Vocal Style Factorization for Effective Speaker Recognition in Affective Scenarios
2023 IEEE International Joint Conference on Biometrics (IJCB)(2023)
摘要
The accuracy of automated speaker recognition is negatively impacted by change in emotions in a person's speech. In this paper, we hypothesize that speaker identity is composed of various vocal style factors that may be learned from unlabeled data and re-combined using a neural network architecture to generate holistic speaker identity representations for affective scenarios. In this regard we propose the E-Vector architecture, composed of a 1-D CNN for learning speaker identity features and a vocal style factorization technique for determining vocal styles. Experiments conducted on the MSP-Podcast dataset demonstrate that the proposed architecture improves state-of-the-art speaker recognition accuracy in the affective domain over baseline ECAPA-TDNN speaker recognition models. For instance, the true match rate at a false match rate of 1% improves from 27.6% to 46.2%.
更多查看译文
关键词
Speaker Recognition,Vocal Style,Affective Domain,Personal Emotions,False Matches,Loss Function,Training Data,Recent Literature,Bimodal,Validation Set,Negative Samples,Weight Vector,Trainable Parameters,Sample ID,Pair Of Sets,Matching Score,Audio Data,Pre-trained Weights,Speech Units,Speech Samples,Human Speech,Speech Synthesis,Equal Error Rate,Email Requests,Handcrafted Features,Attention Mechanism,Learning Styles,Area Under Curve
AI 理解论文
溯源树
样例
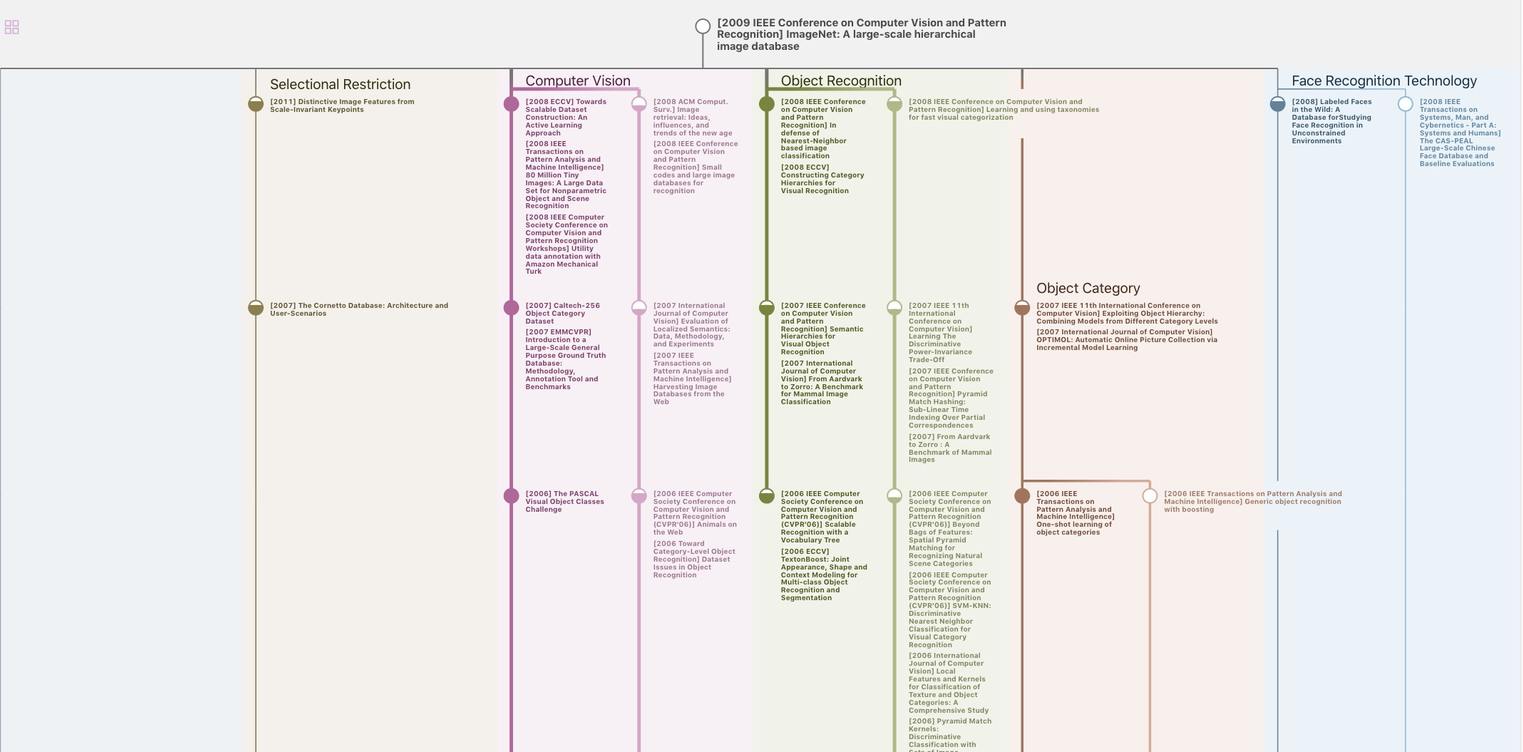
生成溯源树,研究论文发展脉络
Chat Paper
正在生成论文摘要