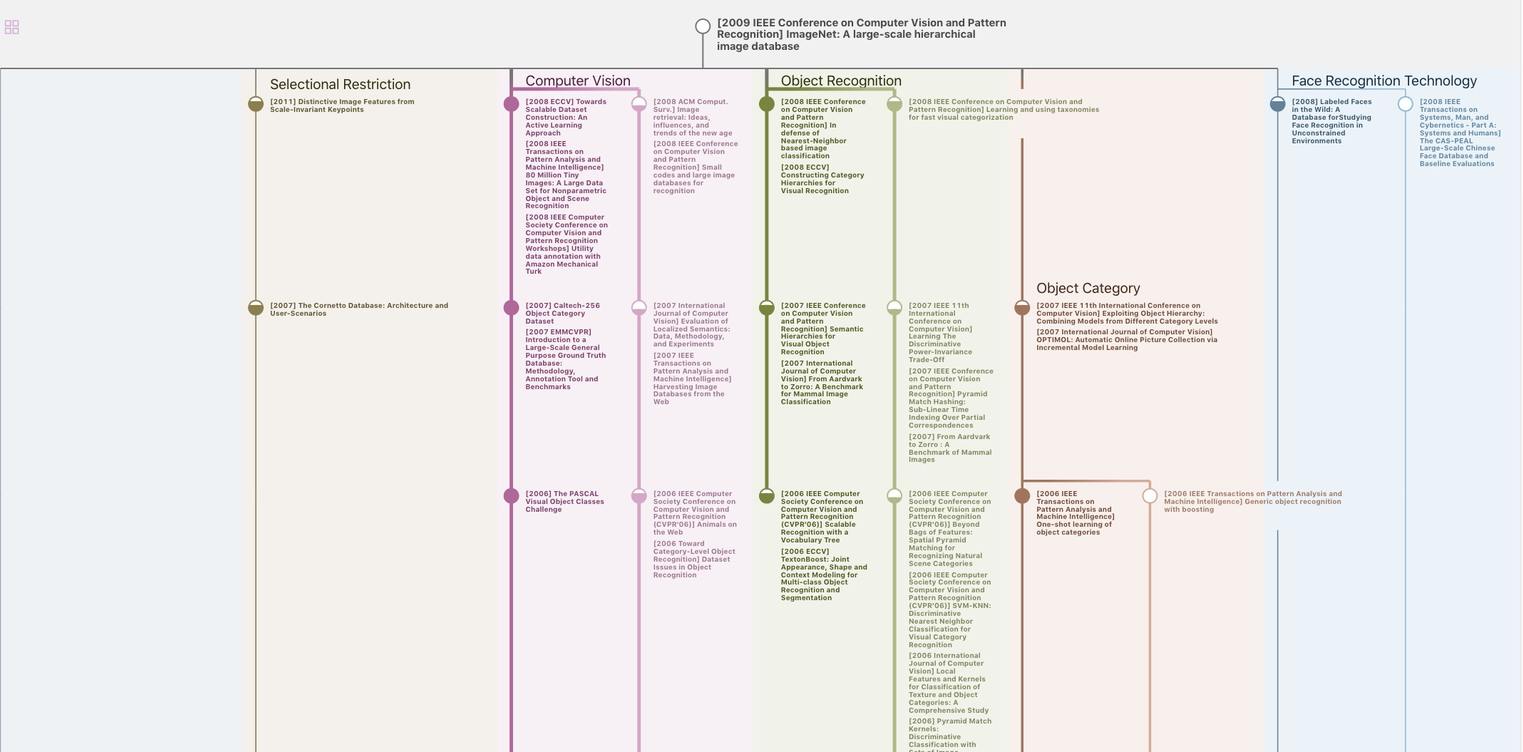
生成溯源树,研究论文发展脉络
Chat Paper
正在生成论文摘要