Discovering Diverse Top-K Characteristic Lists
Advances in Intelligent Data Analysis XXI(2023)
摘要
In this work, we define the new problem of finding diverse top-k characteristic lists to provide different statistically robust explanations of the same dataset. This type of problem is often encountered in complex domains, such as medicine, in which a single model cannot consistently explain the already established ground truth, needing a diversity of models. We propose a solution for this new problem based on Subgroup Discovery (SD). Moreover, the diversity is described in terms of coverage and descriptions. The characteristic lists are obtained using an extension of SD, in which a subgroup identifies a set of relations between attributes (description) with respect to an attribute of interest (target). In particular, the generation of these characteristic lists is driven by the Minimum Description Length (MDL) principle, which is based on the idea that the best explanation of the data is the one that achieves the greatest compression. Finally, we also propose an algorithm called GMSL which is simple and easy to interpret and obtains a collection of diverse top-k characteristic lists.
更多查看译文
关键词
Subgroup Discovery, Subgroup List, the Minimum Description Length principle, Algorithm, Interpretable Machine Learning
AI 理解论文
溯源树
样例
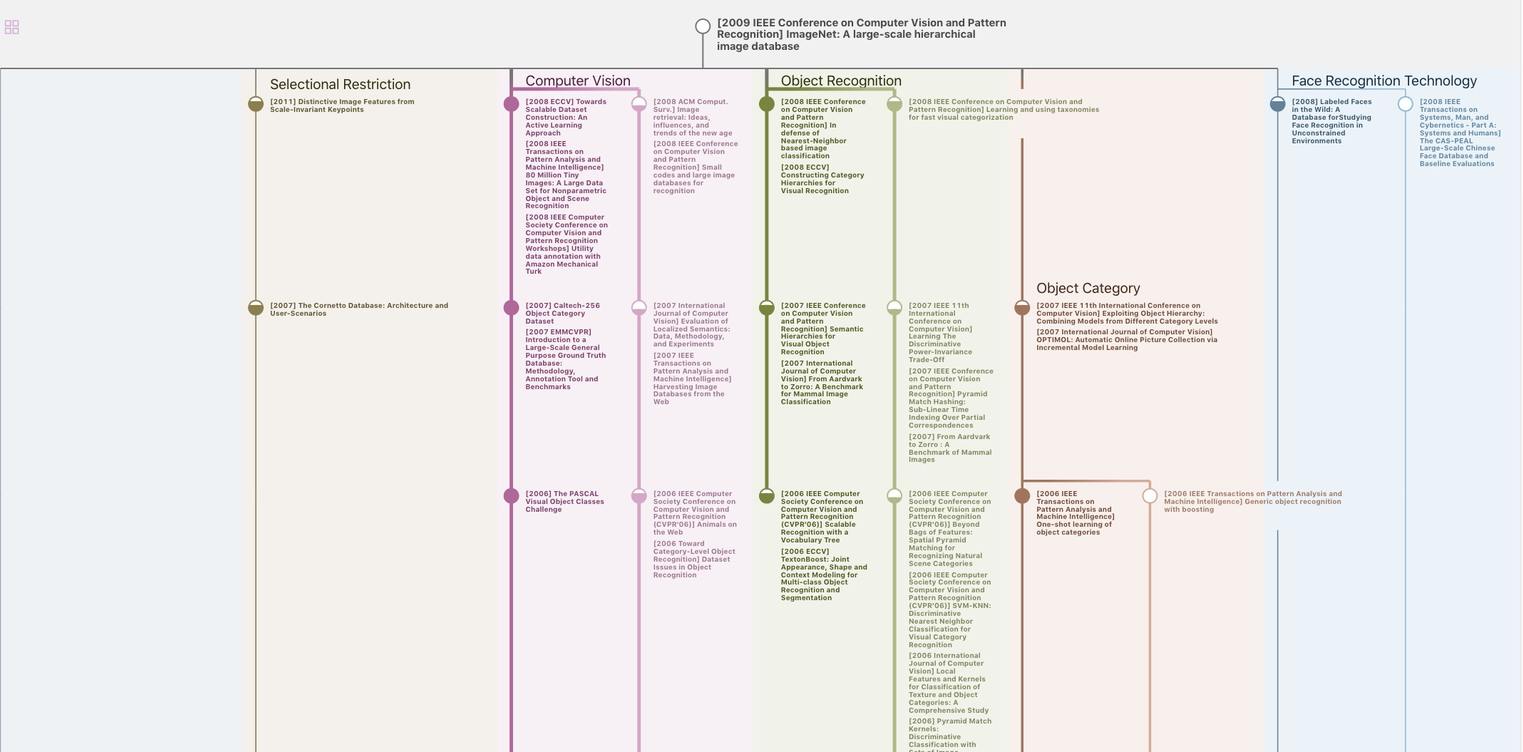
生成溯源树,研究论文发展脉络
Chat Paper
正在生成论文摘要