Jet Diffusion versus JetGPT -- Modern Networks for the LHC
arXiv (Cornell University)(2023)
摘要
We introduce two diffusion models and an autoregressive transformer for LHC physics simulations. Bayesian versions allow us to control the networks and capture training uncertainties. After illustrating their different density estimation methods for simple toy models, we discuss their advantages for Z plus jets event generation. While diffusion networks excel through their precision, the transformer scales best with the phase space dimensionality. Given the different training and evaluation speed, we expect LHC physics to benefit from dedicated use cases for normalizing flows, diffusion models, and autoregressive transformers.
更多查看译文
关键词
jetgpt,lhc,modern networks,diffusion
AI 理解论文
溯源树
样例
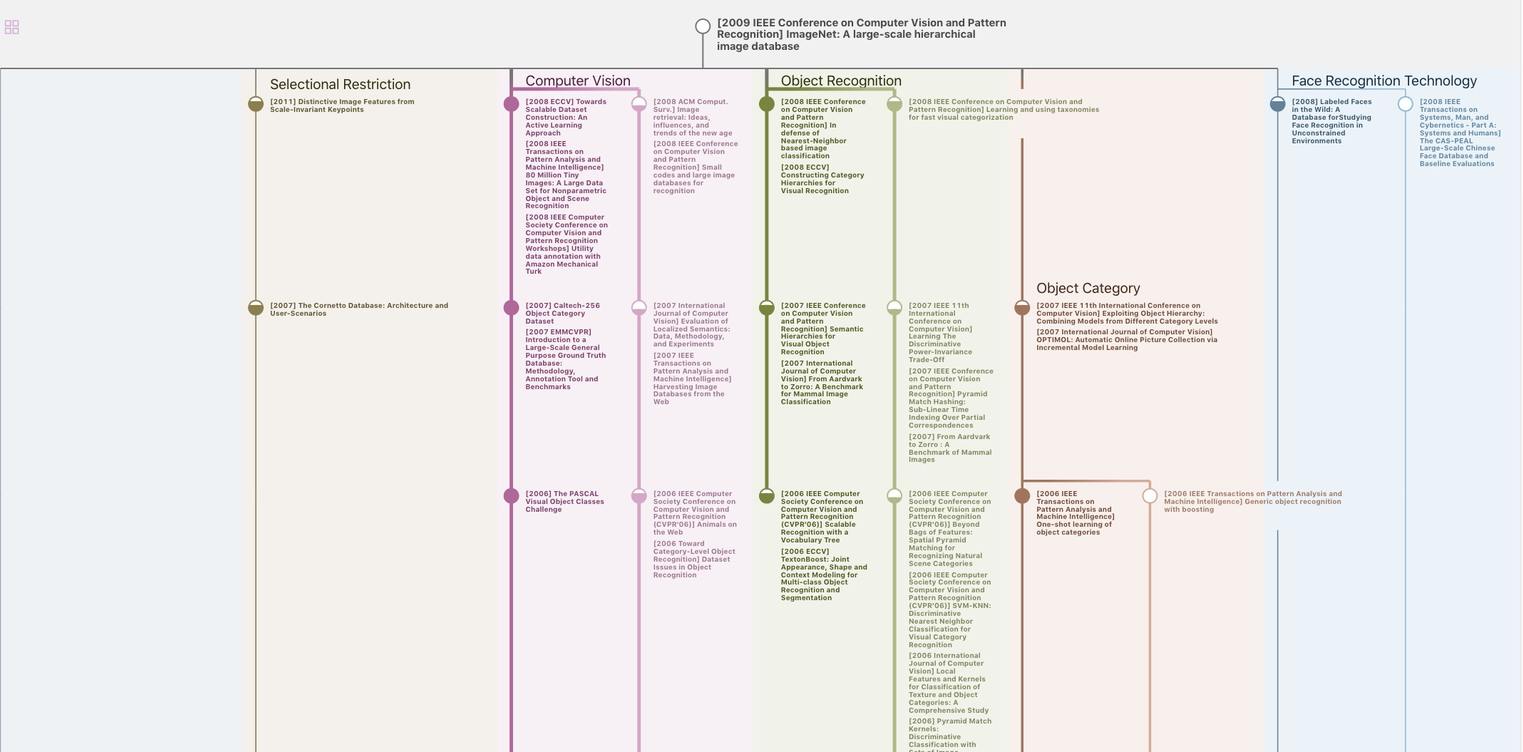
生成溯源树,研究论文发展脉络
Chat Paper
正在生成论文摘要