Implicitly normalized forecaster with clipping for linear and non-linear heavy-tailed multi-armed bandits
Computational Management Science(2024)
摘要
The Implicitly Normalized Forecaster (INF) algorithm is considered to be an optimal solution for adversarial multi-armed bandit (MAB) problems. However, most of the existing complexity results for INF rely on restrictive assumptions, such as bounded rewards. Recently, a related algorithm was proposed that works for both adversarial and stochastic heavy-tailed MAB settings. However, this algorithm fails to fully exploit the available data. In this paper, we propose a new version of INF called the Implicitly Normalized Forecaster with clipping (INF-clip) for MAB problems with heavy-tailed reward distributions. We establish convergence results under mild assumptions on the rewards distribution and demonstrate that INF-clip is optimal for linear heavy-tailed stochastic MAB problems and works well for non-linear ones. Furthermore, we show that INF-clip outperforms the best-of-both-worlds algorithm in cases where it is difficult to distinguish between different arms.
更多查看译文
关键词
Multi-armed bandits,Clipping,Online mirror descent
AI 理解论文
溯源树
样例
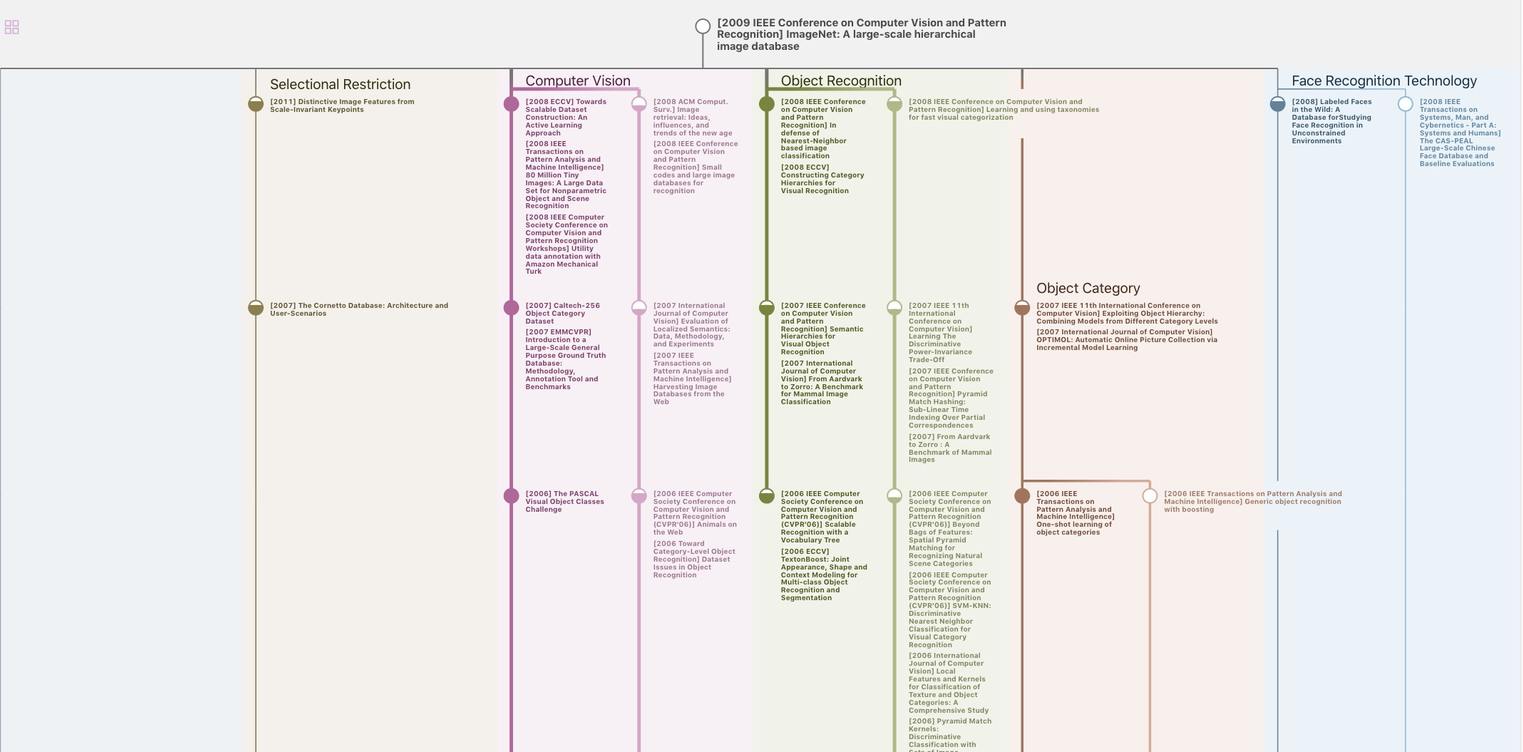
生成溯源树,研究论文发展脉络
Chat Paper
正在生成论文摘要