How to Train Your CheXDragon: Training Chest X-Ray Models for Transfer to Novel Tasks and Healthcare Systems
CoRR(2023)
摘要
Self-supervised learning (SSL) enables label efficient training for machine learning models. This is essential for domains such as medical imaging, where labels are costly and time-consuming to curate. However, the most effective supervised or SSL strategy for transferring models to different healthcare systems or novel tasks is not well understood. In this work, we systematically experiment with a variety of supervised and self-supervised pretraining strategies using multimodal datasets of medical images (chest X-rays) and text (radiology reports). We then evaluate their performance on data from two external institutions with diverse sets of tasks. In addition, we experiment with different transfer learning strategies to effectively adapt these pretrained models to new tasks and healthcare systems. Our empirical results suggest that multimodal SSL gives substantial gains over unimodal SSL in performance across new healthcare systems and tasks, comparable to models pretrained with full supervision. We demonstrate additional performance gains with models further adapted to the new dataset and task, using multimodal domain-adaptive pretraining (DAPT), linear probing then finetuning (LP-FT), and both methods combined. We offer suggestions for alternative models to use in scenarios where not all of these additions are feasible. Our results provide guidance for improving the generalization of medical image interpretation models to new healthcare systems and novel tasks.
更多查看译文
关键词
chexdragon,training,models,healthcare systems,x-ray
AI 理解论文
溯源树
样例
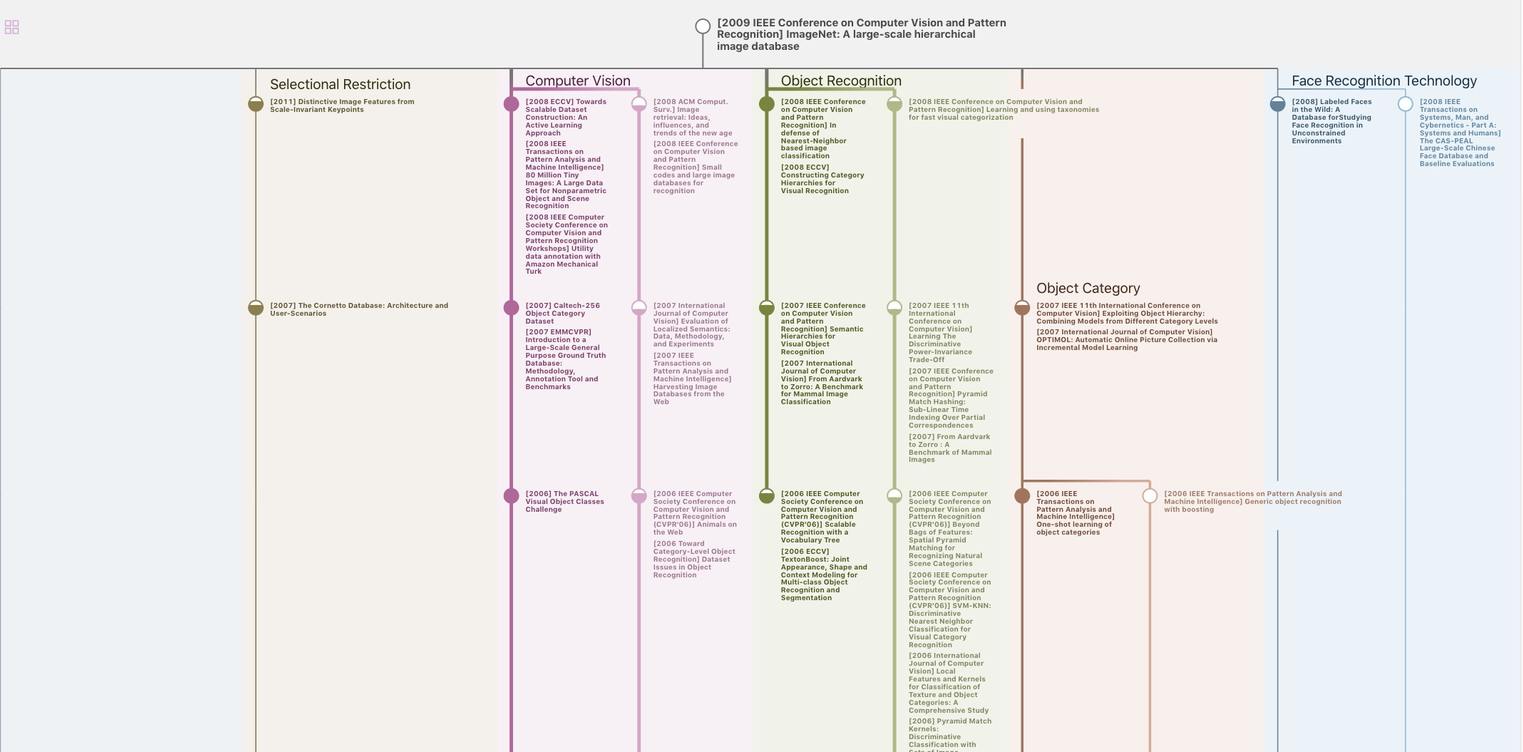
生成溯源树,研究论文发展脉络
Chat Paper
正在生成论文摘要