Deep Learning-Based Radiomics for Prognostic Stratification of Low-Grade Gliomas Using a Multiple-Gene Signature
APPLIED SCIENCES-BASEL(2023)
摘要
Low-grade gliomas are a heterogeneous group of infiltrative neoplasms. Radiomics allows the characterization of phenotypes with high-throughput extraction of quantitative imaging features from radiologic images. Deep learning models, such as convolutional neural networks (CNNs), offer well-performing models and a simplified pipeline by automatic feature learning. In our study, MRI data were retrospectively obtained from The Cancer Imaging Archive (TCIA), which contains MR images for a subset of the LGG patients in The Cancer Genome Atlas (TCGA). Corresponding molecular genetics and clinical information were obtained from TCGA. Three genes included in the genetic signatures were WEE1, CRTAC1, and SEMA4G. A CNN-based deep learning model was used to classify patients into low and high-risk groups, with the median gene signature risk score as the cut-off value. The data were randomly split into training and test sets, with 61 patients in the training set and 20 in the test set. In the test set, models using T1 and T2 weighted images had an area under the receiver operating characteristic curve of 73% and 79%, respectively. In conclusion, we developed a CNN-based model to predict non-invasively the risk stratification provided by the prognostic gene signature in LGGs. Numerous previously discovered gene signatures and novel genetic identifiers that will be developed in the future may be utilized with this method.
更多查看译文
关键词
deep learning, radiomics, neural networks, gliomas, LGG, gene signatures
AI 理解论文
溯源树
样例
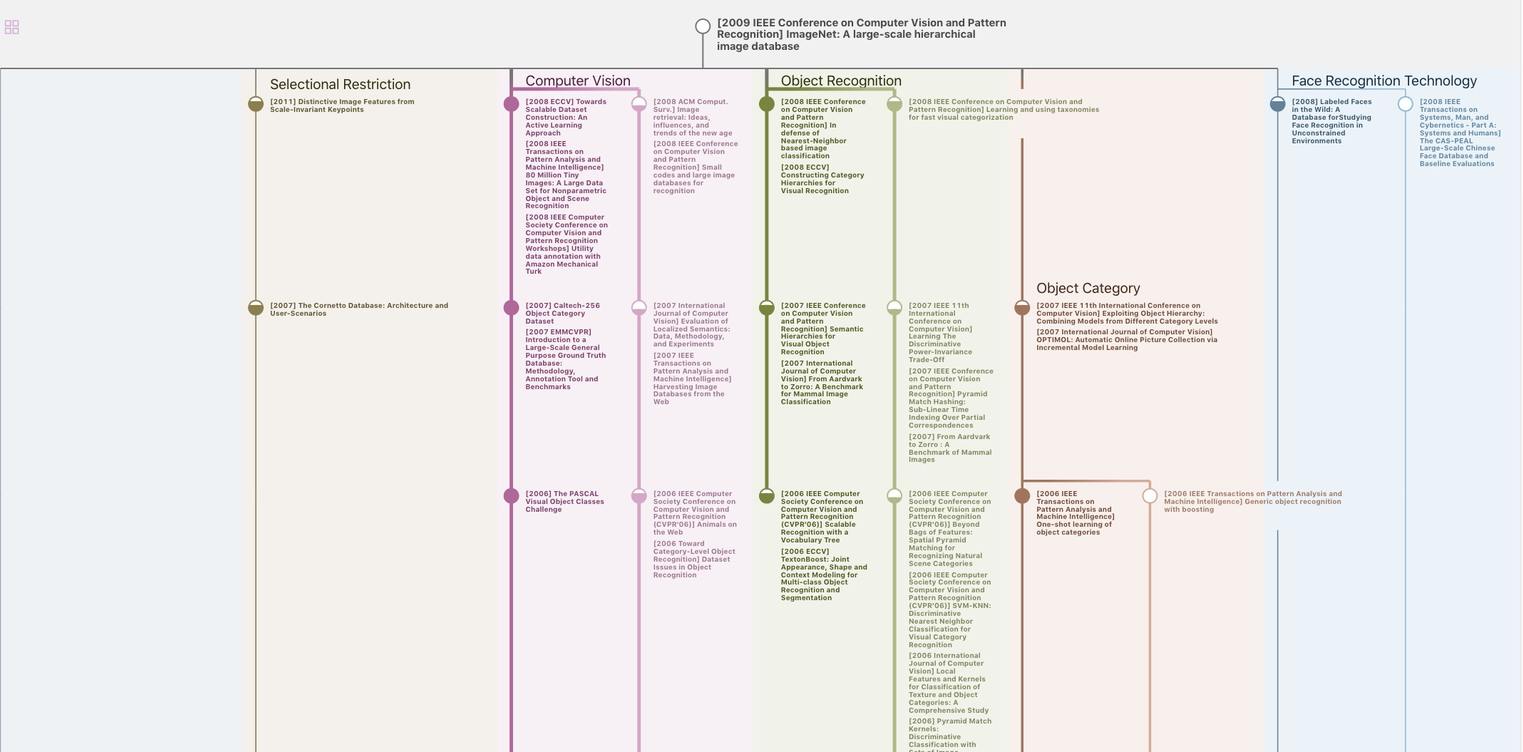
生成溯源树,研究论文发展脉络
Chat Paper
正在生成论文摘要