Robust Kalman Filters Based on the Sub-Gaussian $\alpha$-stable Distribution
arXiv (Cornell University)(2023)
摘要
Motivated by filtering tasks under a linear system with non-Gaussian heavy-tailed noise, various robust Kalman filters (RKFs) based on different heavy-tailed distributions have been proposed. Although the sub-Gaussian $\alpha$-stable (SG$\alpha$S) distribution captures heavy tails well and is applicable in various scenarios, its potential has not yet been explored in RKFs. The main hindrance is that there is no closed-form expression of its mixing density. This paper proposes a novel RKF framework, RKF-SG$\alpha$S, where the signal noise is assumed to be Gaussian and the heavy-tailed measurement noise is modelled by the SG$\alpha$S distribution. The corresponding joint posterior distribution of the state vector and auxiliary random variables is approximated by the Variational Bayesian (VB) approach. Also, four different minimum mean square error (MMSE) estimators of the scale function are presented. The first two methods are based on the Importance Sampling (IS) and Gauss-Laguerre quadrature (GLQ), respectively. In contrast, the last two estimators combine a proposed Gamma series (GS) based method with the IS and GLQ estimators and hence are called GSIS and GSGL. Besides, the RKF-SG$\alpha$S is compared with the state-of-the-art RKFs under three kinds of heavy-tailed measurement noises, and the simulation results demonstrate its estimation accuracy and efficiency.
更多查看译文
关键词
robust kalman filters,sub-gaussian
AI 理解论文
溯源树
样例
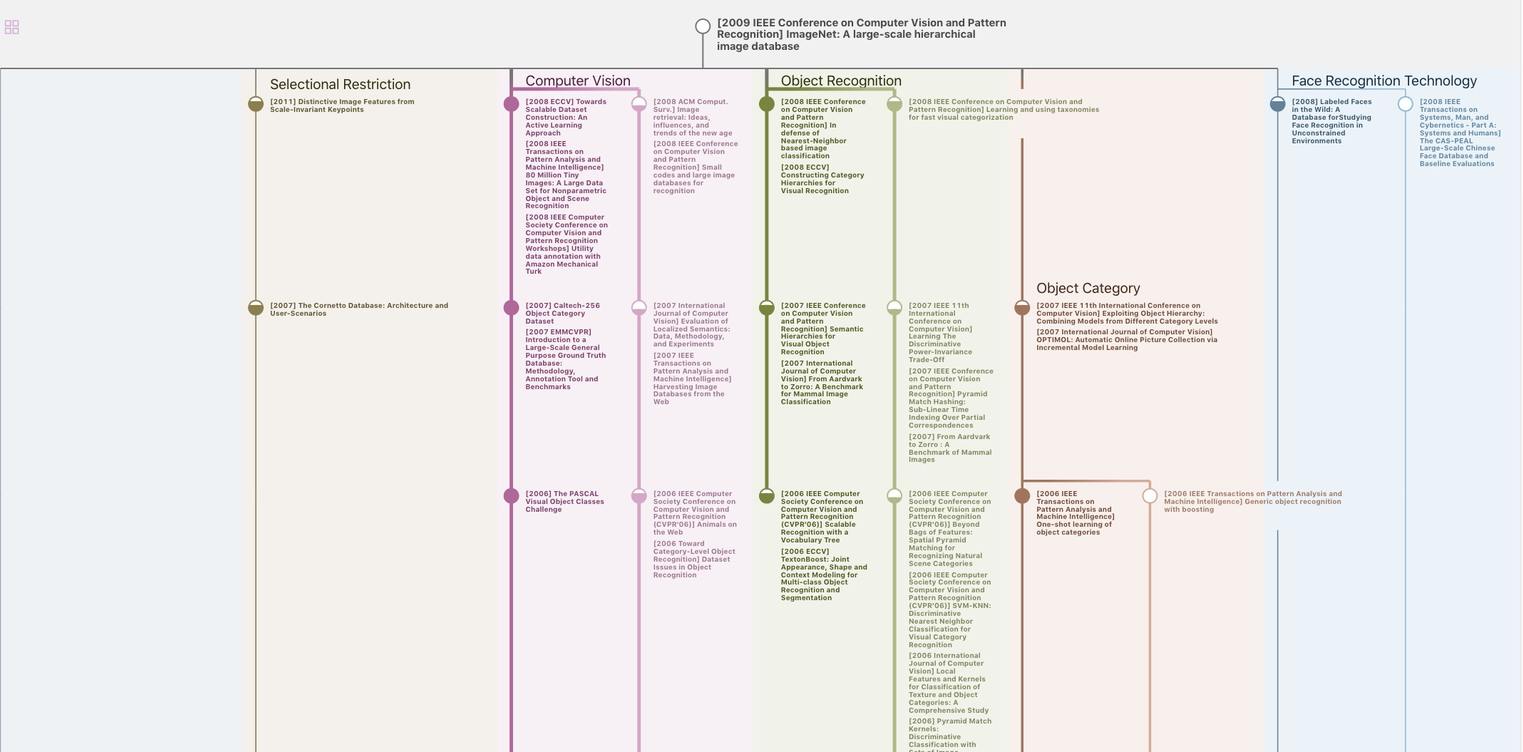
生成溯源树,研究论文发展脉络
Chat Paper
正在生成论文摘要