New encoder–decoder convolutional LSTM neural network architectures for next-day global ionosphere maps forecast
GPS SOLUTIONS(2023)
摘要
Global navigation satellite system (GNSS) signals are significantly affected by the ionosphere. An efficient way to assess the ionospheric effects on GNSS signals is by retrieving the vertical total electron content (VTEC). Here, we propose convolutional recurrent neural network architectures to forecast VTEC based on global ionosphere maps (GIMs) of the days before the prediction period. We proposed modifications to the encoder–decoder convolutional long short-term memory (ED-ConvLSTM) architecture by innovatively using GIMs from several days and exploring the previous day’s GIM. Three new architectures were tested: The first one uses a residual connection to force the network to learn the difference between the previous and the next-day GIM; the second one uses the memory from the encoder to improve the transformation from the previous to the next-day GIM; and a third one uses 3 × 3 kernels on the encoder and 1 × 1 kernels on the decoder. Two experiments were performed using the international GNSS service (IGS) final GIM product from the years 2014–2015 (high solar activity) and 2019–2020 (low solar activity). The proposed architectures obtained better results than the original version of ED-ConvLSTM and other baseline models. We evaluated the influence of the number of GIMs (from one to four days) in the next-day GIM predictions. The results suggest that providing more than one day of GIM to the proposed networks can lead to better prediction metrics.
更多查看译文
关键词
Deep learning,Total Electron Content,Ionosphere,Forecast,ConvLSTM,Residual neural networks
AI 理解论文
溯源树
样例
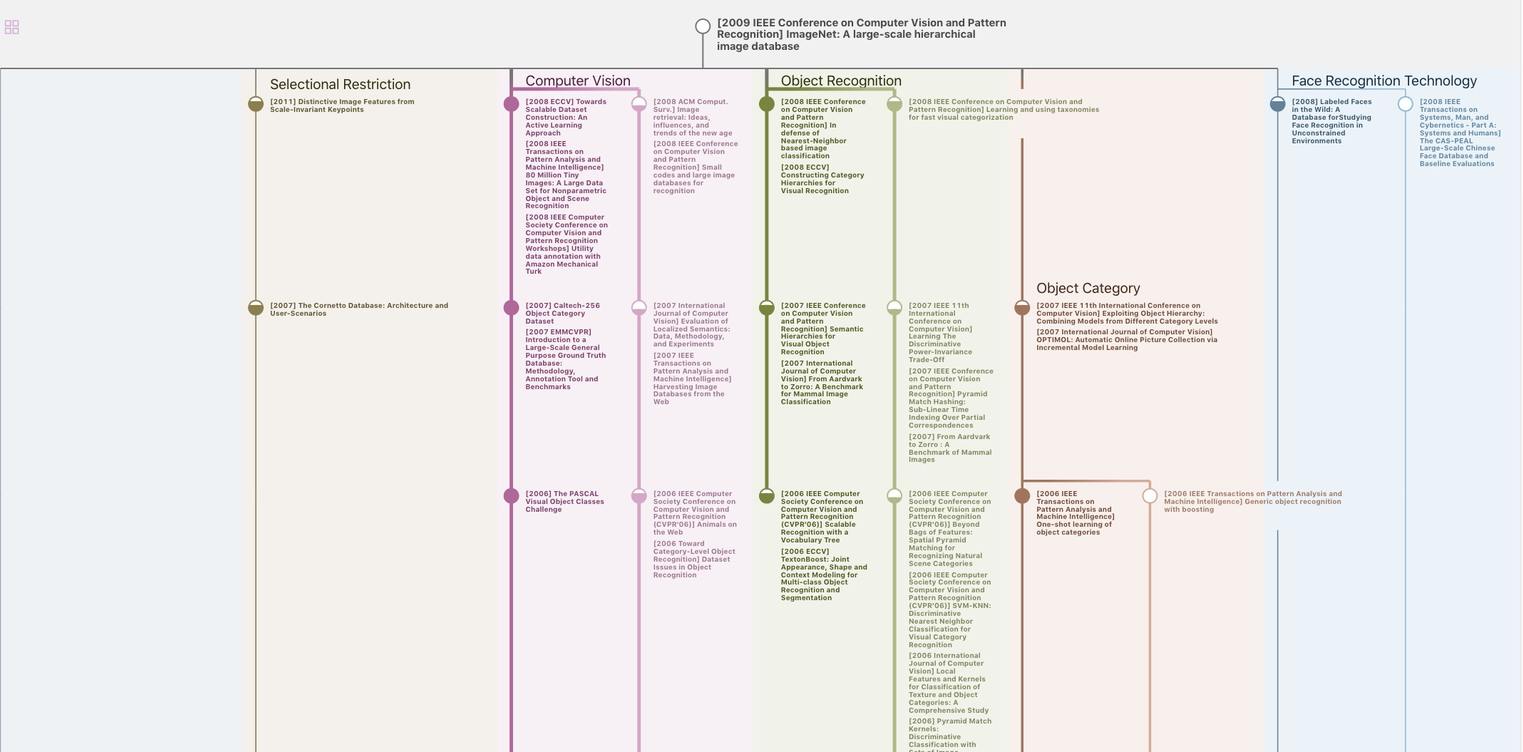
生成溯源树,研究论文发展脉络
Chat Paper
正在生成论文摘要