Q-SHED: Distributed Optimization at the Edge via Hessian Eigenvectors Quantization
ICC 2023 - IEEE INTERNATIONAL CONFERENCE ON COMMUNICATIONS(2023)
摘要
Edge networks call for communication efficient (low overhead) and robust distributed optimization (DO) algorithms. These are, in fact, desirable qualities for DO frameworks, such as federated edge learning techniques, in the presence of data and system heterogeneity, and in scenarios where inter-node communication is the main bottleneck. Although computationally demanding, Newton-type (NT) methods have been recently advocated as enablers of robust convergence rates in challenging DO problems where edge devices have sufficient computational power. Along these lines, in this work we propose Q-SHED, an original NT algorithm for DO featuring a novel bit-allocation scheme based on incremental Hessian eigenvectors quantization. The proposed technique is integrated with the recent SHED algorithm, from which it inherits appealing features like the small number of required Hessian computations, while being bandwidth-versatile at a bit-resolution level. Our empirical evaluation against competing approaches shows that Q-SHED can reduce by up to 60% the number of communication rounds required for convergence.
更多查看译文
关键词
Newton method,distributed optimization,federated edge learning,wireless networks,6G
AI 理解论文
溯源树
样例
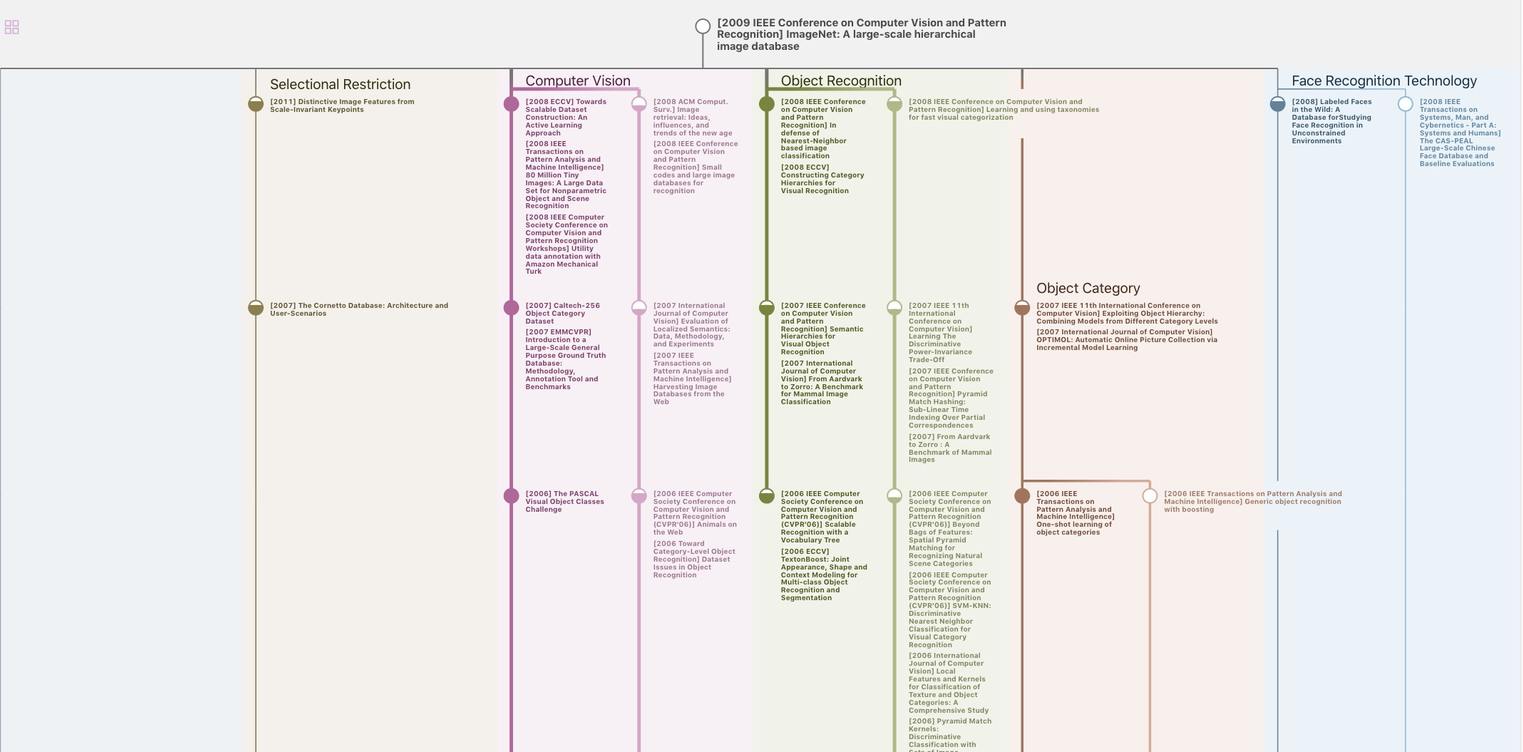
生成溯源树,研究论文发展脉络
Chat Paper
正在生成论文摘要