Modeling and predictive control of nonlinear processes using transfer learning method
AICHE JOURNAL(2023)
摘要
This work develops a transfer learning (TL) framework for modeling and predictive control of nonlinear systems using recurrent neural networks (RNNs) with the knowledge obtained in modeling one process transferred to another. Specifically, transfer learning uses a pretrained model developed based on a source domain as the starting point, and adapts the model to a target process with similar configurations. The generalization error for TL-based RNN (TL-RNN) is first derived to demonstrate the generalization capability on the target process. The theoretical error bound that depends on model capacity and the discrepancy between source and target domains is then utilized to guide the development of pretrained models for improved model transferability. Subsequently, the TL-RNN model is utilized as the prediction model in model predictive controller (MPC) for the target process. Finally, the simulation study of chemical reactors via Aspen Plus Dynamics is used to demonstrate the benefits of transfer learning.
更多查看译文
关键词
transfer learning method,predictive control,nonlinear processes
AI 理解论文
溯源树
样例
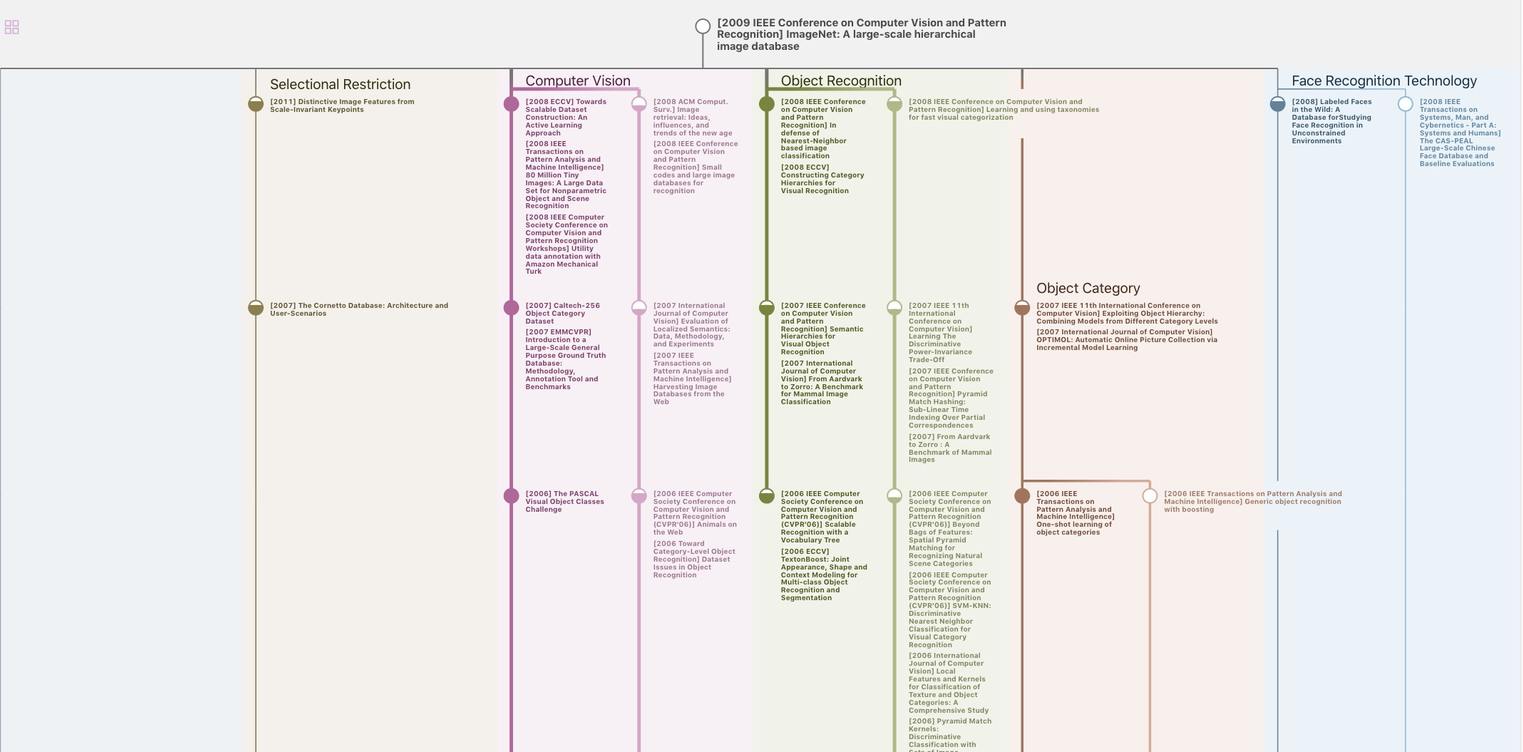
生成溯源树,研究论文发展脉络
Chat Paper
正在生成论文摘要