Contrastive State Augmentations for Reinforcement Learning-Based Recommender Systems
PROCEEDINGS OF THE 46TH INTERNATIONAL ACM SIGIR CONFERENCE ON RESEARCH AND DEVELOPMENT IN INFORMATION RETRIEVAL, SIGIR 2023(2023)
摘要
Learning reinforcement learning (RL)-based recommenders from historical user-item interaction sequences is vital to generate high-reward recommendations and improve long-term cumulative benefits. However, existing RL recommendation methods encounter difficulties (i) to estimate the value functions for states which are not contained in the offline training data, and (ii) to learn effective state representations from user implicit feedback due to the lack of contrastive signals. In this work, we propose contrastive state augmentations (CSA) for the training of RL-based recommender systems. To tackle the first issue, we propose four state augmentation strategies to enlarge the state space of the offline data. The proposed method improves the generalization capability of the recommender by making the RL agent visit the local state regions and ensuring the learned value functions are similar between the original and augmented states. For the second issue, we propose introducing contrastive signals between augmented states and the state randomly sampled from other sessions to improve the state representation learning further. To verify the effectiveness of the proposed CSA, we conduct extensive experiments on two publicly accessible datasets and one dataset collected from a real-life e-commerce platform. We also conduct experiments on a simulated environment as the online evaluation setting. Experimental results demonstrate that CSA can effectively improve recommendation performance.
更多查看译文
关键词
Recommender system,Reinforcement learning,Contrastive learning,Data augmentation,Sequential recommendation
AI 理解论文
溯源树
样例
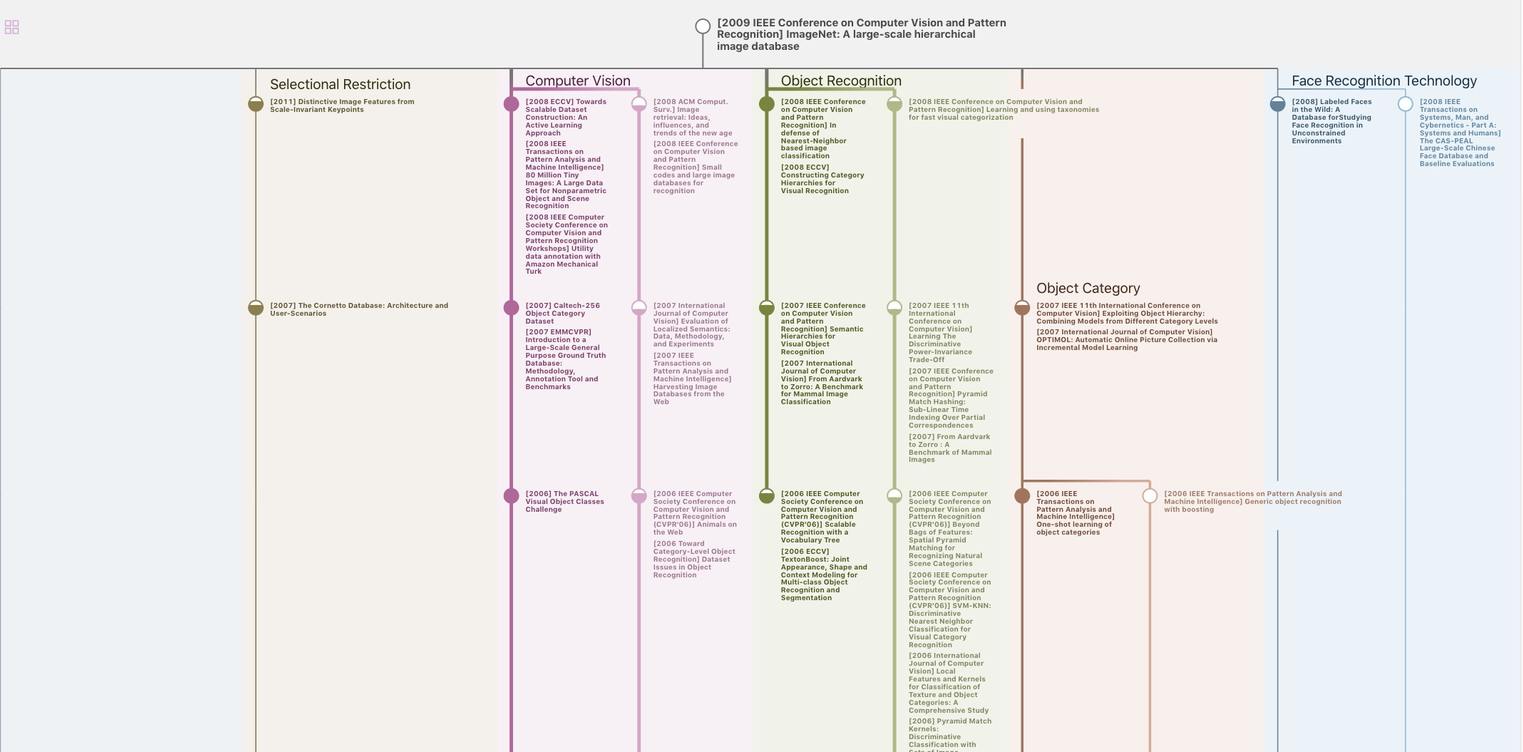
生成溯源树,研究论文发展脉络
Chat Paper
正在生成论文摘要