Model Selection for Time Series Forecasting An Empirical Analysis of Multiple Estimators
NEURAL PROCESSING LETTERS(2023)
摘要
Evaluating predictive models is a crucial task in predictive analytics. This process is especially challenging with time series data because observations are not independent. Several studies have analyzed how different performance estimation methods compare with each other for approximating the true loss incurred by a given forecasting model. However, these studies do not address how the estimators behave for model selection: the ability to select the best solution among a set of alternatives. This paper addresses this issue. The goal of this work is to compare a set of estimation methods for model selection in time series forecasting tasks. This objective is split into two main questions: (i) analyze how often a given estimation method selects the best possible model; and (ii) analyze what is the performance loss when the best model is not selected. Experiments were carried out using a case study that contains 3111 time series. The accuracy of the estimators for selecting the best solution is low, despite being significantly better than random selection. Moreover, the overall forecasting performance loss associated with the model selection process ranges from 0.28 to 0.58%. Yet, no considerable differences between different approaches were found. Besides, the sample size of the time series is an important factor in the relative performance of the estimators.
更多查看译文
关键词
Model selection, Performance estimation, Cross-validation, Time series, Forecasting
AI 理解论文
溯源树
样例
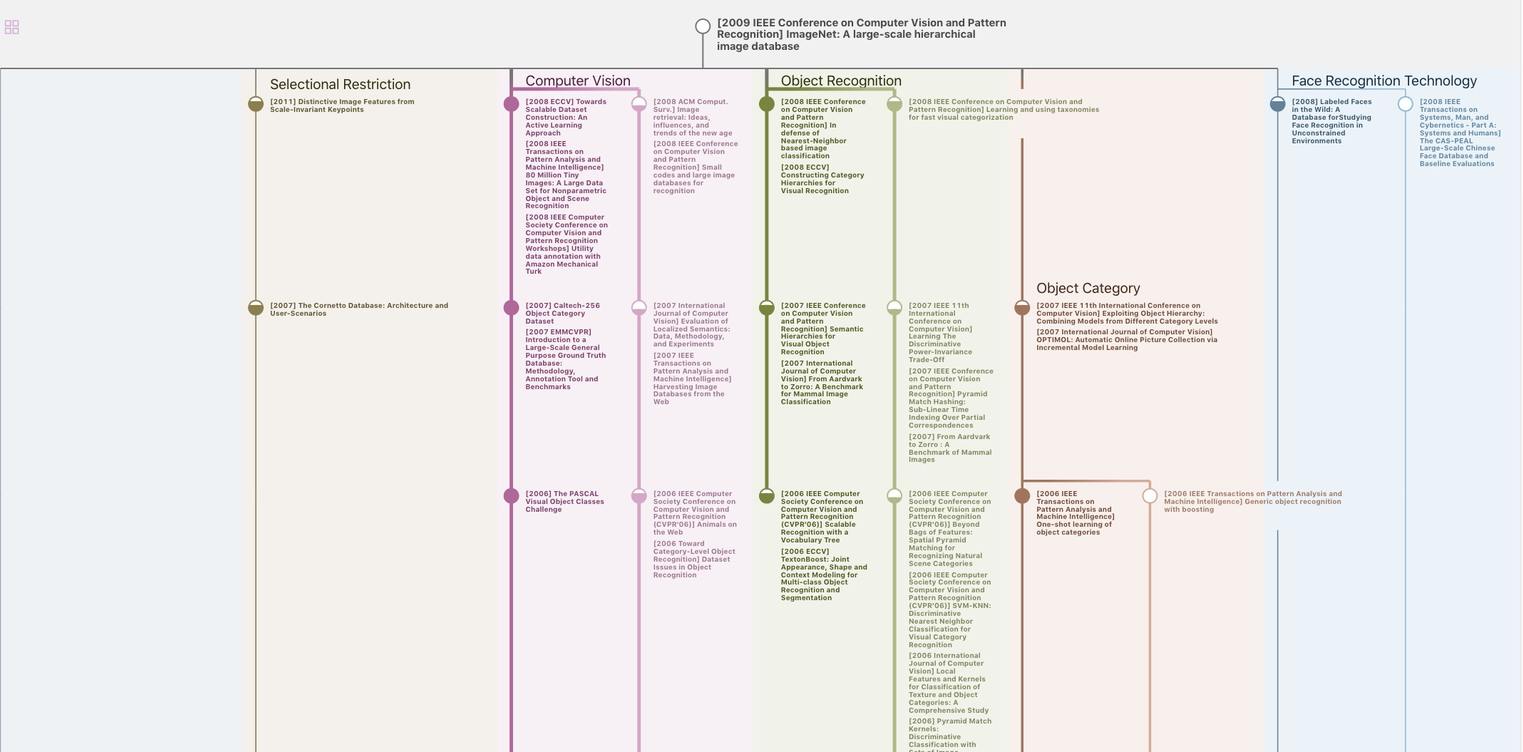
生成溯源树,研究论文发展脉络
Chat Paper
正在生成论文摘要