Triple-kernel gated attention-based multiple instance learning with contrastive learning for medical image analysis
Applied Intelligence(2023)
摘要
In machine learning, multiple instance learning is a method evolved from supervised learning algorithms, which defines a “bag” as a collection of multiple examples with a wide range of applications. In this paper, we propose a novel deep multiple instance learning model for medical image analysis, called triple-kernel gated attention-based multiple instance learning with contrastive learning. It can be used to overcome the limitations of the existing multiple instance learning approaches to medical image analysis. Our model consists of four steps. i) Extracting the representations by a simple convolutional neural network using contrastive learning for training. ii) Using three different kernel functions to obtain the importance of each instance from the entire image and forming an attention map. iii) Based on the attention map, aggregating the entire image together by attention-based MIL pooling. iv) Feeding the results into the classifier for prediction. The results on different datasets demonstrate that the proposed model outperforms state-of-the-art methods on binary and weakly supervised classification tasks. It can provide more efficient classification results for various disease models and additional explanatory information.
更多查看译文
关键词
Deep learning,Multiple instance learning,Medical image analysis
AI 理解论文
溯源树
样例
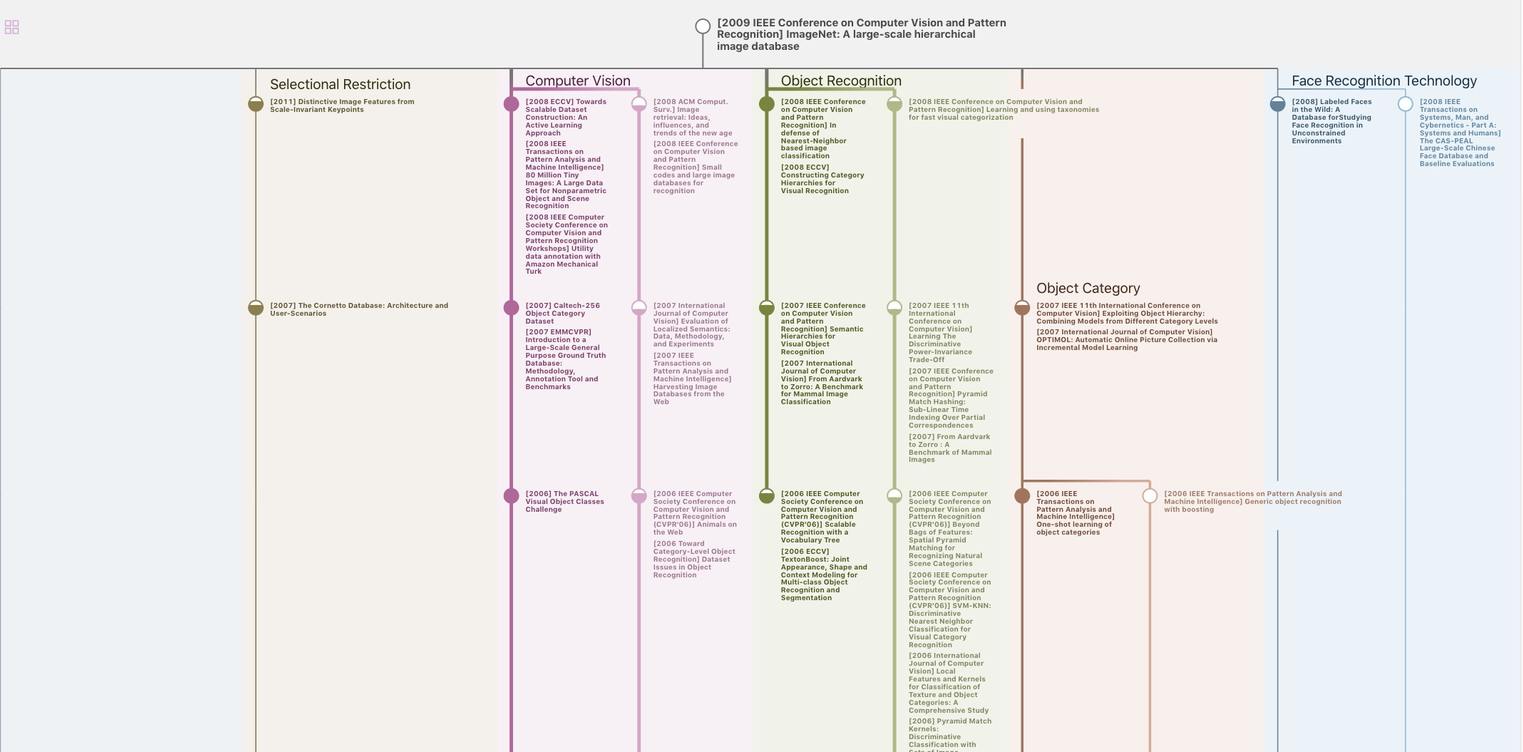
生成溯源树,研究论文发展脉络
Chat Paper
正在生成论文摘要