Utilizing Transformers on OCT Imagery and Metadata for Treatment Response Prediction in Macular Edema Patients
Image Analysis(2023)
摘要
Macular edema is a type of eye disease commonly associated with diabetes and treated with three injections namely Ranibizumab, Aflibercept, and Bevacizumab. Often, the prescription is majorly dependent on the decision of a physician supported by optical coherence tomography (OCT) images that capture the progress or failure of treatments. Nevertheless, comparative clinical effectiveness of these treatments for the management of macular edema remains unclear. Additionally, each of these treatments are driven by cost-to-patient, as well as medical side effects. Thus, there is a high demand for artificial intelligence (AI)-driven decision assistance to avoid ineffective treatments and unnecessary costs. Therefore, this work aims at proposing a machine learning (ML)-based decision support system for physicians. The results illustrate that the transformer-based model is capable of predicting if the respective treatment will have a positive, or negative impact for the patient with an accuracy higher than a skillful physician (acc of 89.07). In addition, we have shown that the combination of metadata from patient clinical records with OCT images shows 12% increase in accuracy as compared to the models trained only using OCT images. In conclusion, the proposed ML model provides comparative effectiveness probabilities for the three types of injections which can assist physicians in macular edema treatment after conducting proper follow-up and clinical trial studies.
更多查看译文
关键词
Medical Decision Support System, Artificial Intelligence, Transformer, Multi-head Attention Mechanism, Neural Network
AI 理解论文
溯源树
样例
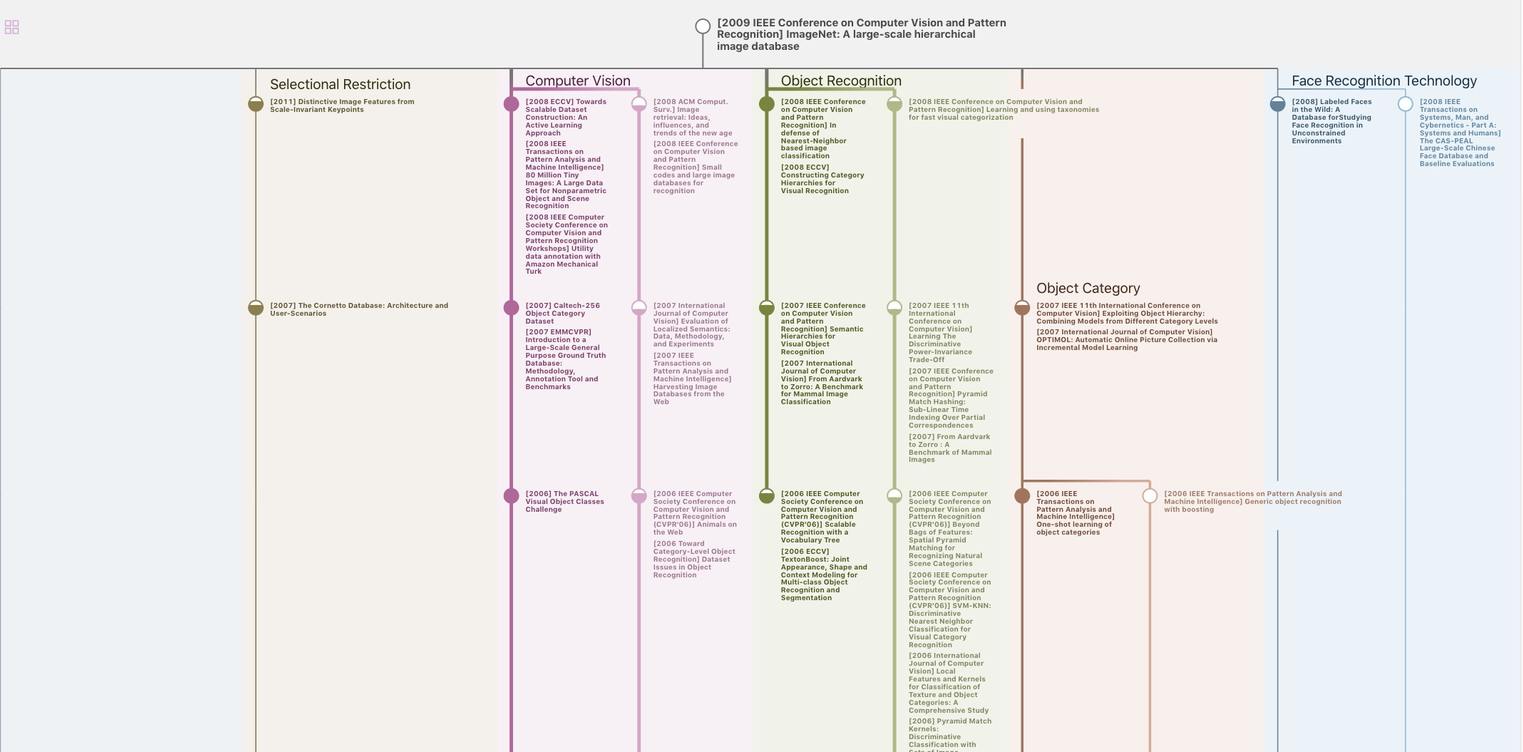
生成溯源树,研究论文发展脉络
Chat Paper
正在生成论文摘要