Neural network based prediction of the efficacy of ball milling to separate cable waste materials
Communications Engineering(2023)
摘要
Material recycling technologies are essential for achieving a circular economy while reducing greenhouse gas emissions. However, most of them remain in laboratory development. Machine learning (ML) can promote industrial application while maximising yield and environmental performance. Herein, an asynchronous-parallel recurrent neural network was developed to predict the dynamic behaviour when separating copper and poly(vinyl chloride) components from the cable waste. The model was trained with six datasets (treatment conditions) at 3600 epochs. High accuracy was confirmed based on a mean-square error of 0.0015–0.0145 between the prediction and experimental results. The quantitative relationship between the input features and the separation yield was identified using sensitivity analysis. The charged weight of cables and impact energy were determined as the critical factors affecting the separation efficiency. The ML framework can be widely applied to recycling technologies to reveal the process mechanism and establish a quantitative relationship between process variables and treatment outputs.
更多查看译文
关键词
Environmental sciences,Mechanical engineering,Engineering,general
AI 理解论文
溯源树
样例
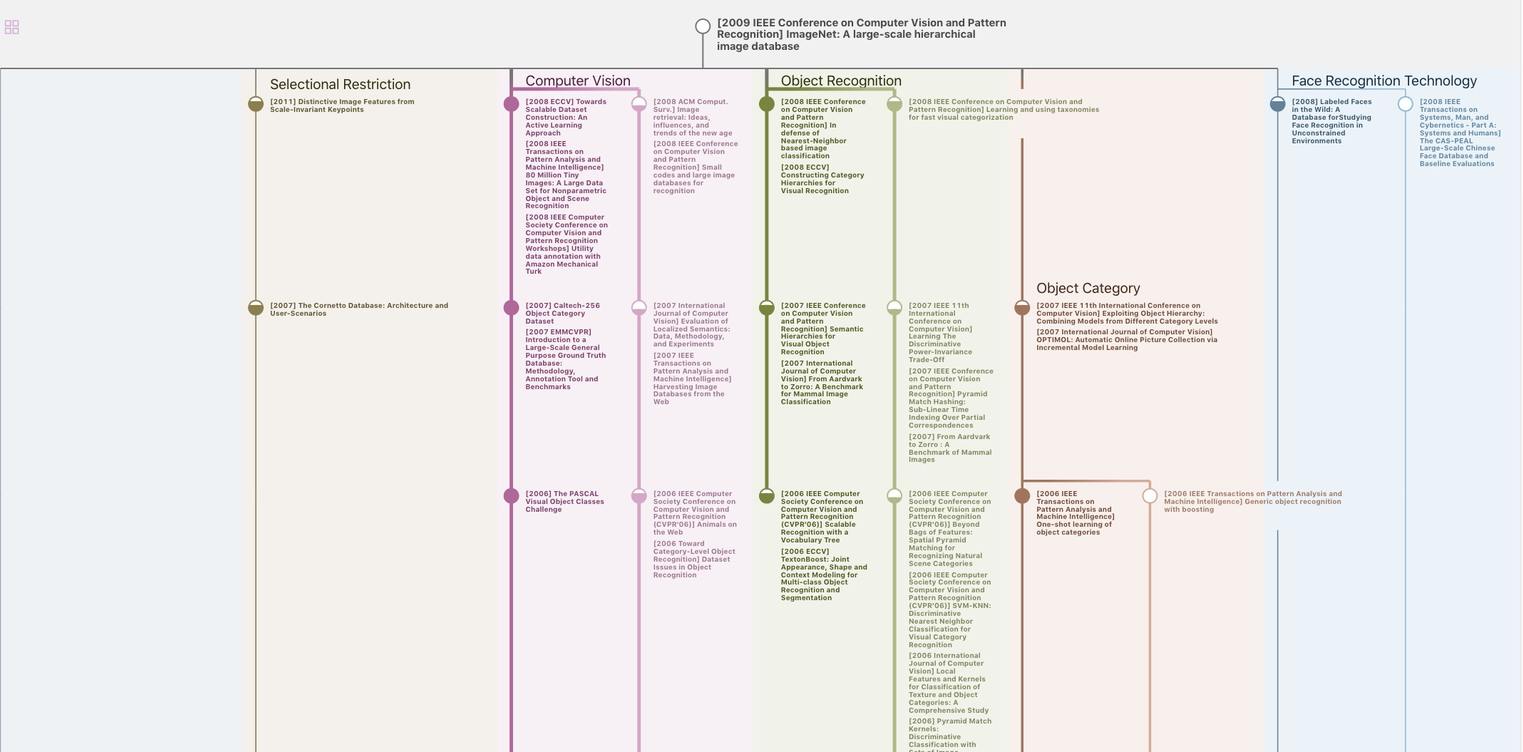
生成溯源树,研究论文发展脉络
Chat Paper
正在生成论文摘要