Portfolio Selection via Graph-Aware Gaussian Processes With Generalized Gaussian Likelihood.
IEEE Transactions on Artificial Intelligence(2024)
摘要
Portfolio selection aims to manage the allocation of wealth among different assets, which remains to be a fundamental and challenging financial task. Markowitz's mean-variance analysis is one of the most well-known and widely adopted techniques for this problem. However, it requires accurate estimations of both the mean and variance of the return, while predicting the variance is particularly difficult. In this paper, we propose a novel portfolio selection strategy based on a graph-aware Gaussian process model equipped with generalized Gaussian distribution likelihood. Our method unleashes the potential of mean-variance analysis by exploiting the Gaussian process model's ability in capturing uncertainty, and the graph information is also incorporated so that correlation among different assets can be utilized. We notice that most existing financial models assume the returns follow the log-normal distribution, whereas we observe that it cannot perfectly explain real-world market data. Based on this discovery, we introduce the generalized Gaussian distribution as the likelihood function. We further improve our method by proposing a passive variant to address transaction fees, and designing the mean function of the Gaussian process with the mean reversion principle. Thorough experiments were performed on synthetic and real-world financial datasets to verify the effectiveness of our method.
更多查看译文
关键词
Artificial intelligence in finance,bayesian learning,statistical inference
AI 理解论文
溯源树
样例
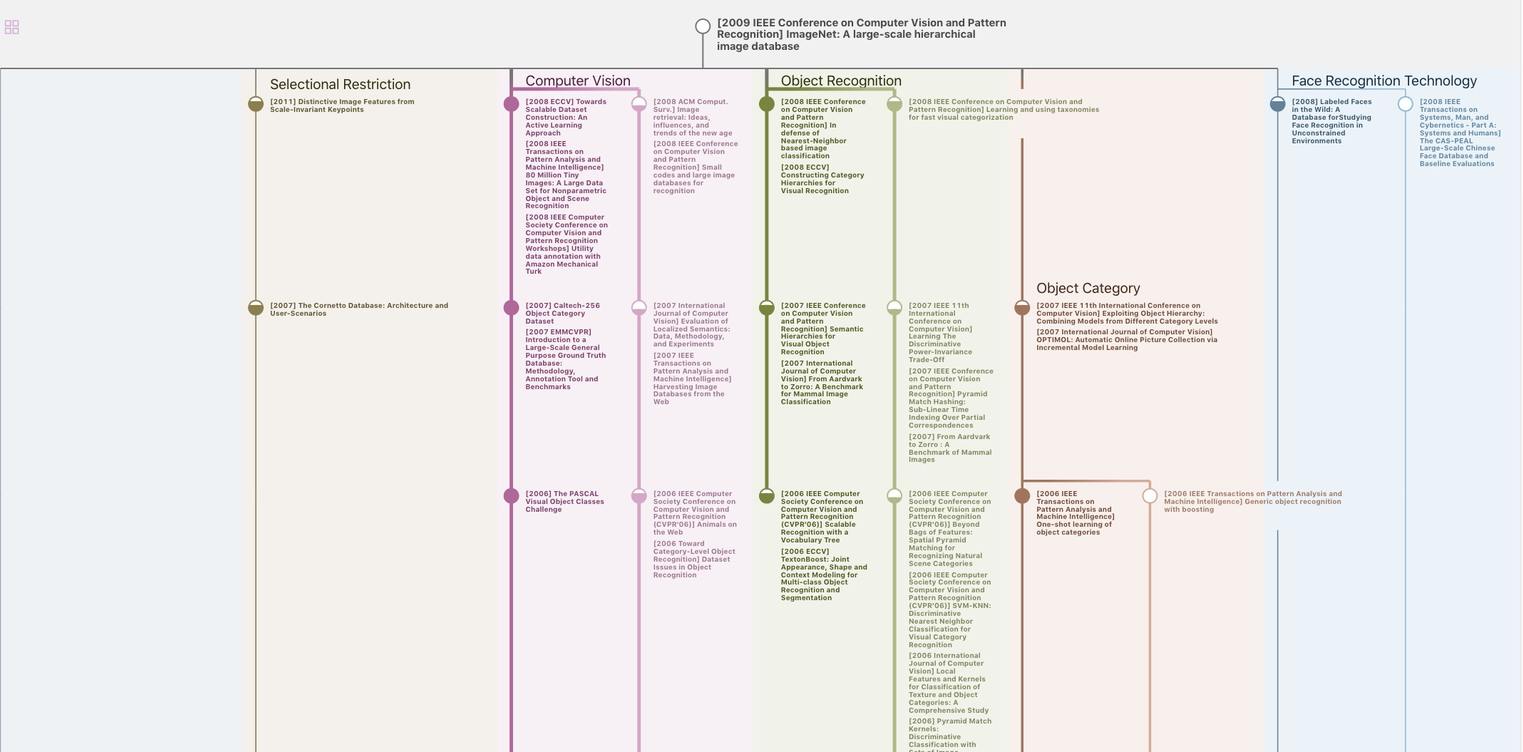
生成溯源树,研究论文发展脉络
Chat Paper
正在生成论文摘要