Rethinking k-means from manifold learning perspective
CoRR(2023)
摘要
Although numerous clustering algorithms have been developed, many existing methods still leverage k-means technique to detect clusters of data points. However, the performance of k-means heavily depends on the estimation of centers of clusters, which is very difficult to achieve an optimal solution. Another major drawback is that it is sensitive to noise and outlier data. In this paper, from manifold learning perspective, we rethink k-means and present a new clustering algorithm which directly detects clusters of data without mean estimation. Specifically, we construct distance matrix between data points by Butterworth filter such that distance between any two data points in the same clusters equals to a small constant, while increasing the distance between other data pairs from different clusters. To well exploit the complementary information embedded in different views, we leverage the tensor Schatten p-norm regularization on the 3rd-order tensor which consists of indicator matrices of different views. Finally, an efficient alternating algorithm is derived to optimize our model. The constructed sequence was proved to converge to the stationary KKT point. Extensive experimental results indicate the superiority of our proposed method.
更多查看译文
关键词
manifold learning perspective,k-means
AI 理解论文
溯源树
样例
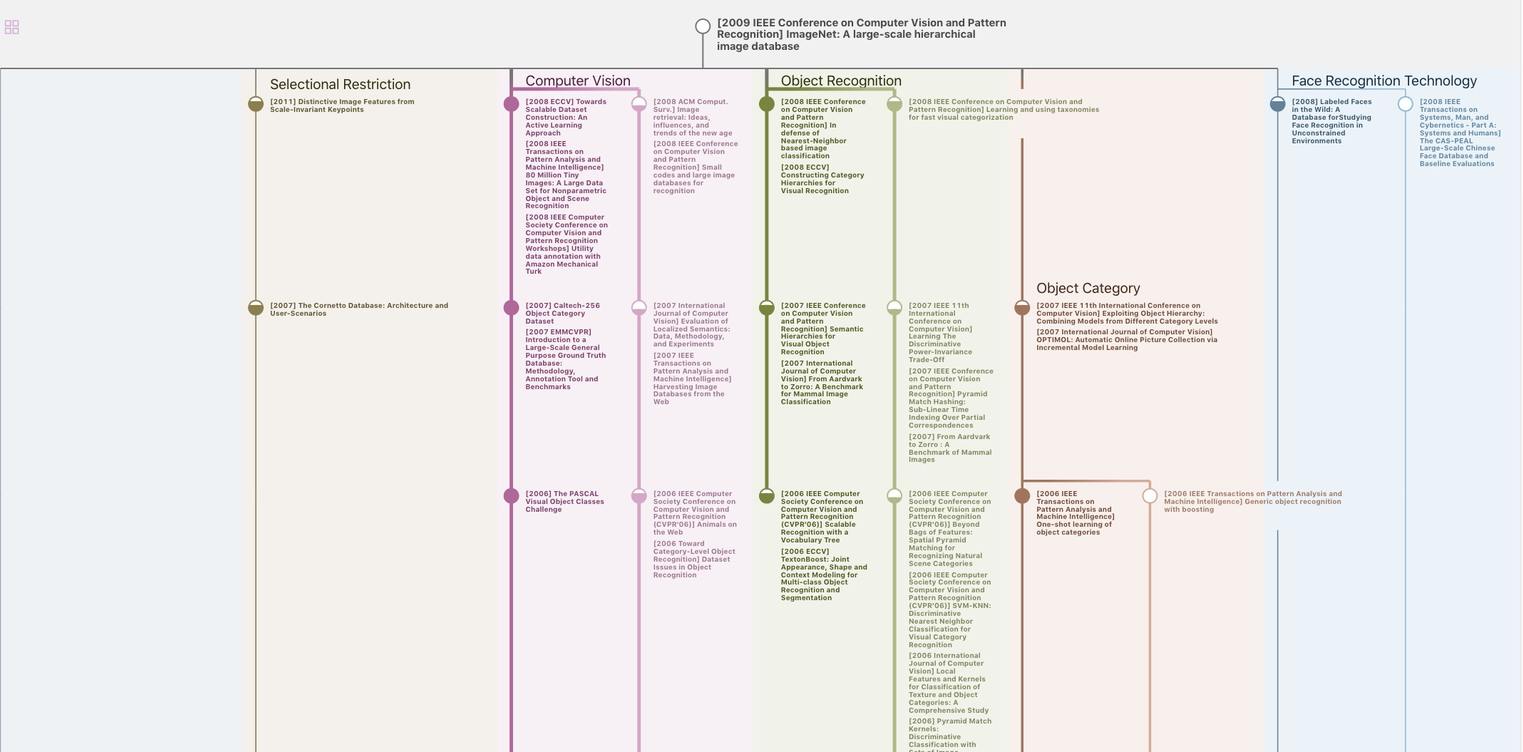
生成溯源树,研究论文发展脉络
Chat Paper
正在生成论文摘要