GeometricImageNet: Extending convolutional neural networks to vector and tensor images
CoRR(2023)
摘要
Convolutional neural networks and their ilk have been very successful for many learning tasks involving images. These methods assume that the input is a scalar image representing the intensity in each pixel, possibly in multiple channels for color images. In natural-science domains however, image-like data sets might have vectors (velocity, say), tensors (polarization, say), pseudovectors (magnetic field, say), or other geometric objects in each pixel. Treating the components of these objects as independent channels in a CNN neglects their structure entirely. Our formulation -- the GeometricImageNet -- combines a geometric generalization of convolution with outer products, tensor index contractions, and tensor index permutations to construct geometric-image functions of geometric images that use and benefit from the tensor structure. The framework permits, with a very simple adjustment, restriction to function spaces that are exactly equivariant to translations, discrete rotations, and reflections. We use representation theory to quantify the dimension of the space of equivariant polynomial functions on 2-dimensional vector images. We give partial results on the expressivity of GeometricImageNet on small images. In numerical experiments, we find that GeometricImageNet has good generalization for a small simulated physics system, even when trained with a small training set. We expect this tool will be valuable for scientific and engineering machine learning, for example in cosmology or ocean dynamics.
更多查看译文
关键词
convolutional neural networks,tensor,neural networks,images
AI 理解论文
溯源树
样例
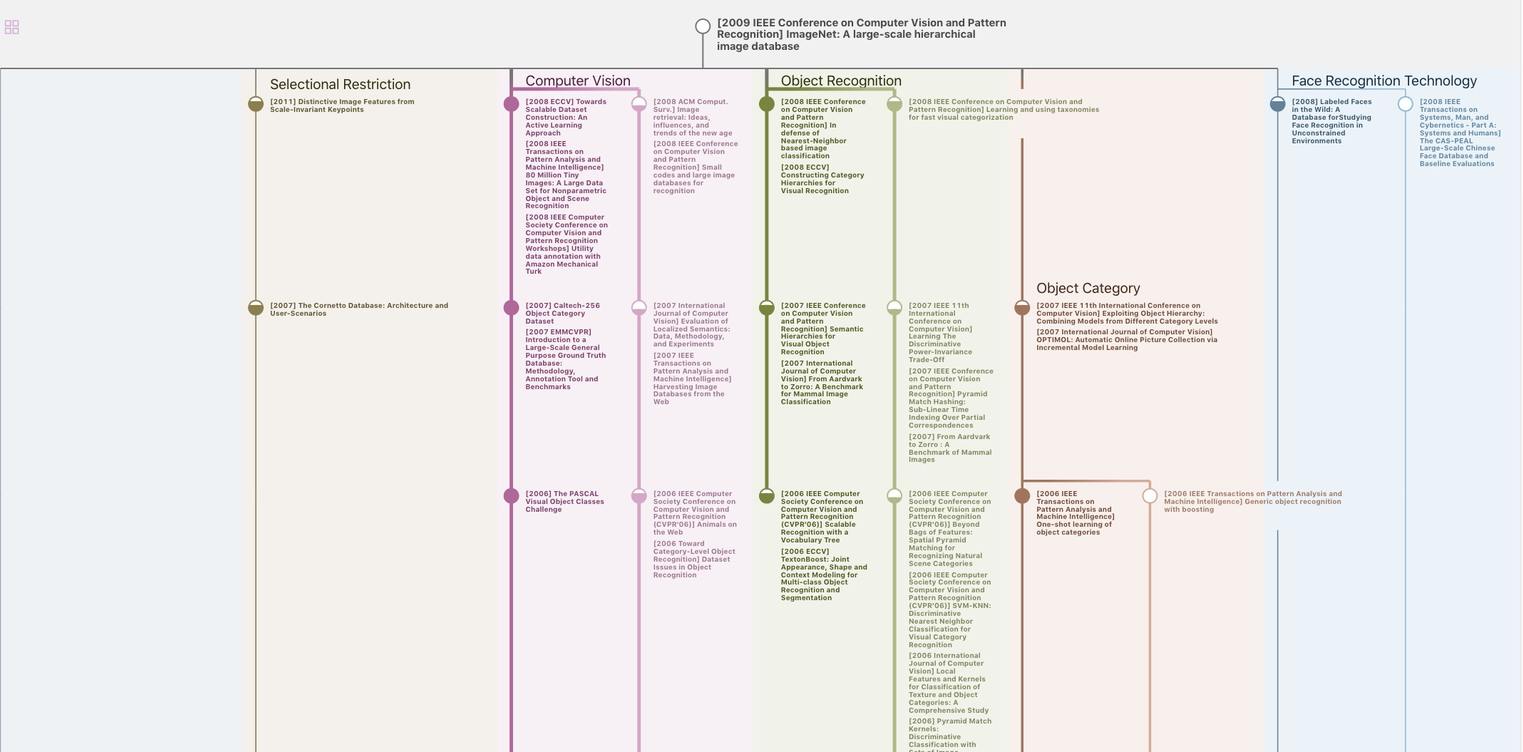
生成溯源树,研究论文发展脉络
Chat Paper
正在生成论文摘要