Fast-StrucTexT: an efficient hourglass transformer with modality-guided dynamic token merge for document understanding
CoRR(2023)
摘要
Transformers achieve promising performance in document understanding because of their high effectiveness and still suffer from quadratic computational complexity dependency on the sequence length. General efficient transformers are challenging to be directly adapted to model document. They are unable to handle the layout representation in documents, e.g. word, line and paragraph, on different granularity levels and seem hard to achieve a good trade-off between efficiency and performance. To tackle the concerns, we propose Fast-StrucTexT , an efficient multi-modal framework based on the StrucTexT algorithm with an hourglass transformer architecture, for visual document understanding. Specifically, we design a modality-guided dynamic token merging block to make the model learn multi-granularity representation and prunes redundant tokens. Additionally, we present a multi-modal interaction module called Symmetry Cross-Attention (SCA) to consider multi-modal fusion and efficiently guide the token mergence. The SCA allows one modality input as query to calculate cross attention with another modality in a dual phase. Extensive experiments on FUNSD, SROIE, and CORD datasets demonstrate that our model achieves the state-of-the-art performance and almost 1.9× faster inference time than the state-of-the-art methods.
更多查看译文
关键词
efficient hourglass transformer,dynamic token merge,fast-structext,modality-guided
AI 理解论文
溯源树
样例
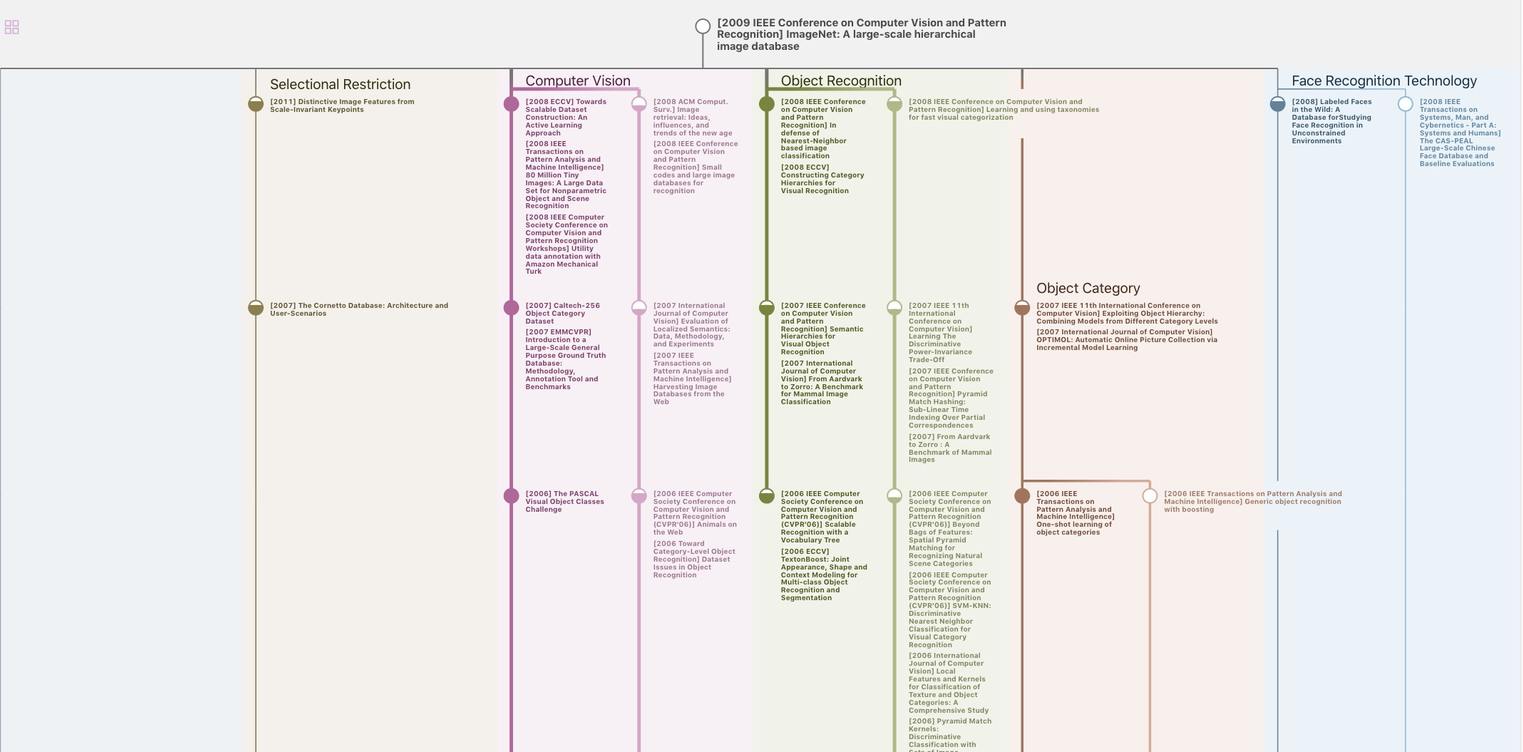
生成溯源树,研究论文发展脉络
Chat Paper
正在生成论文摘要