Semi-supervised domain adaptation in graph transfer learning
IJCAI 2023(2023)
摘要
As a specific case of graph transfer learning, unsupervised domain adaptation on graphs aims for knowledge transfer from label-rich source graphs to unlabeled target graphs. However, graphs with topology and attributes usually have considerable cross-domain disparity and there are numerous real-world scenarios where merely a subset of nodes are labeled in the source graph. This imposes critical challenges on graph transfer learning due to serious domain shifts and label scarcity. To address these challenges, we propose a method named Semi-supervised Graph Domain Adaptation (SGDA). To deal with the domain shift, we add adaptive shift parameters to each of the source nodes, which are trained in an adversarial manner to align the cross-domain distributions of node embedding, thus the node classifier trained on labeled source nodes can be transferred to the target nodes. Moreover, to address the label scarcity, we propose pseudo-labeling on unlabeled nodes, which improves classification on the target graph via measuring the posterior influence of nodes based on their relative position to the class centroids. Finally, extensive experiments on a range of publicly accessible datasets validate the effectiveness of our proposed SGDA in different experimental settings.
更多查看译文
关键词
graph transfer learning,domain adaptation,semi-supervised
AI 理解论文
溯源树
样例
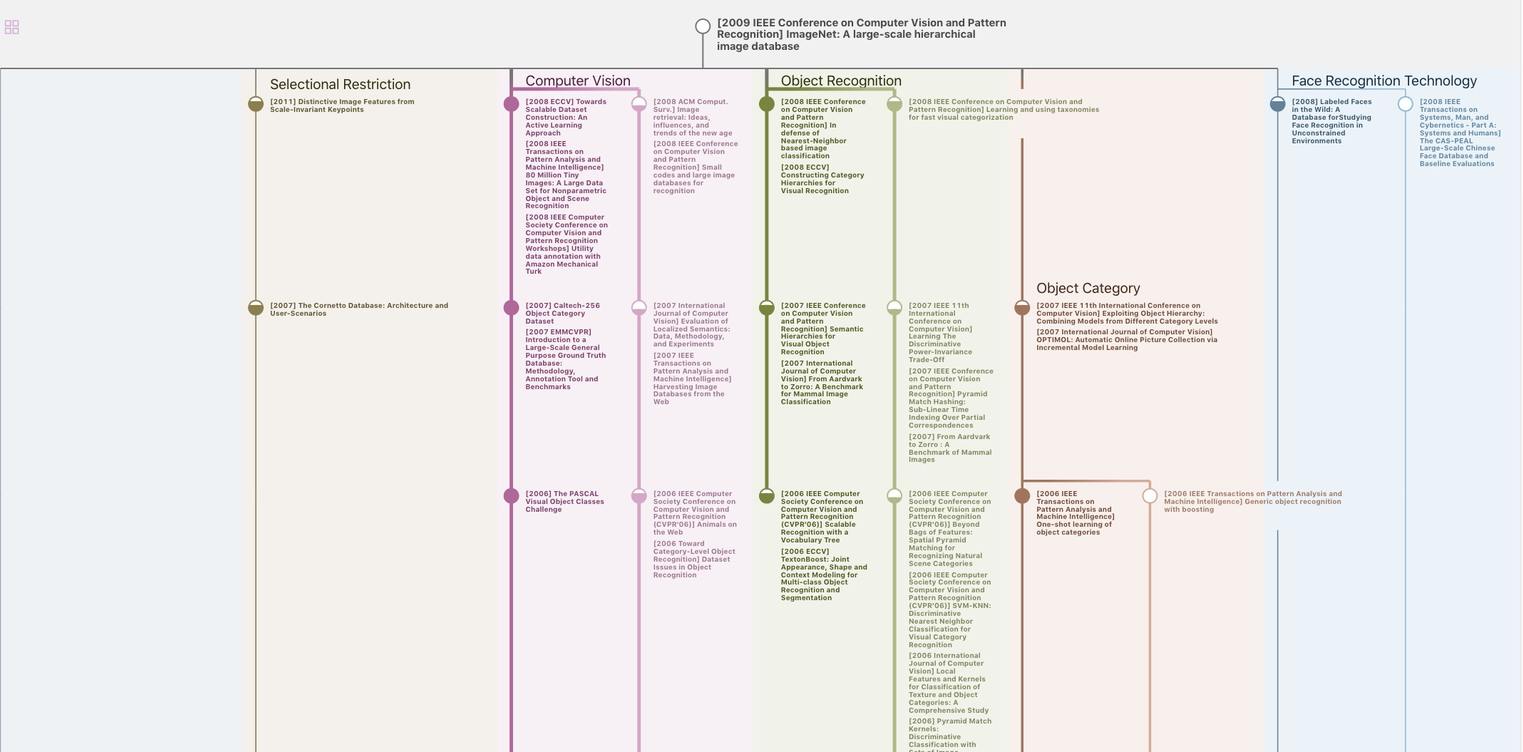
生成溯源树,研究论文发展脉络
Chat Paper
正在生成论文摘要