Spatiotemporal self-supervised representation learning from multi-lead ECG signals
BIOMEDICAL SIGNAL PROCESSING AND CONTROL(2023)
摘要
Automatic analysis of electrocardiogram(ECG) signals is one of the applications in the medical domain where deep learning methods demonstrate impressive performance. However, label scarcity remains a critical obstacle limiting the development and clinical application of deep learning methods. Self-supervised pretraining exhibits its potential to alleviate this issue. Motivated by the idea that multi-lead ECG signals gathered simultane-ously represent similar underlying cardiac status, we propose ECG-MAE, a novel generative self-supervised pretraining approach. Specifically, an efficient encoder is designed to learn spatiotemporal representations by reconstructing 12-lead ECG signals that have been randomly masked in both time and lead dimensions. Experiments on a multi-label classification problem reveal that our pretrained model obtains a macro-averaged AUC of 0.9474, exceeding prior studies. We observe enhanced downstream performance and label efficiency compared with purely supervised models, allowing us to train more powerful models with the same amount of data and diagnose rare diseases beyond the reach of conventional methods.
更多查看译文
关键词
signals,learning,self-supervised,multi-lead
AI 理解论文
溯源树
样例
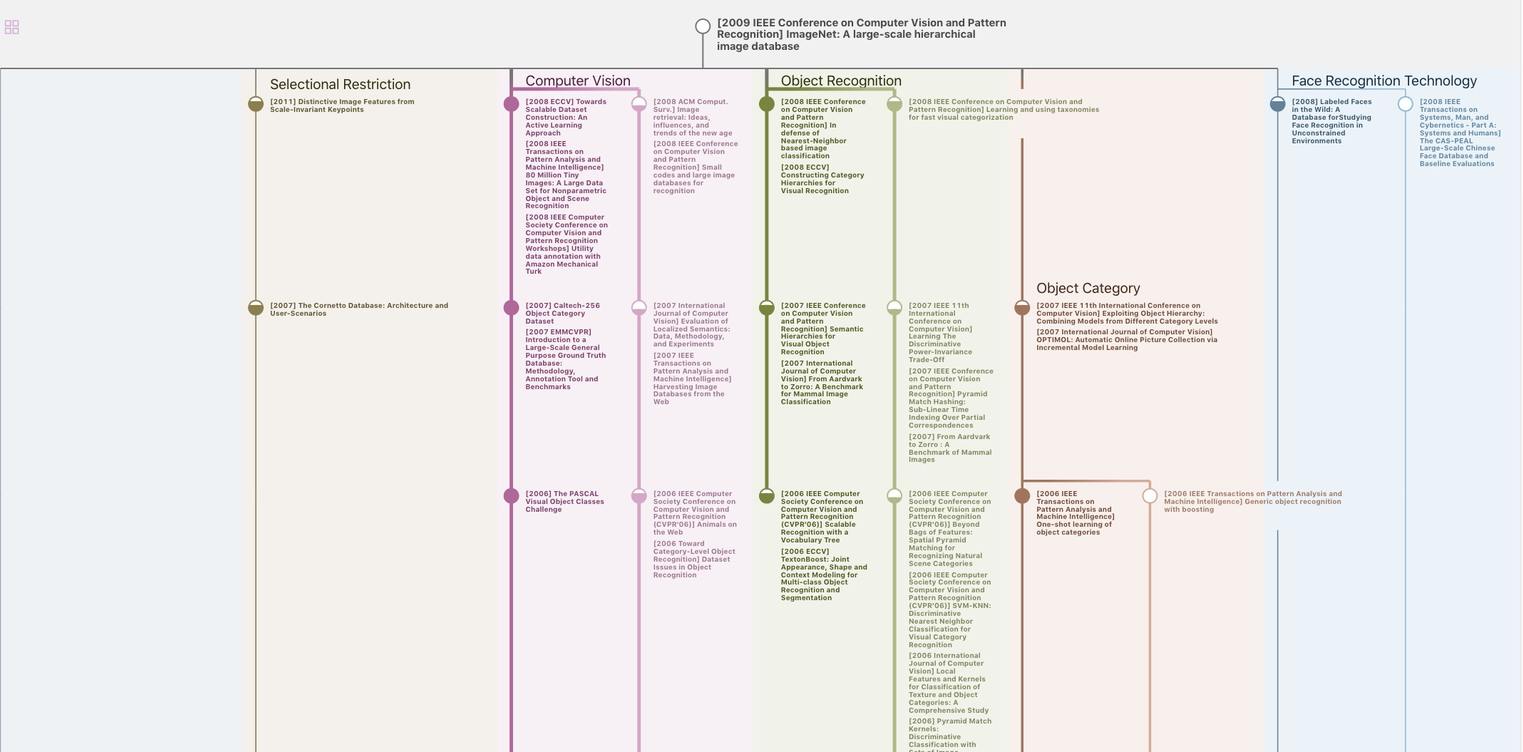
生成溯源树,研究论文发展脉络
Chat Paper
正在生成论文摘要