BDD-Net+: A Building Damage Detection Framework Based on Modified Coat-Net
IEEE Journal of Selected Topics in Applied Earth Observations and Remote Sensing(2023)
摘要
The accurate and fast assessment of damaged buildings following a disaster is critical for planning rescue and reconstruction efforts. The damage assessment by the traditional methods is time-consuming and with limited performance. In this article, we propose an end-to-end deep-learning network named building damage detection network-plus (BDD-Net+). The BDD-Net+ is based on a combination of convolution layers and transformer blocks. The proposed framework takes the advantage of the multiscale residual convolution blocks and self-attention layers. The proposed framework consists of four main steps: data preparation, model training, damage map generation and evaluation, and the use of an explainable artificial intelligence (XAI) framework for understanding and interpretation of the operation model. The experimental results include two representative real-world benchmark datasets (i.e., the Haiti earthquake and the Bata explosion). The obtained results illustrate that BDD-Net+ achieves excellent efficacy in comparison with other state-of-the-art methods. Furthermore, the visualization of the results by XAI shows that BDD-Net+ provides more interpretable and explainable results for damage detection than the other studied methods.
更多查看译文
关键词
building damage detection framework,bdd-net,coat-net
AI 理解论文
溯源树
样例
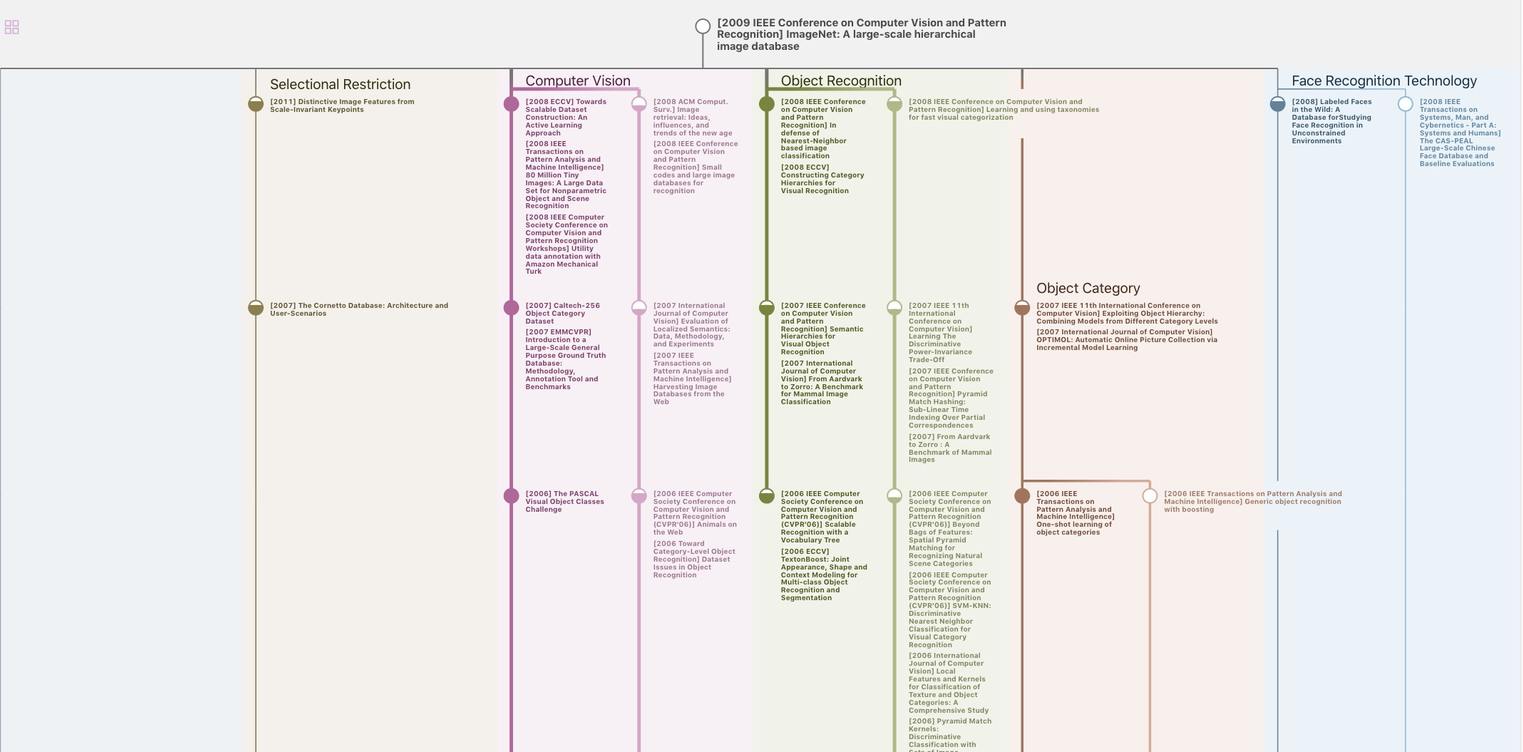
生成溯源树,研究论文发展脉络
Chat Paper
正在生成论文摘要