What matters in reinforcement learning for tractography
MEDICAL IMAGE ANALYSIS(2024)
摘要
Recently, deep reinforcement learning (RL) has been proposed to learn the tractography procedure and train agents to reconstruct the structure of the white matter without manually curated reference streamlines. While the performances reported were competitive, the proposed framework is complex, and little is still known about the role and impact of its multiple parts. In this work, we thoroughly explore the different components of the proposed framework, such as the choice of the RL algorithm, seeding strategy, the input signal and reward function, and shed light on their impact. Approximately 7,400 models were trained for this work, totalling nearly 41,000 h of GPU time. Our goal is to guide researchers eager to explore the possibilities of deep RL for tractography by exposing what works and what does not work with the category of approach. As such, we ultimately propose a series of recommendations concerning the choice of RL algorithm, the input to the agents, the reward function and more to help future work using reinforcement learning for tractography. We also release the open source codebase, trained models, and datasets for users and researchers wanting to explore reinforcement learning for tractography.
更多查看译文
关键词
Tractography,Tractography Reinforcement learning,Machine learning
AI 理解论文
溯源树
样例
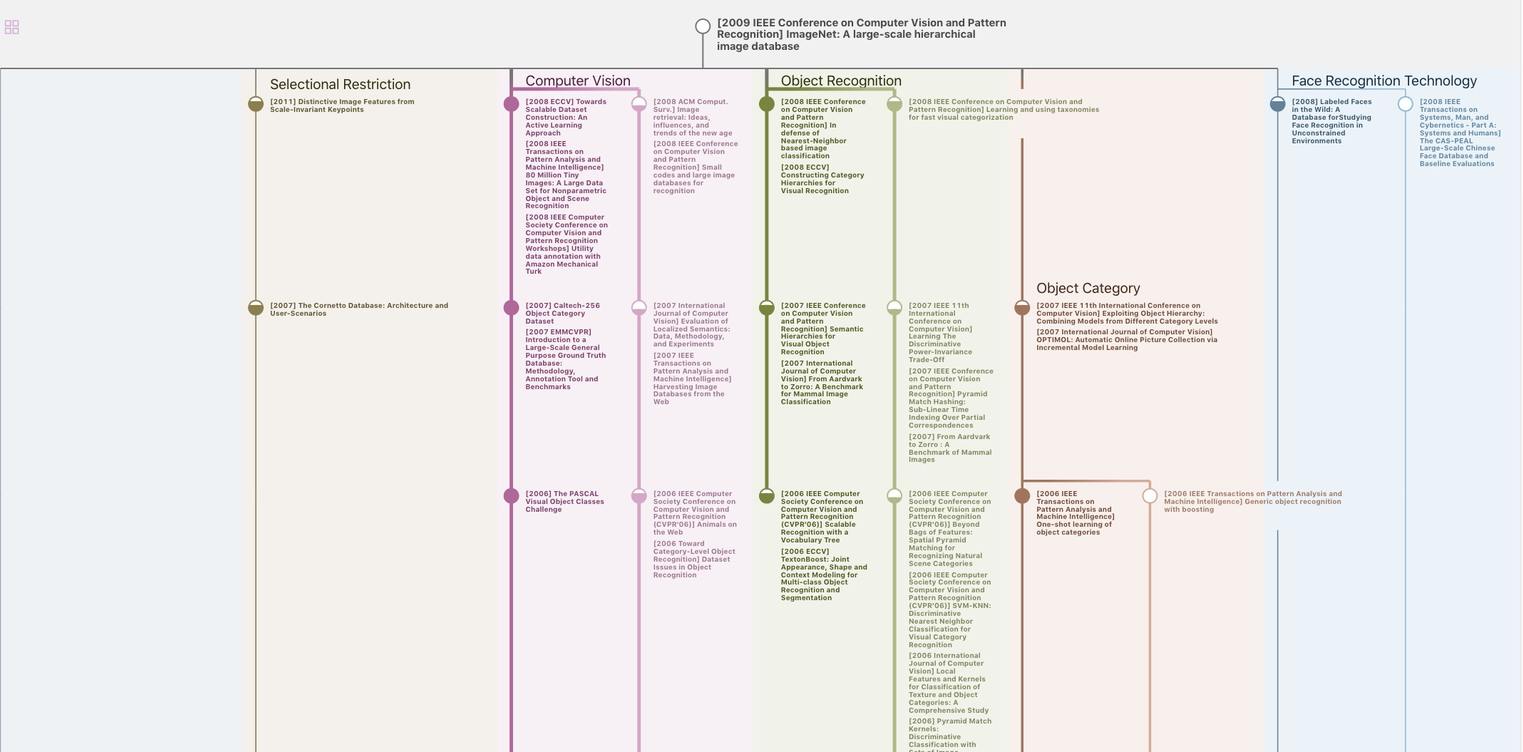
生成溯源树,研究论文发展脉络
Chat Paper
正在生成论文摘要