iWarpGAN: Disentangling Identity and Style to Generate Synthetic Iris Images
2023 IEEE International Joint Conference on Biometrics (IJCB)(2023)
摘要
Generative Adversarial Networks (GANs) have shown success in approximating complex distributions for synthetic image generation and for editing specific portions of an input image, particularly in faces. However, current GAN-based methods for generating biometric images, such as iris, have limitations in controlling the identity of the generated images, i.e., the synthetically generated images often closely resemble images in the training dataset. Further, the generated images often lack diversity in terms of the number of unique identities represented in them. To overcome these issues, we propose iWarpGAN that disentangles identity and style in the context of the iris modality by using two transformation pathways: Identity Transformation Pathway to generate unique identities from the training set, and Style Transformation Pathway to extract the style code from a reference image and output an iris image using this style. By concatenating the transformed identity code and reference style code, iWarpGAN generates iris images with both inter and intra-class variations. The efficacy of the proposed method in generating Iris DeepFakes is evaluated both qualitatively and quantitatively using ISO/IEC 29794-6 Standard Quality Metrics and the VeriEye iris matcher. Finally, the utility of the synthetically generated images is demonstrated by improving the performance of multiple deep learning based iris matchers that augment synthetic data with real data during the training process.
更多查看译文
关键词
Iris Images,Training Set,Training Dataset,Unique Identification,Generative Adversarial Networks,Image Generation,Reference Image,Quality Metrics,Synthetic Images,Intra-class Variance,Transformation Pathways,Synthetic Generation,Identity Transformation,Performance Of Deep Learning,GAN-based Methods,Poor Quality,Training Data,Input Image,Number Of Images,Pupil Size,Warp Field,Latent Space,StyleGAN,Latent Code,Source Images,Good Image,Generative Adversarial Networks Training,Image Transformation,Warping Function,Convolutional Autoencoder
AI 理解论文
溯源树
样例
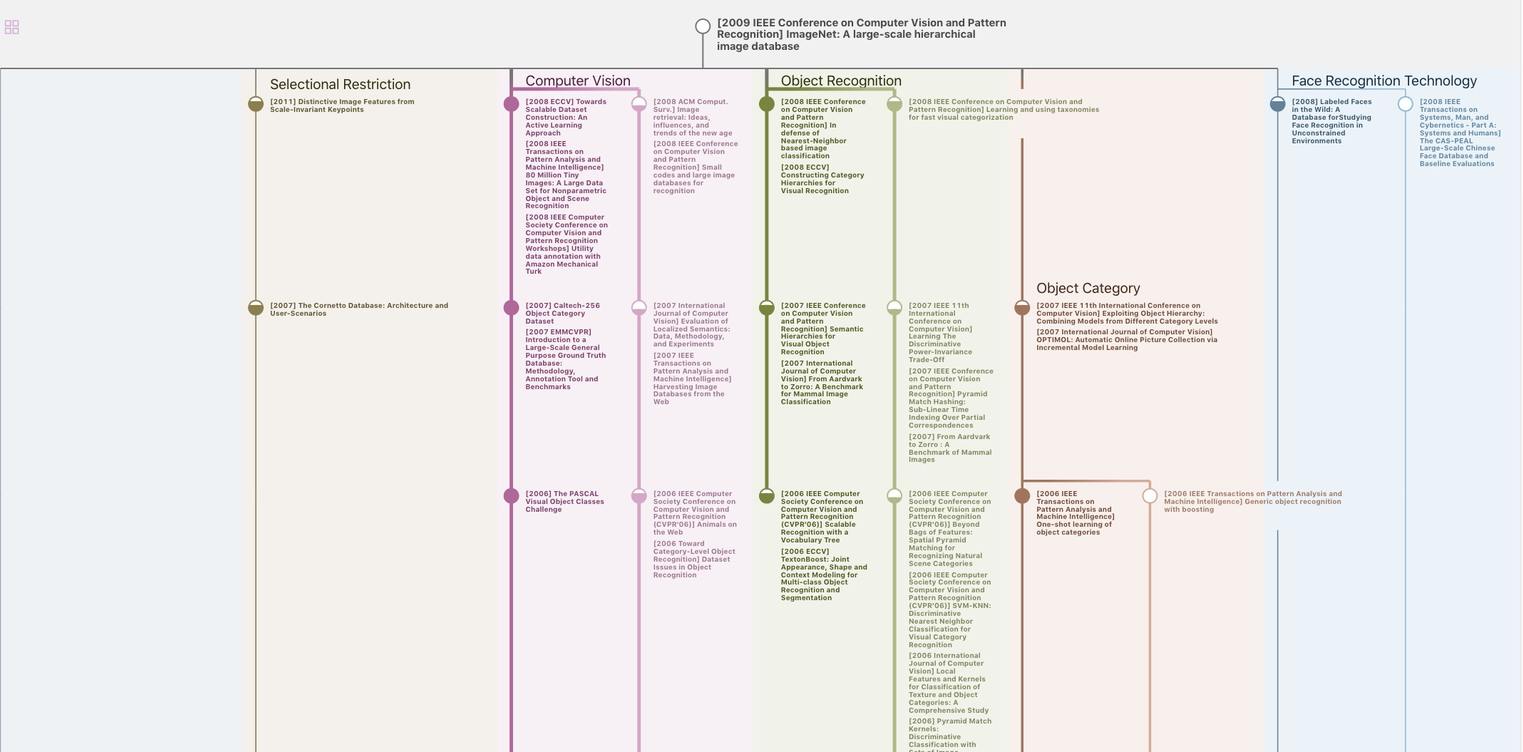
生成溯源树,研究论文发展脉络
Chat Paper
正在生成论文摘要