Causal view mechanism for adversarial domain adaptation
Multimedia Tools and Applications(2023)
摘要
Studies show that the challenge for adversarial domain adaptation is learning domain-invariant representations and alleviating the domain gap. However, the construction of domain-invariant representations suppresses the class-level structure information, and the pursuit of class-level structure information distorts the constructed domain-invariant representations. Till present, it still has difficulty to capture the domain-invariant representations while preserving the class-level structure information in the adversarial training process explicitly. In this paper, we propose a Causal view Mechanism for adversarial Domain Adaptation (CMDA). Firstly, a causal effect model of adversarial DA is proposed and reveals the influence of potential confounders in the adversarial training process. Then, CMDA is proposed to disentangle the domain-specific representations into multiple underlying factors and filter out irrelevant confounding characteristics. Specifically, CMDA capture the desired domain-invariant representations by subtracting the domain-level and class-level confounding characteristics. CMDA not only could preserve the class-level structure information to reduce classification error, but also improve transferability simultaneously. Finally, experiments carried out on Office-31, Office-Home, and VisDA-2017 datasets show that our CMDA method presents strong competition among some recent domain adaptation methods, and the average accuracies achieve 71.3%, 89.3% and 76.1% respectively.
更多查看译文
关键词
Domain adaptation,Transfer learning,Deep learning,Causal inference,Image classification
AI 理解论文
溯源树
样例
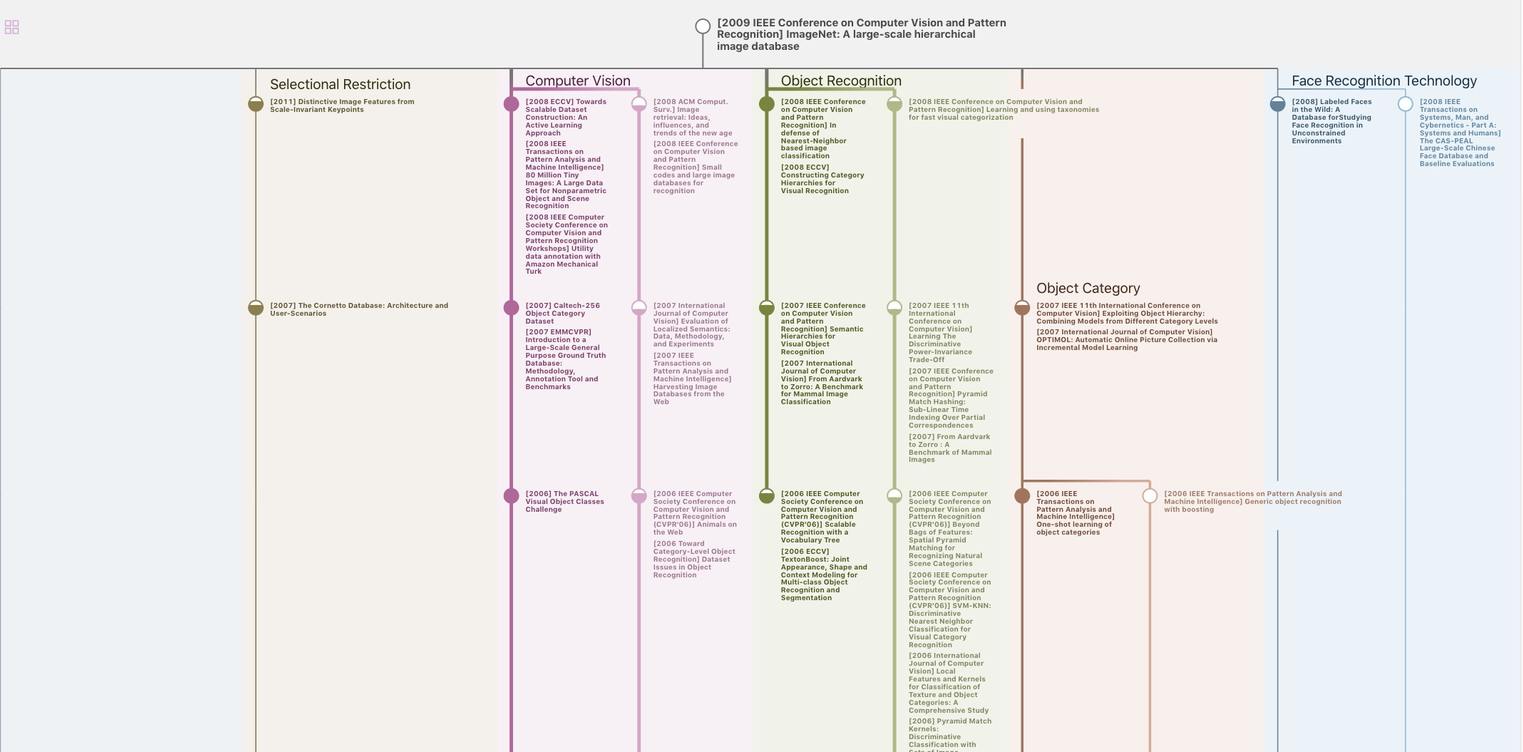
生成溯源树,研究论文发展脉络
Chat Paper
正在生成论文摘要