The Inside Story: Towards Better Understanding of Machine Translation Neural Evaluation Metrics
61ST CONFERENCE OF THE THE ASSOCIATION FOR COMPUTATIONAL LINGUISTICS, ACL 2023, VOL 2(2023)
摘要
Neural metrics for machine translation evaluation, such as COMET, exhibit significant improvements in their correlation with human judgments, as compared to traditional metrics based on lexical overlap, such as BLEU. Yet, neural metrics are, to a great extent, "black boxes" returning a single sentence-level score without transparency about the decision-making process. In this work, we develop and compare several neural explainability methods and demonstrate their effectiveness for interpreting state-of-the-art fine-tuned neural metrics. Our study reveals that these metrics leverage token-level information that can be directly attributed to translation errors, as assessed through comparison of token-level neural saliency maps with Multidimensional Quality Metrics (MQM) annotations and with synthetically-generated critical translation errors. To ease future research, we release our code at: https://github.com/Unbabel/COMET/tree/explainable-metrics.
更多查看译文
AI 理解论文
溯源树
样例
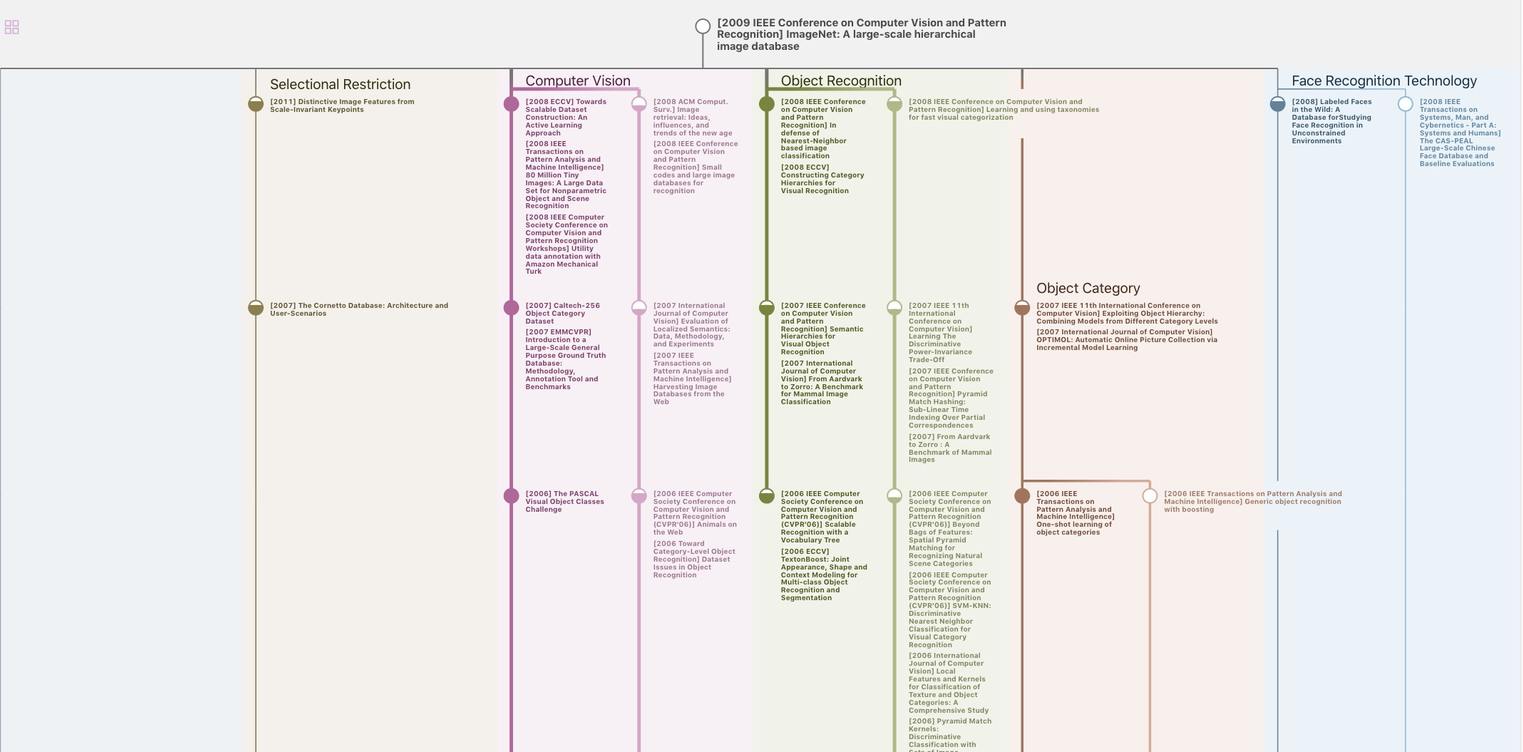
生成溯源树,研究论文发展脉络
Chat Paper
正在生成论文摘要