A comprehensive data-driven assessment scheme for power battery of large-scale electric vehicles in cloud platform
JOURNAL OF ENERGY STORAGE(2023)
摘要
In cloud platform with power battery data from large-scale electric vehicles (EVs), cloud battery management system needs to achieve various monitoring tasks, including safety, degradation, and variation analysis. The unstable data quality and deployment conditions limit the full usage of existing methods, such as model-based estimation method and machine learning algorithm. This paper presents a comprehensive data-driven assessment scheme, named as ComDAS, to improve the multi-tasks realization for cloud platform. The proposed ComDAS can be fast deployed for lithium-ion batteries of EVs, and integrates three mainly concerned aspects: capacity estimation, anomaly detection, and variation evaluation. Neural network is applied for calibration of capacity estimation to achieve higher accuracy. A risk scoring method is proposed to achieve long-scale statistics filtering in time dimension, and weeks-early risk pre-warning for anomaly detection. Considering weak labels or even no labels of cloud EV data, correlation analysis among capacity, anomaly, and variation are also calculated to conduct cross validations and reveal the coupling evolution mechanism among the three aspects. Based on the designed monitoring process for large-scale EVs, ComDAS has been deployed to a realistic cloud big-data platform in Sichuan, China. The working ComDAS can hold the capacity error within 4.5 %, obtain the risk prewarning more than one week earlier, and maintain the variation score of all EVs into 0-100 for further detection. Hence, it proves that ComDAS can maintain satisfying real-time performance for both large-scale EV statistics and specific detection of risky EV.
更多查看译文
关键词
Lithium -ion battery, Anomaly detection, Degradation analysis, Cell -to -cell variation, Data -driven algorithm, Cloud data
AI 理解论文
溯源树
样例
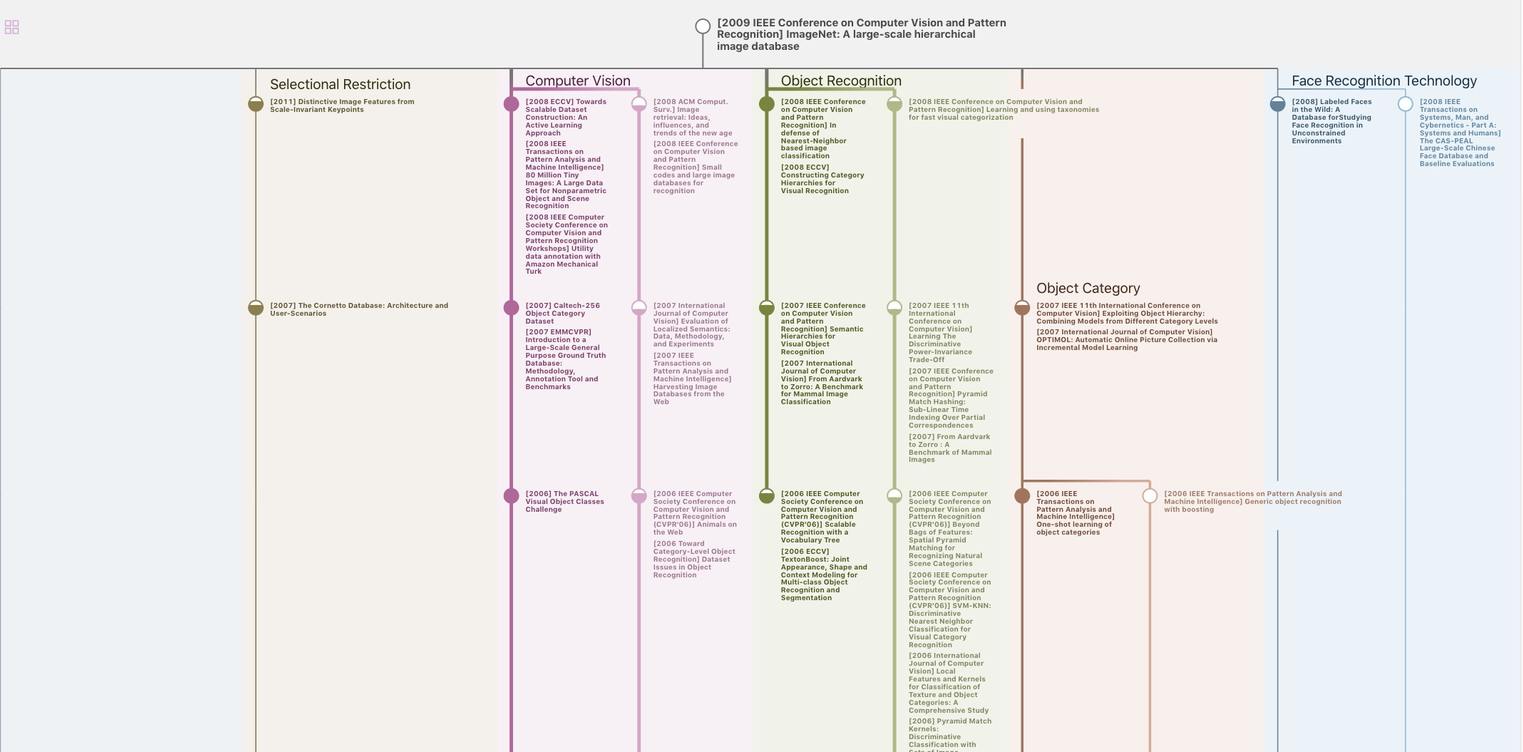
生成溯源树,研究论文发展脉络
Chat Paper
正在生成论文摘要