ZS-GR: zero-shot gesture recognition from RGB-D videos
Multimedia Tools and Applications(2023)
摘要
Gesture Recognition (GR) is a challenging research area in computer vision. To tackle the annotation bottleneck in GR, we formulate the problem of Zero-Shot Gesture Recognition (ZS-GR) and propose a two-stream model from two input modalities: RGB and Depth videos. To benefit from the vision Transformer capabilities, we use two vision Transformer models, for human detection and visual features representation. We configure a transformer encoder-decoder architecture, as a fast and accurate human detection model, to overcome the challenges of the current human detection models. Considering the human keypoints, the detected human body is segmented into nine parts. A spatio-temporal representation from human body is obtained using a vision Transformer and a LSTM network. A semantic space maps the visual features to the lingual embedding of the class labels via a Bidirectional Encoder Representations from Transformers (BERT) model. We evaluated the proposed model on five datasets, Montalbano II, MSR Daily Activity 3D, CAD-60, NTU-60, and isoGD obtaining state-of-the-art results compared to state-of-the-art ZS-GR models as well as the Zero-Shot Action Recognition (ZS-AR).
更多查看译文
关键词
Gesture recognition,Zero-shot learning,Vision transformer,Lingual embedding,BERT,Action recognition
AI 理解论文
溯源树
样例
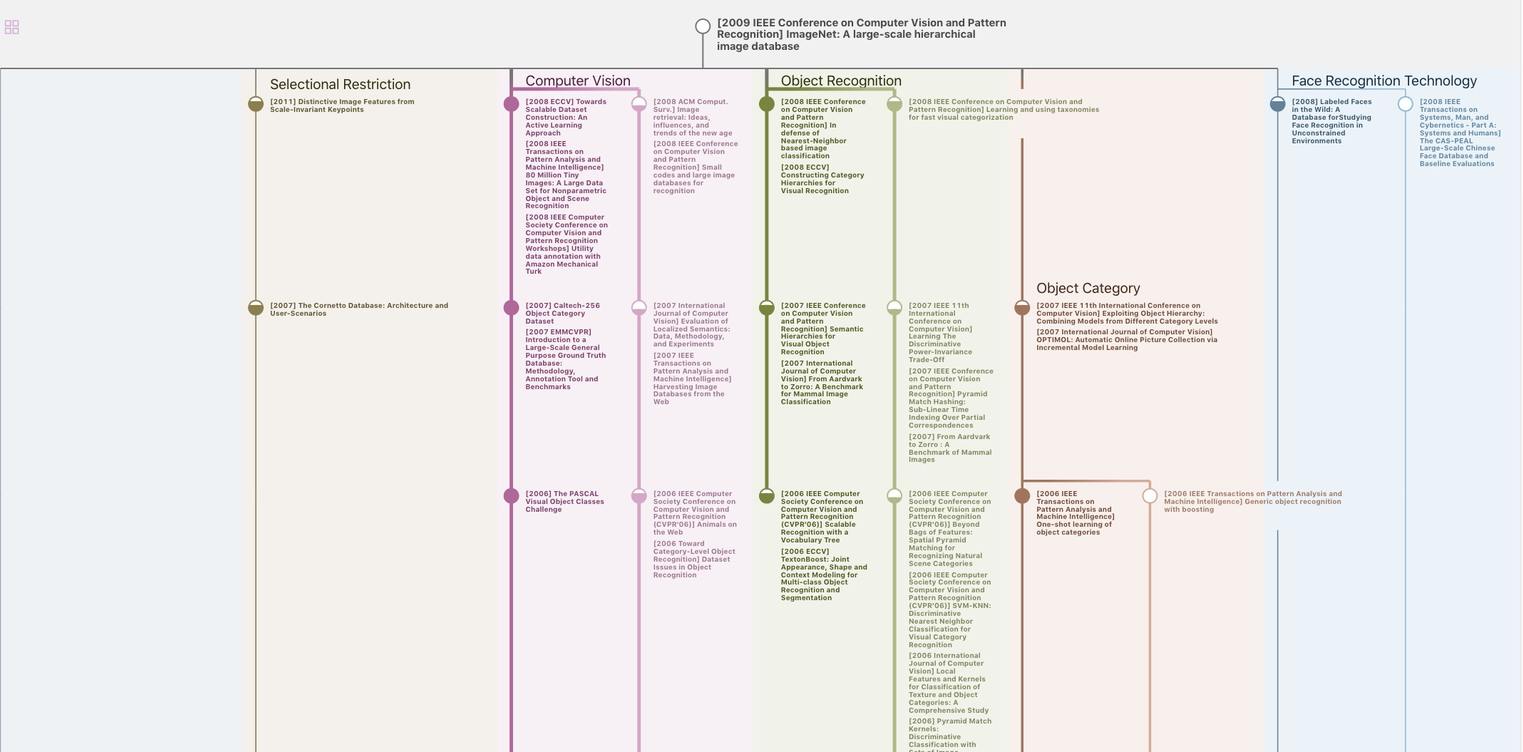
生成溯源树,研究论文发展脉络
Chat Paper
正在生成论文摘要