A Dam Deformation Residual Correction Method for High Arch Dams Using Phase Space Reconstruction and an Optimized Long Short-Term Memory Network
Mathematics(2023)
摘要
Dam safety is an important basic part of national water network security. Building a dam deformation prediction model based on monitoring data is crucial to ensure dam safety. However, traditional statistical regression methods have shortcomings, such as a weak nonlinear fitting ability when constructing dam deformation monitoring and prediction models. The residual part of the statistical regression results usually contains parts that cannot be effectively explained by the linear regression method, that is usually highly variable and noisy. In this study, the phase space reconstruction method is used to smooth the residual term of the statistical regression model to eliminate noise interference. On this basis, an improved long short-term memory (LSTM) neural network is used to learn the nonlinearity contained in the residual term of the linear regression. Considering the impact of parameter selection on model performance, the gray wolf optimization (GWO) algorithm is used to determine the optimal parameters of the model for better performance. A high arch dam is used as a case study, with multiple measuring points used as research objects. The experimental results show that the phase space reconstruction can effectively smooth the high-frequency components in the residual term and remove noise interference. In addition, the GWO algorithm can effectively determine the hyperparameters of the LSTM network, thereby constructing a residual prediction model with high prediction accuracy. The combination of statistical models and deep learning prediction methods can effectively improve the model prediction performance while preserving the model interpretability and transparency.
更多查看译文
关键词
dam safety monitoring,residual correction,prediction model,deep learning
AI 理解论文
溯源树
样例
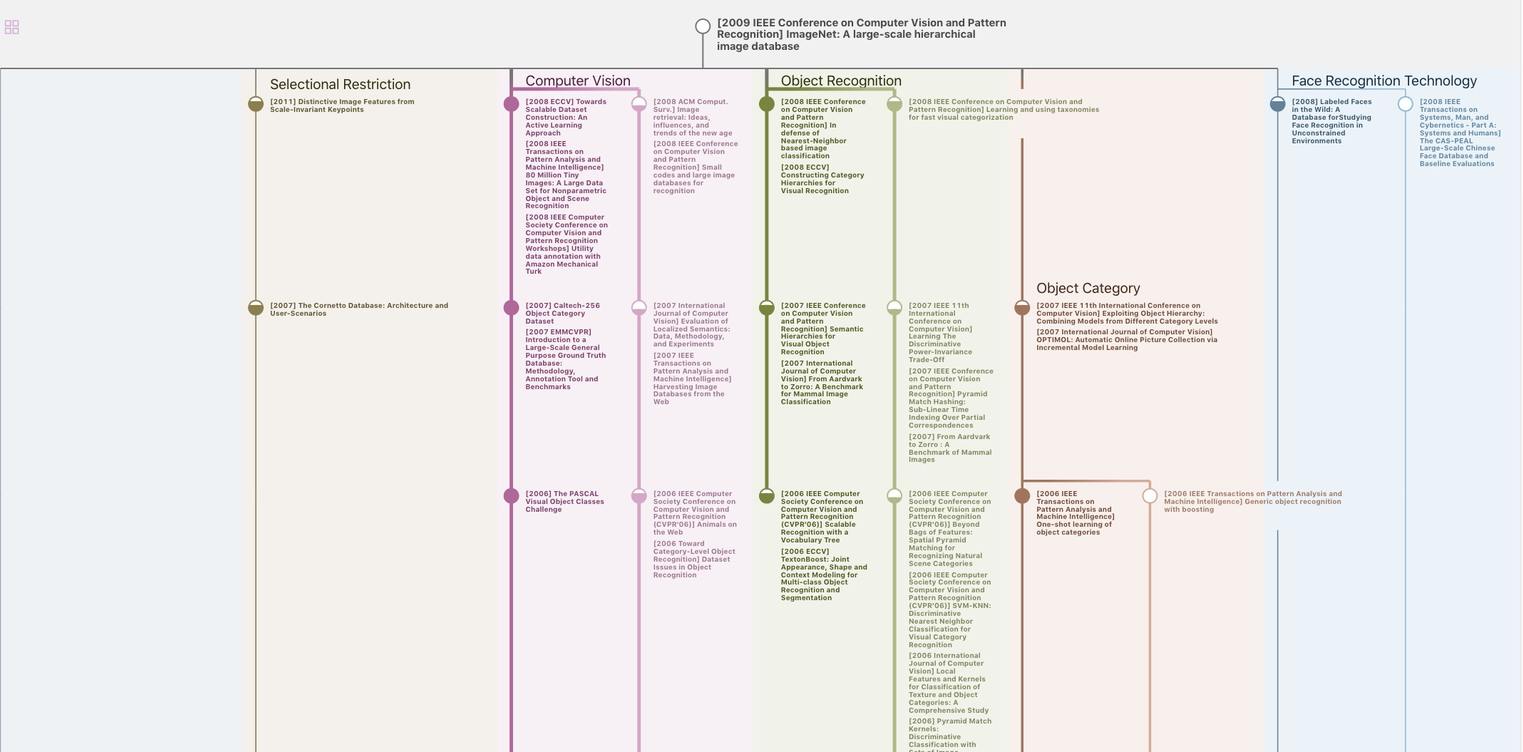
生成溯源树,研究论文发展脉络
Chat Paper
正在生成论文摘要