Single Domain Dynamic Generalization for Iris Presentation Attack Detection
CoRR(2023)
摘要
Iris presentation attack detection (PAD) has achieved great success under intra-domain settings but easily degrades on unseen domains. Conventional domain generalization methods mitigate the gap by learning domain-invariant features. However, they ignore the discriminative information in the domain-specific features. Moreover, we usually face a more realistic scenario with only one single domain available for training. To tackle the above issues, we propose a Single Domain Dynamic Generalization (SDDG) framework, which simultaneously exploits domain-invariant and domain-specific features on a per-sample basis and learns to generalize to various unseen domains with numerous natural images. Specifically, a dynamic block is designed to adaptively adjust the network with a dynamic adaptor. And an information maximization loss is further combined to increase diversity. The whole network is integrated into the meta-learning paradigm. We generate amplitude perturbed images and cover diverse domains with natural images. Therefore, the network can learn to generalize to the perturbed domains in the meta-test phase. Extensive experiments show the proposed method is effective and outperforms the state-of-the-art on LivDet-Iris 2017 dataset.
更多查看译文
关键词
Iris Presentation Attack Detection,Single Domain Generalization,Dynamic Network,Meta-Learning
AI 理解论文
溯源树
样例
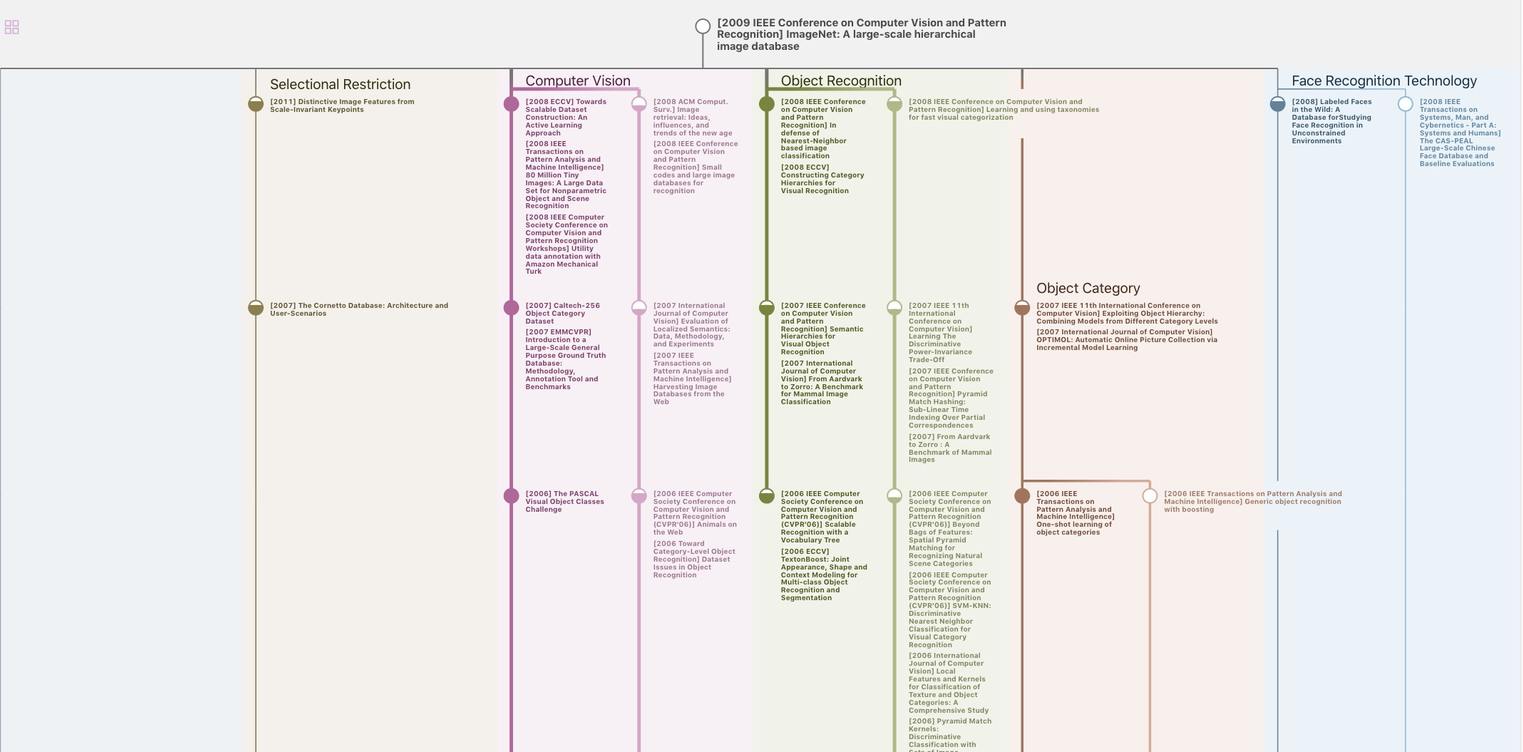
生成溯源树,研究论文发展脉络
Chat Paper
正在生成论文摘要