On the Remarkable Efficiency of SMART
Scale Space and Variational Methods in Computer Vision(2023)
摘要
We consider the problem of minimizing the Kullback-Leibler divergence between two unnormalised positive measures, where the first measure lies in a finitely generated convex cone. We identify SMART (simultaneous multiplicative algebraic reconstruction technique) as a Riemannian gradient descent on the parameter manifold of the Poisson distribution. By comparing SMART to recent acceleration techniques from convex optimization that rely on Bregman geometry and first-order information, we demonstrate that it solves this problem very efficiently.
更多查看译文
关键词
KL divergence, accelerated mirror descent, relative smoothness, information geometry, Riemannian gradient descent
AI 理解论文
溯源树
样例
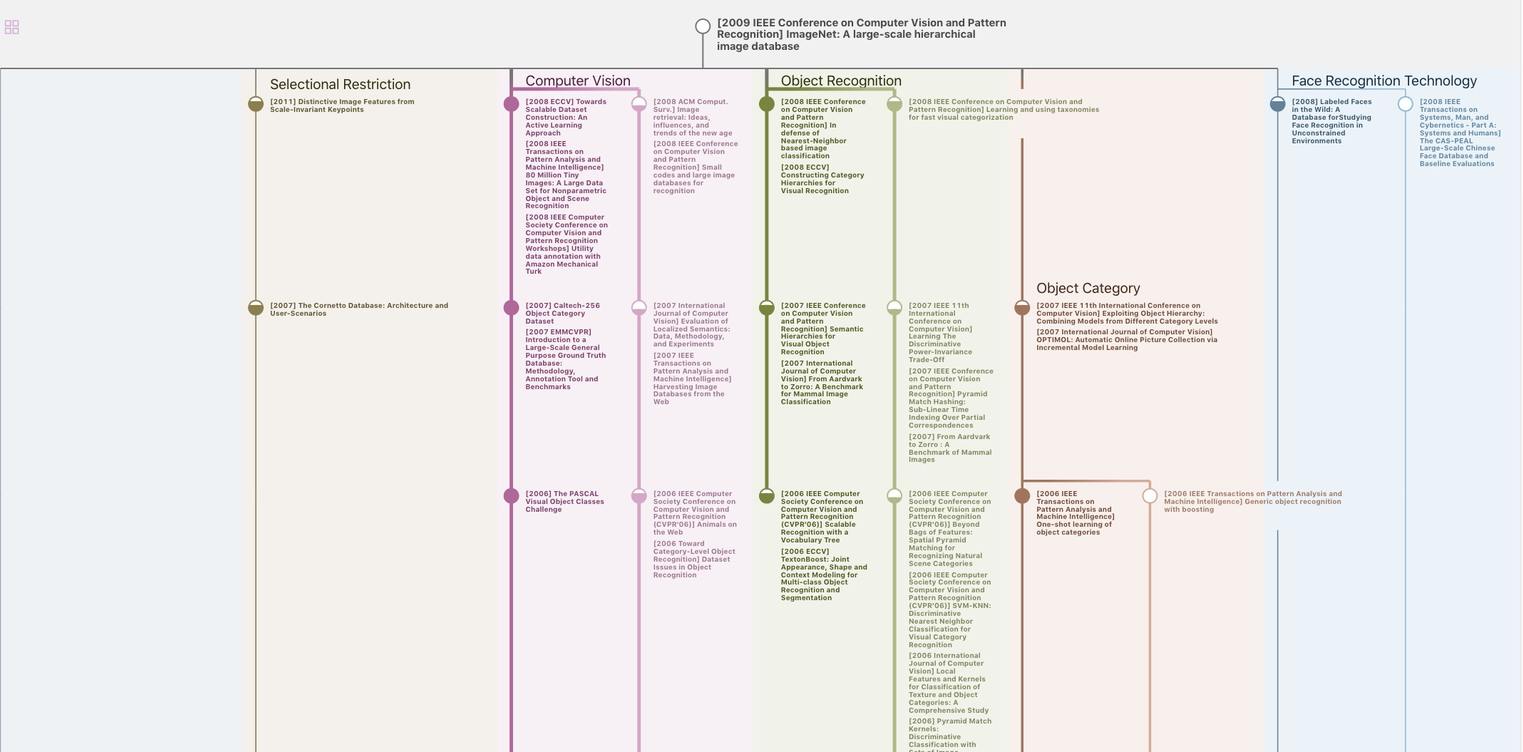
生成溯源树,研究论文发展脉络
Chat Paper
正在生成论文摘要