Density Peaks Clustering Algorithm for Manifold Data Based on Geodesic Distance and Weighted Nearest Neighbor Similarity
Advances in Smart Vehicular Technology, Transportation, Communication and Applications(2023)
摘要
The common forms of manifold data are curvilinear, spiral, and circular. Density peak clustering algorithm (DPC) is difficult to find the cluster center accurately when facing manifold dataset, and it is easy to assign the samples belonging to the same cluster to other cluster centers which are closer to it, resulting in poor clustering accuracy. To this end, Density Peak Clustering Algorithm for Manifold Data Based on Geodesic Distance and Weighted Nearest Neighbor Similarity (DPC-GWNN) is provided in this paper. The DPC-GWNN algorithm firstly combines the weighted K-nearest neighbors to redefine the local density in order to find the clustering center more accurately; secondly, the geodesic distance is used to calculate the sample spacing instead of the Euclidean distance, so that the samples on the same manifold cluster are closer to each other and the samples between different manifold clusters are farther apart; finally, the shared nearest neighbor Finally, the new inter-sample similarity is defined using the shared nearest neighbor and the natural nearest neighbor weighted similarity to fully integrate the local information of samples and avoid the cascading effect of errors, thus achieving a better clustering effect. In this paper, we compare the DPC-GWNN algorithm with other algorithms and find that the DPC-GWNN algorithm can accurately identify the manifold class clusters and find the cluster center to achieve accurate clustering.
更多查看译文
关键词
manifold data,weighted nearest neighbor similarity,geodesic distance,algorithm
AI 理解论文
溯源树
样例
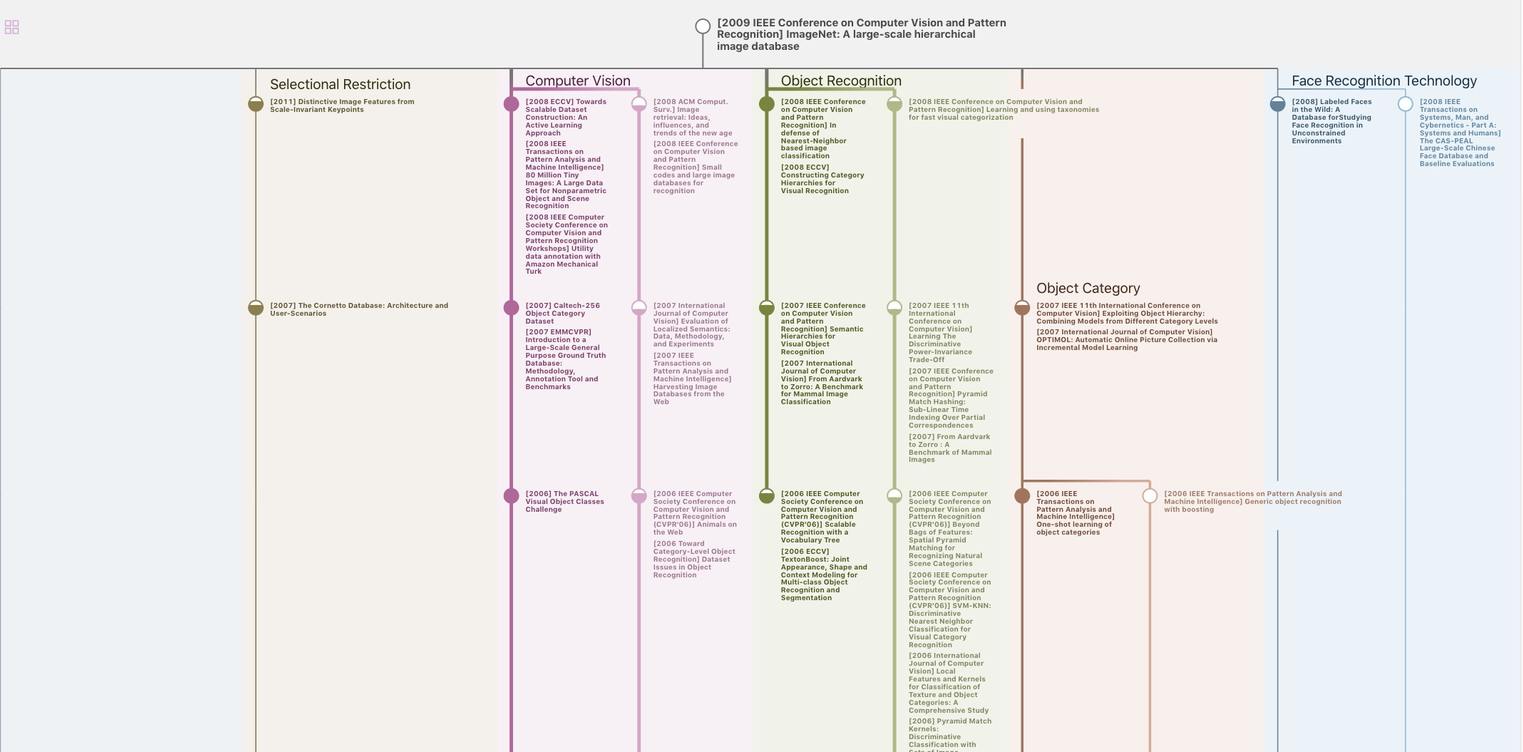
生成溯源树,研究论文发展脉络
Chat Paper
正在生成论文摘要