An Enhanced Res2Net with Local and Global Feature Fusion for Speaker Verification
CoRR(2023)
摘要
Effective fusion of multi-scale features is crucial for improving speaker verification performance. While most existing methods aggregate multi-scale features in a layer-wise manner via simple operations, such as summation or concatenation. This paper proposes a novel architecture called Enhanced Res2Net (ERes2Net), which incorporates both local and global feature fusion techniques to improve the performance. The local feature fusion (LFF) fuses the features within one single residual block to extract the local signal. The global feature fusion (GFF) takes acoustic features of different scales as input to aggregate global signal. To facilitate effective feature fusion in both LFF and GFF, an attentional feature fusion module is employed in the ERes2Net architecture, replacing summation or concatenation operations. A range of experiments conducted on the VoxCeleb datasets demonstrate the superiority of the ERes2Net in speaker verification.
更多查看译文
关键词
res2net,global feature fusion,speaker
AI 理解论文
溯源树
样例
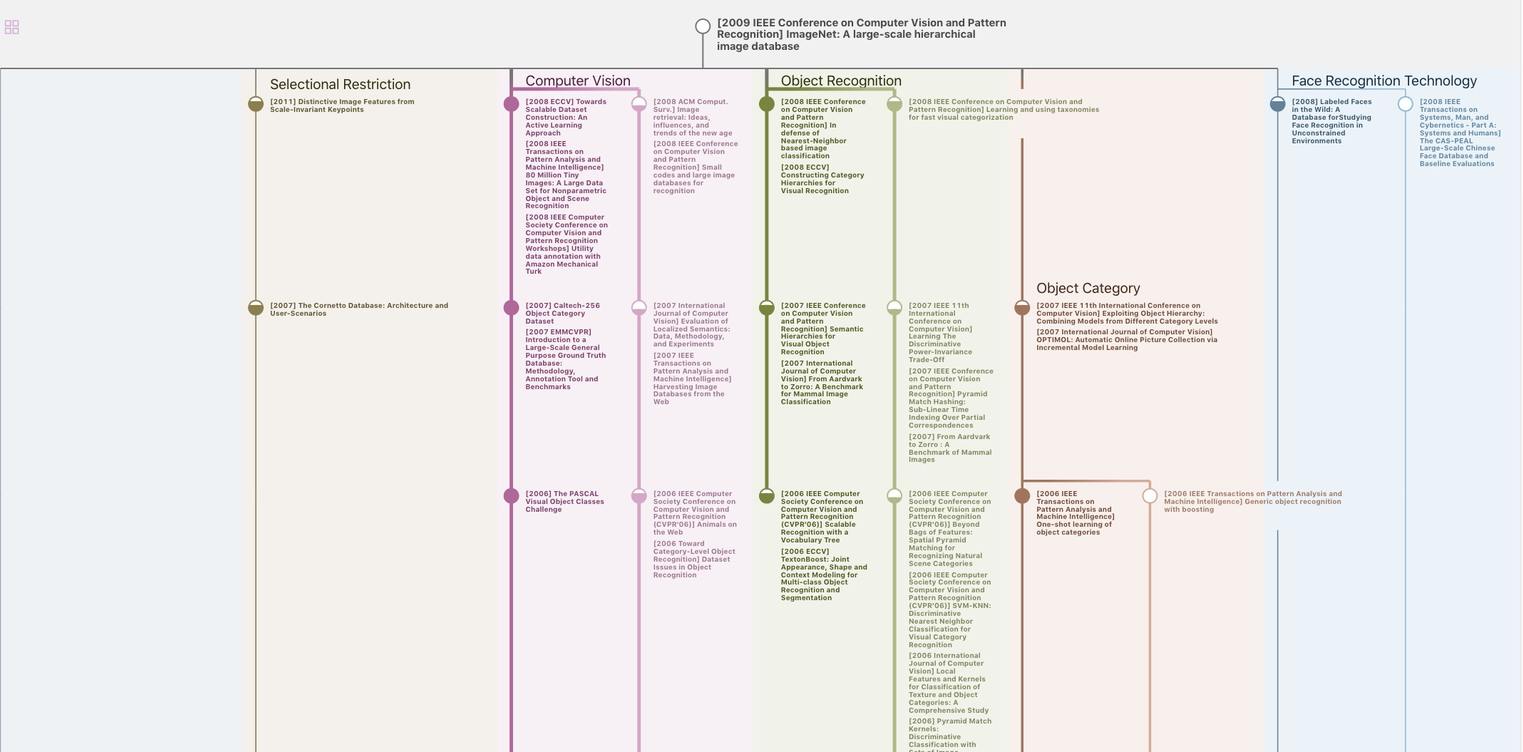
生成溯源树,研究论文发展脉络
Chat Paper
正在生成论文摘要