Interactive Data Synthesis for Systematic Vision Adaptation via LLMs-AIGCs Collaboration
CoRR(2023)
摘要
Recent text-to-image generation models have shown promising results in generating high-fidelity photo-realistic images. In parallel, the problem of data scarcity has brought a growing interest in employing AIGC technology for high-quality data expansion. However, this paradigm requires well-designed prompt engineering that cost-less data expansion and labeling remain under-explored. Inspired by LLM's powerful capability in task guidance, we propose a new paradigm of annotated data expansion named as ChatGenImage. The core idea behind it is to leverage the complementary strengths of diverse models to establish a highly effective and user-friendly pipeline for interactive data augmentation. In this work, we extensively study how LLMs communicate with AIGC model to achieve more controllable image generation and make the first attempt to collaborate them for automatic data augmentation for a variety of downstream tasks. Finally, we present fascinating results obtained from our ChatGenImage framework and demonstrate the powerful potential of our synthetic data for systematic vision adaptation. Our codes are available at https://github.com/Yuqifan1117/Labal-Anything-Pipeline.
更多查看译文
关键词
systematic vision adaptation,llms-aigcs
AI 理解论文
溯源树
样例
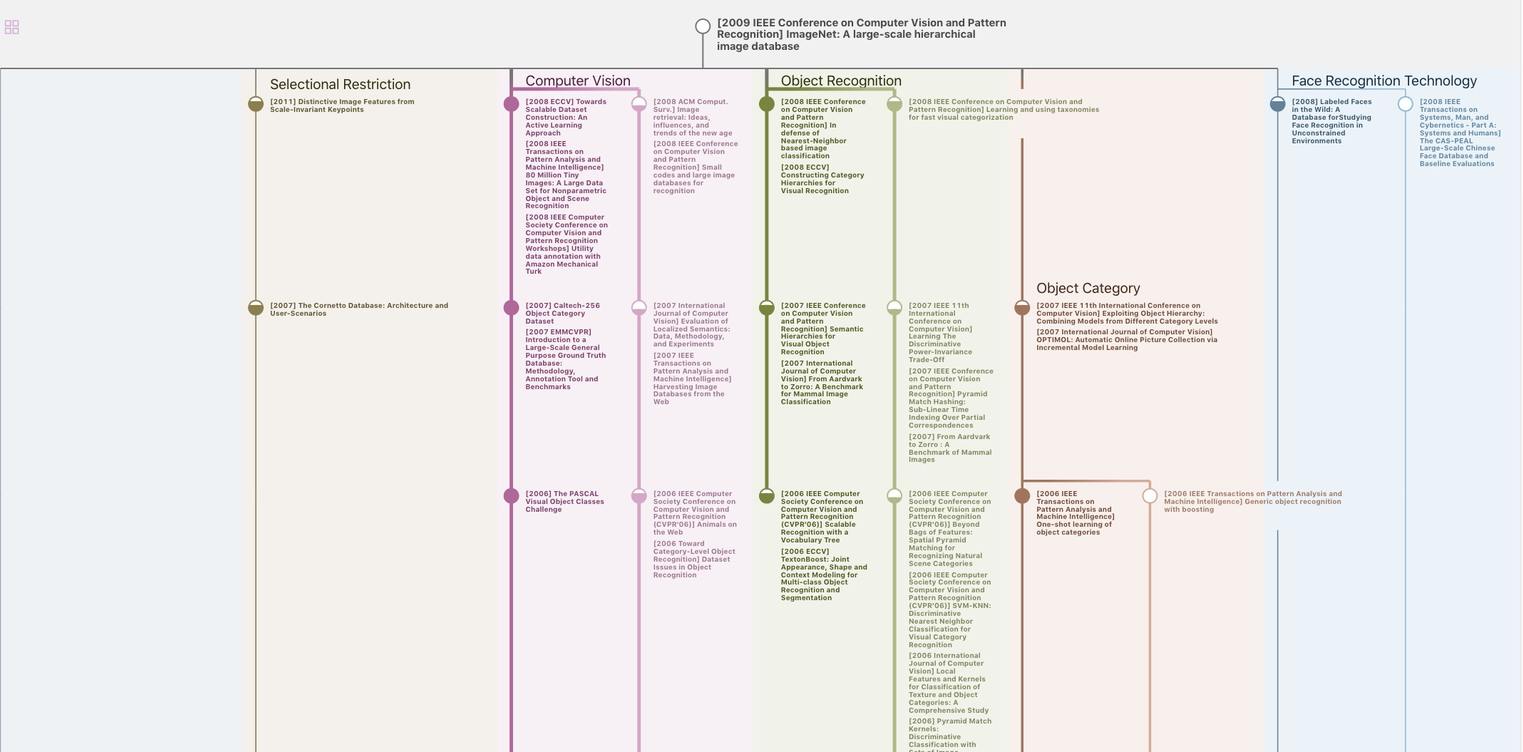
生成溯源树,研究论文发展脉络
Chat Paper
正在生成论文摘要