Occupancy inference using infrastructure elements in indoor environment: a multi-sensor data fusion
CCF TRANSACTIONS ON PERVASIVE COMPUTING AND INTERACTION(2023)
摘要
Gathering occupancy information in indoor environments has gained extensive research interest due to its potential use in energy savings, user comfort and degree of automation. Occupancy information has become a decisive element in many automation systems, such as smart homes and offices, where energy demand is directly linked to user occupancy. However, there is a trade-off between energy consumption and user comfort, thus requiring fine-grained and reliable occupancy information. In this context, this paper presents a multi-modal occupancy inference system to infer the occupancy status of a large Lab or an office setting. Many past works have used different sensors to infer the occupancy of a room. However, we believe that this work is the first of its kind that uses non-intrusive infrastructure-based sensing modalities, such as WiFi and Bluetooth, and fuses them using Dempster–Shafer evidence theory to obtain accurate occupancy information. The system is tested in a graduate student Lab, and the experimental results show that the proposed method provides substantially high accuracy (87.69%) as compared to any single sensing modality. We also establish through empirical studies that the proposed system gives optimal results when the mass assignments are calculated over three to six days on our datasets.
更多查看译文
关键词
indoor environment,infrastructure elements,fusion,multi-sensor
AI 理解论文
溯源树
样例
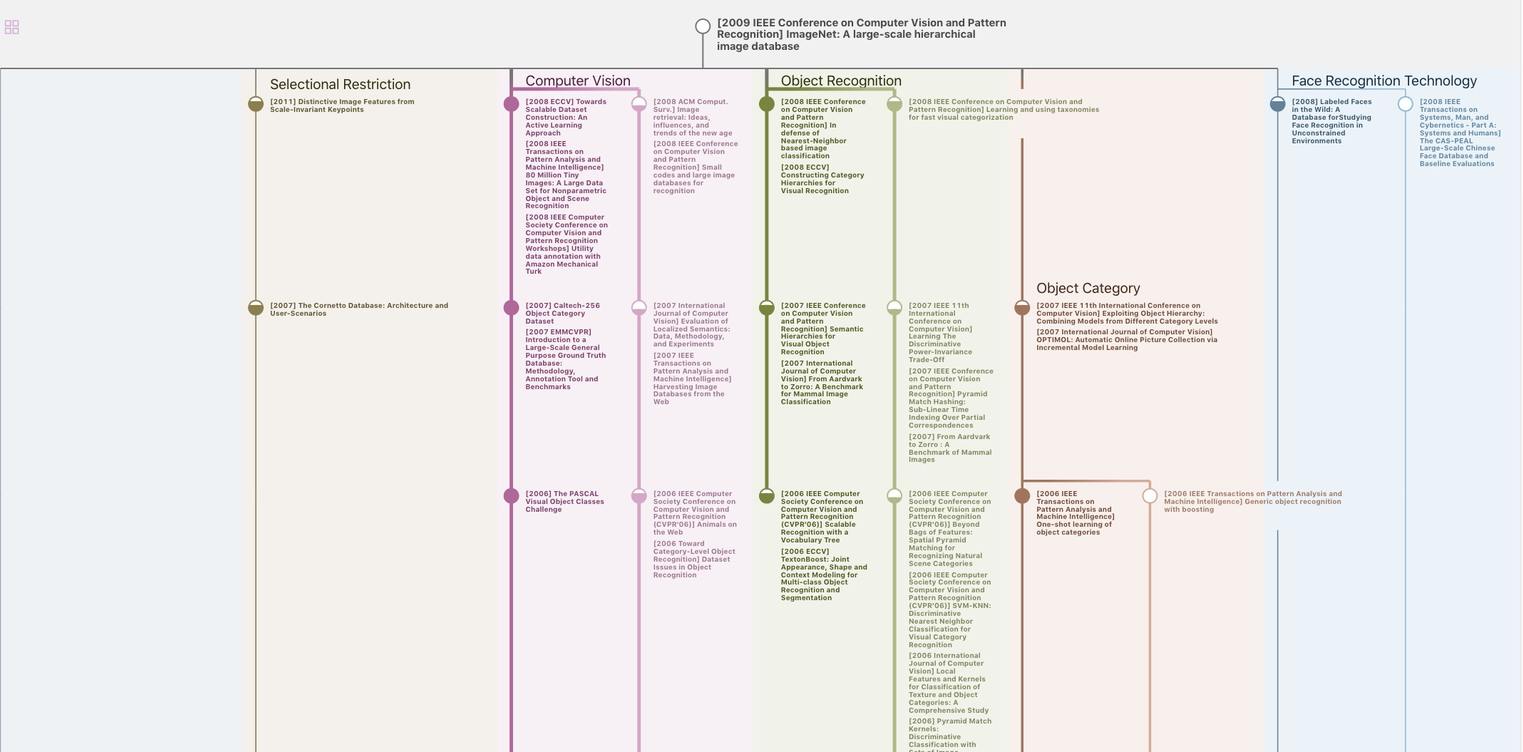
生成溯源树,研究论文发展脉络
Chat Paper
正在生成论文摘要