Multimodal convolutional neural networks for predicting evolution of gyrokinetic simulations
CONTRIBUTIONS TO PLASMA PHYSICS(2023)
摘要
Gyrokinetic simulations are required for the quantitative calculation of fluxes due to turbulence, which dominates over other transport mechanisms in tokamaks. However, nonlinear gyrokinetic simulations are computationally expensive. A multimodal convolutional neural network model that reads images and values generated by nonlinear gyrokinetic simulations and predicts electrostatic turbulent heat fluxes was developed to support efficient runs. The model was extended to account for squared electrostatic potential fluctuations, which are proportional to the fluxes in the quasilinear model, as well as images containing fluctuating electron and ion distribution functions and fluctuating electrostatic potentials in wavenumber space. This multimodal model can predict the time and electron and ion turbulent heat fluxes corresponding to the input data. The model trained on the Cyclone base case data successfully predicted times and fluxes not only for its test data, but also for the completely different and unknown JT-60U case, with high accuracy. The predictive performance of the model depended on the similarity of the linear stability of the case used to train the model to the case being predicted.
更多查看译文
关键词
gyrokinetic simulations,multimodal convolutional neural networks,convolutional neural networks,neural networks
AI 理解论文
溯源树
样例
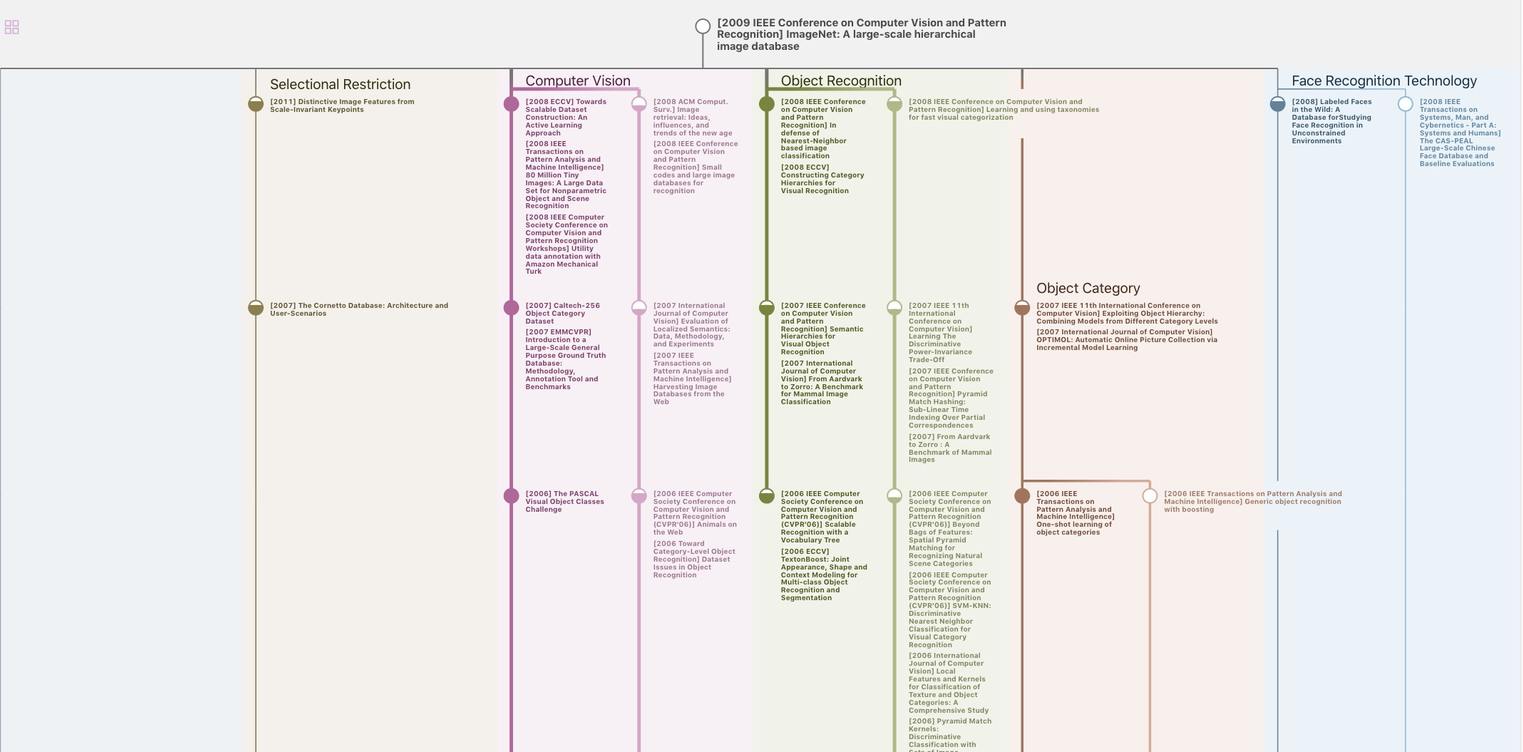
生成溯源树,研究论文发展脉络
Chat Paper
正在生成论文摘要