A Multi-Scale Channel Attention Network for Prostate Segmentation
IEEE Transactions on Circuits and Systems II: Express Briefs(2023)
摘要
Prostate cancer is one of the most common malignant tumors in men. Magnetic resonance imaging (MRI) has evolved to an important tool for the diagnosis of prostate cancer. Targeted biopsy is required for accurate diagnosis. This often requires MRI-ultrasound (MRI-US) fusion, as the biopsy is usually performed using transrectal ultrasound. Accurate prostate segmentation on MRI is essential for MRI-US fusion biopsy. However, the variation in prostate shape, appearance, and size makes the automatic segmentation challenging, given the limit of the annotated data. In this brief, we propose a method using multi-scale and Channel-wise Self-Attention (CSA) to re-calibrate the feature maps from multiple layers. By embedding the multi-scale CSA on the skip-connection in a UNet structure, called as UCAnet, we show the consistent improvement of the prostate segmentation in Dice, IoU and ASSD. For comparison, we also investigate the single-scale CSA in the networks, and incorporate the vision transformer to test if a transformer would boost the performance. Experiments on a public dataset with 204 prostate MRI scans show that UCAnet achieves the best performance and outperforms the state-of-the-art methods for prostate segmentation such as ENet, UNet, USE-Net and TransUNet.
更多查看译文
关键词
Magnetic resonance imaging,Transformers,Image segmentation,Biomedical imaging,Shape,Glands,Feature extraction,Prostate segmentation,self-attention,vision transformer,CNN,channel-wise,multi-scale
AI 理解论文
溯源树
样例
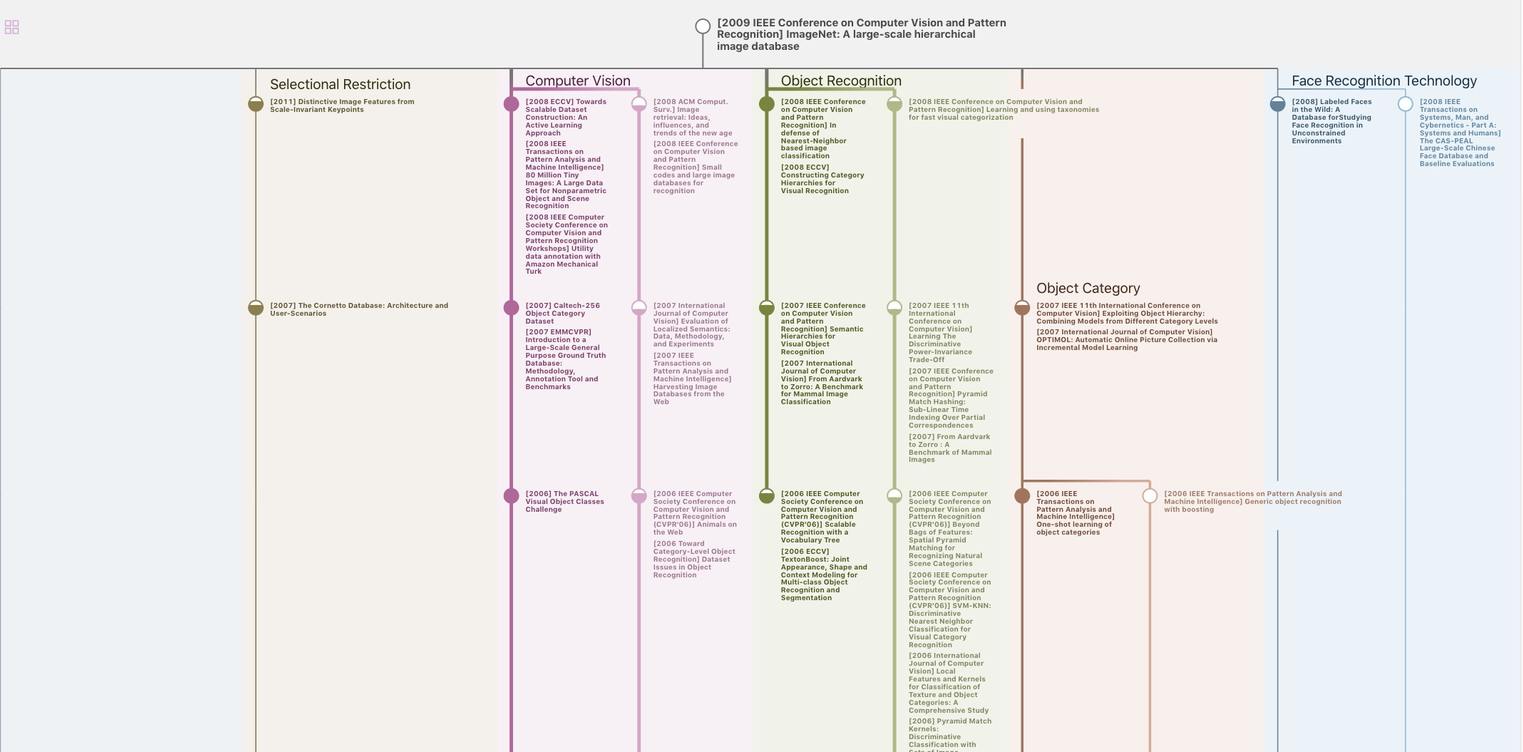
生成溯源树,研究论文发展脉络
Chat Paper
正在生成论文摘要